Coarse2Fine: a Two-Stage Training Method for Fine-Grained Visual Classification
Machine Vision and Applications(2021)
关键词
Fine-grained visual classification,Weakly supervised object localization,Visual attention networks
AI 理解论文
溯源树
样例
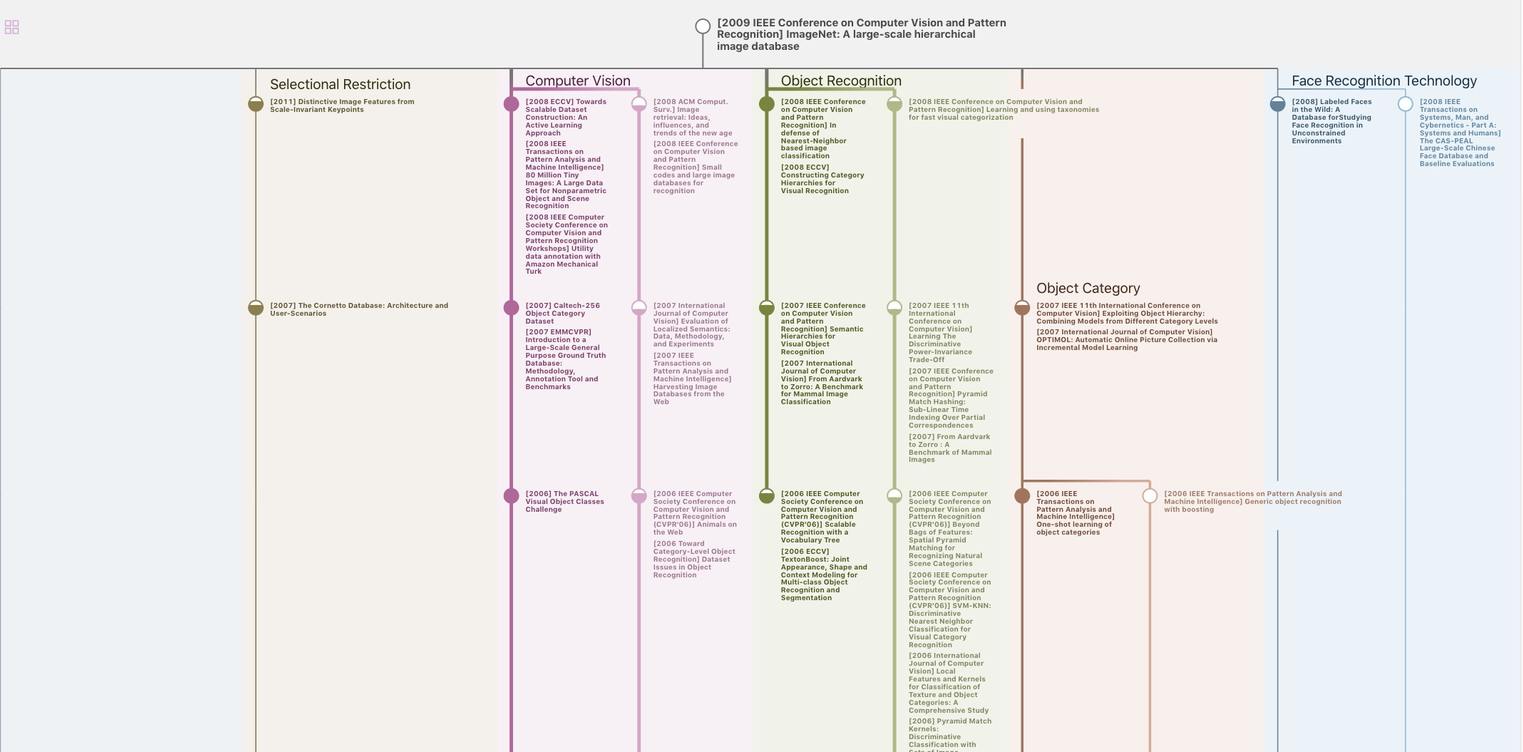
生成溯源树,研究论文发展脉络
Chat Paper
正在生成论文摘要