Causal Heterogeneity Discovery by Bottom-Up Pattern Search for Personalised Decision Making
APPLIED INTELLIGENCE(2023)
关键词
Personalised decision making,Treatment effect heterogeneity,Treatment effect pattern,Conditional average treatment effect
AI 理解论文
溯源树
样例
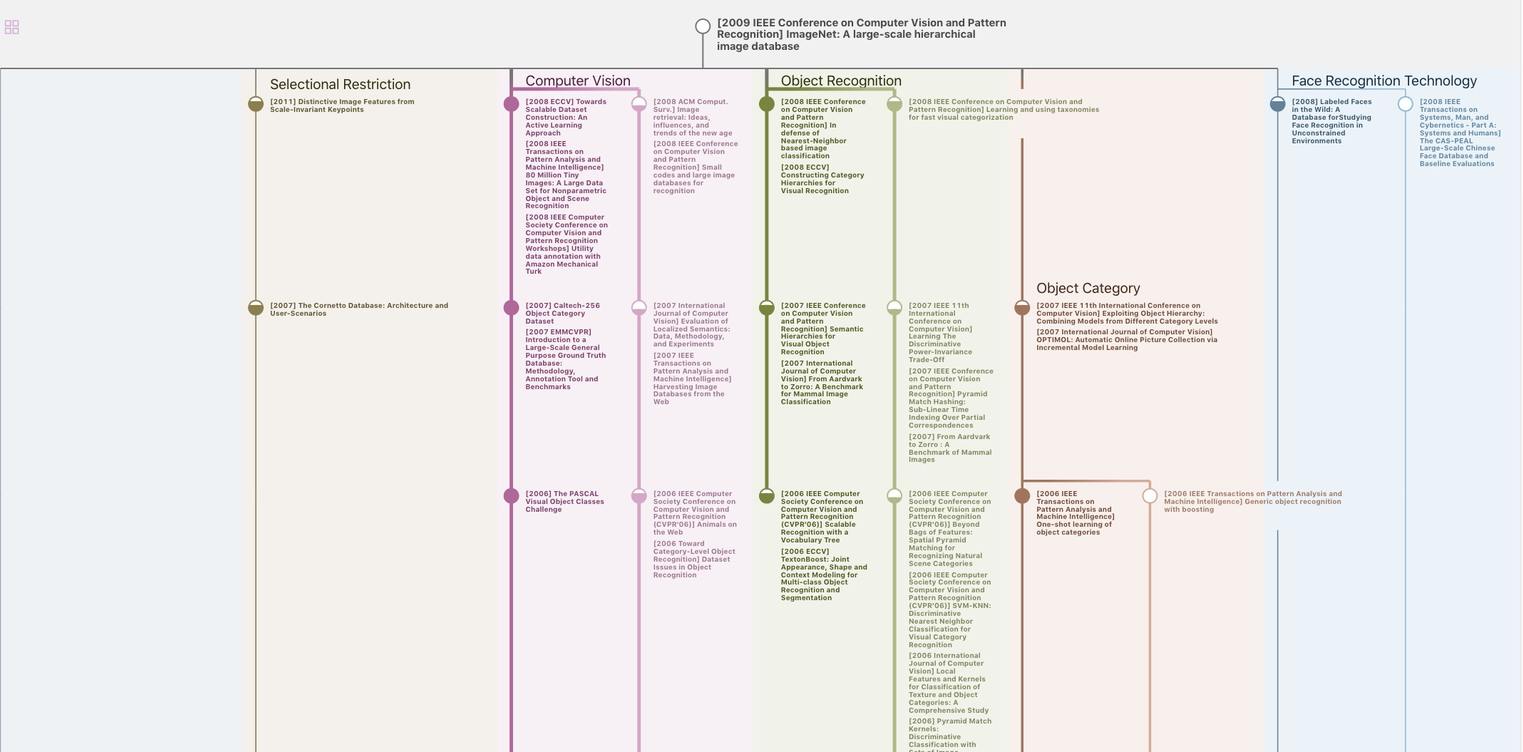
生成溯源树,研究论文发展脉络
Chat Paper
正在生成论文摘要