An Improved Deep Convolutional Neural Network with Multi-Scale Information for Bearing Fault Diagnosis
Neurocomputing(2019)
关键词
Deep learning,Convolutional neural network,Multi-scale cascade,Rolling bearing,Fault diagnosis
AI 理解论文
溯源树
样例
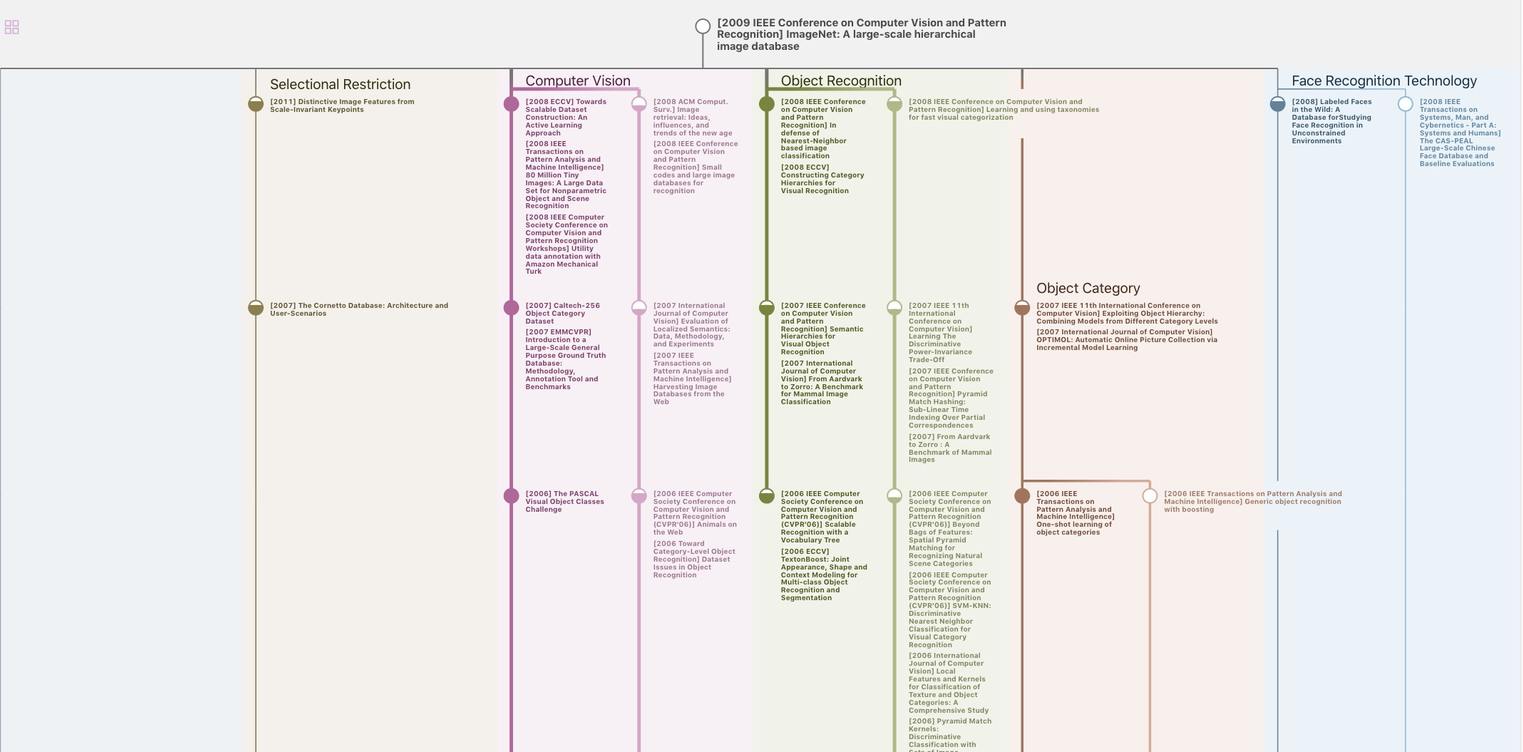
生成溯源树,研究论文发展脉络
Chat Paper
正在生成论文摘要