Explainable Machine Learning in Deployment
FAT* '20: PROCEEDINGS OF THE 2020 CONFERENCE ON FAIRNESS, ACCOUNTABILITY, AND TRANSPARENCY(2019)
摘要
Explainable machine learning seeks to provide various stakeholders with insights into model behavior via feature importance scores, counterfactual explanations, and influential samples, among other techniques. Recent advances in this line of work, however, have gone without surveys of how organizations are using these techniques in practice. This study explores how organizations view and use explainability for stakeholder consumption. We find that the majority of deployments are not for end users affected by the model but for machine learning engineers, who use explainability to debug the model itself. There is a gap between explainability in practice and the goal of public transparency, since explanations primarily serve internal stakeholders rather than external ones. Our study synthesizes the limitations with current explainability techniques that hamper their use for end users. To facilitate end user interaction, we develop a framework for establishing clear goals for explainability, including a focus on normative desiderata.
更多查看译文
关键词
machine learning, explainability, transparency, deployed systems, qualitative study
AI 理解论文
溯源树
样例
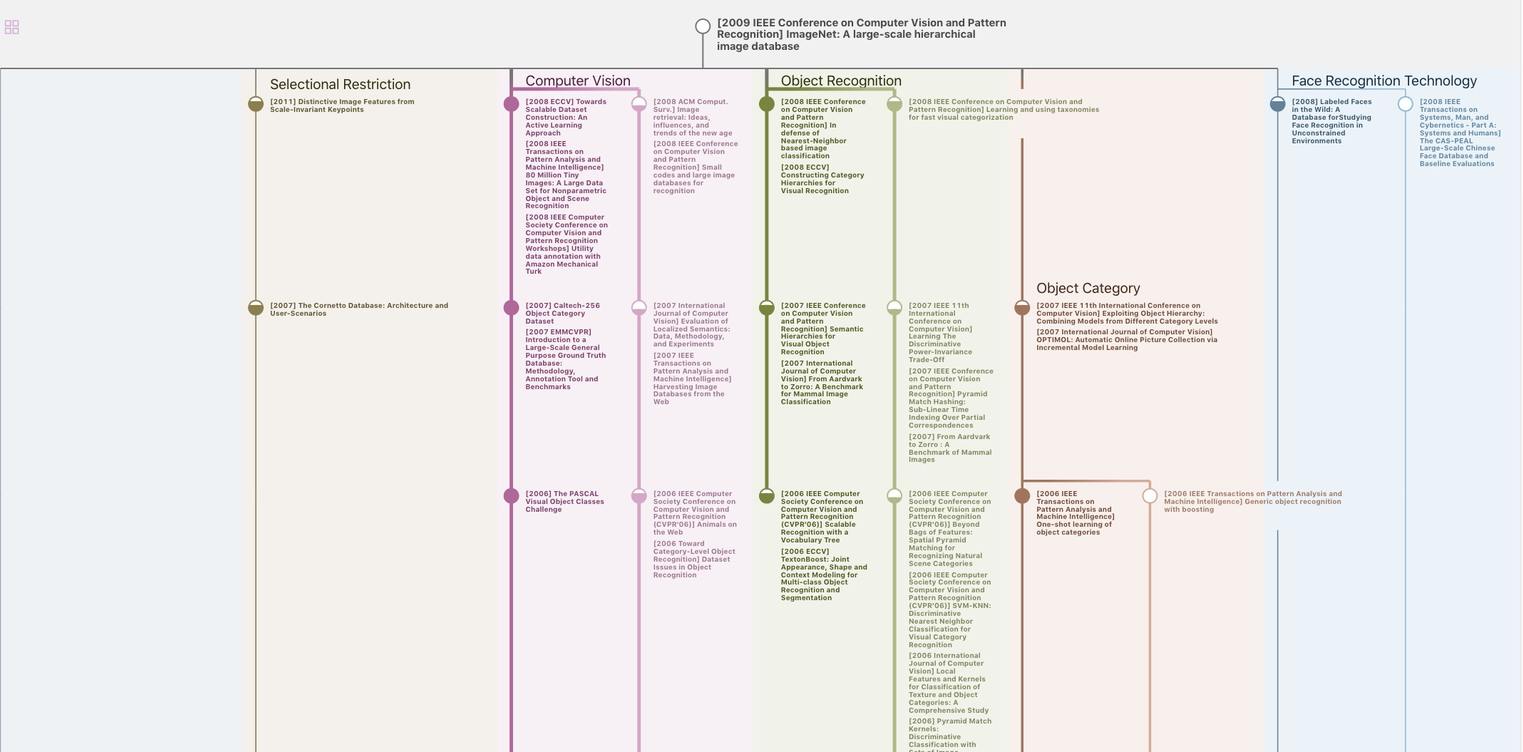
生成溯源树,研究论文发展脉络
Chat Paper
正在生成论文摘要