Robust and Unsupervised KPI Anomaly Detection Based on Conditional Variational Autoencoder
2018 IEEE 37th International Performance Computing and Communications Conference (IPCCC)(2018)
关键词
dropout layer,anomaly detection best F1-score,conditional variational autoencoder,CPU usages,network throughput,page views,online users,trigger timely troubleshooting,detection approaches,traditional statistical approaches,supervised ensemble approaches,time information related anomalies,robust detection algorithm,unsupervised anomaly detection algorithm,key performance indicator,undisrupted Web-based services,unsupervised KPI anomaly detection,unsupervised approach Donut
AI 理解论文
溯源树
样例
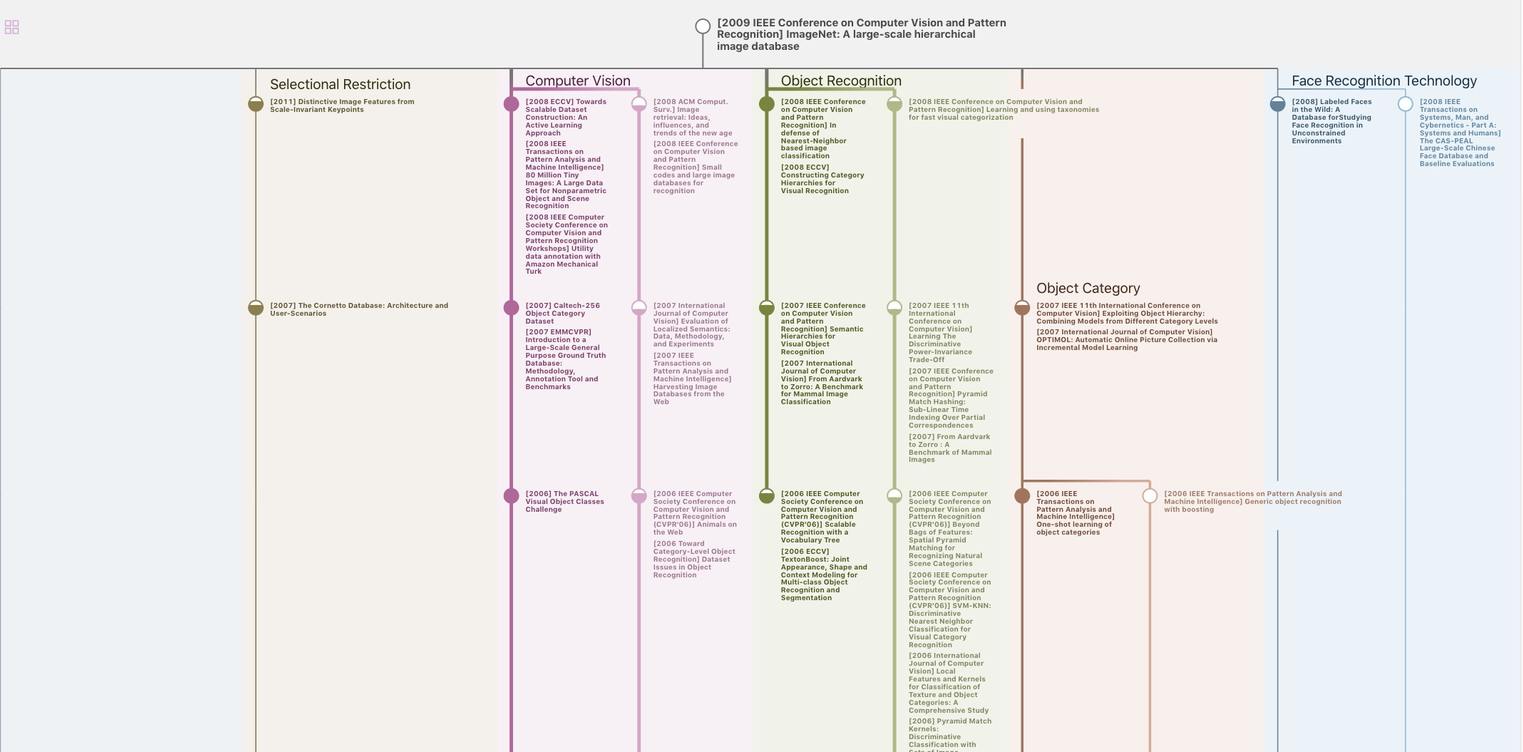
生成溯源树,研究论文发展脉络
Chat Paper
正在生成论文摘要