Semantically-enhanced Kernel Canonical Correlation Analysis: a Multi-Label Cross-Modal Retrieval
Multimedia Tools and Applications(2018)
关键词
Cross-modal retrieval,Kernel CCA,Multi-label information,Concept correlations
AI 理解论文
溯源树
样例
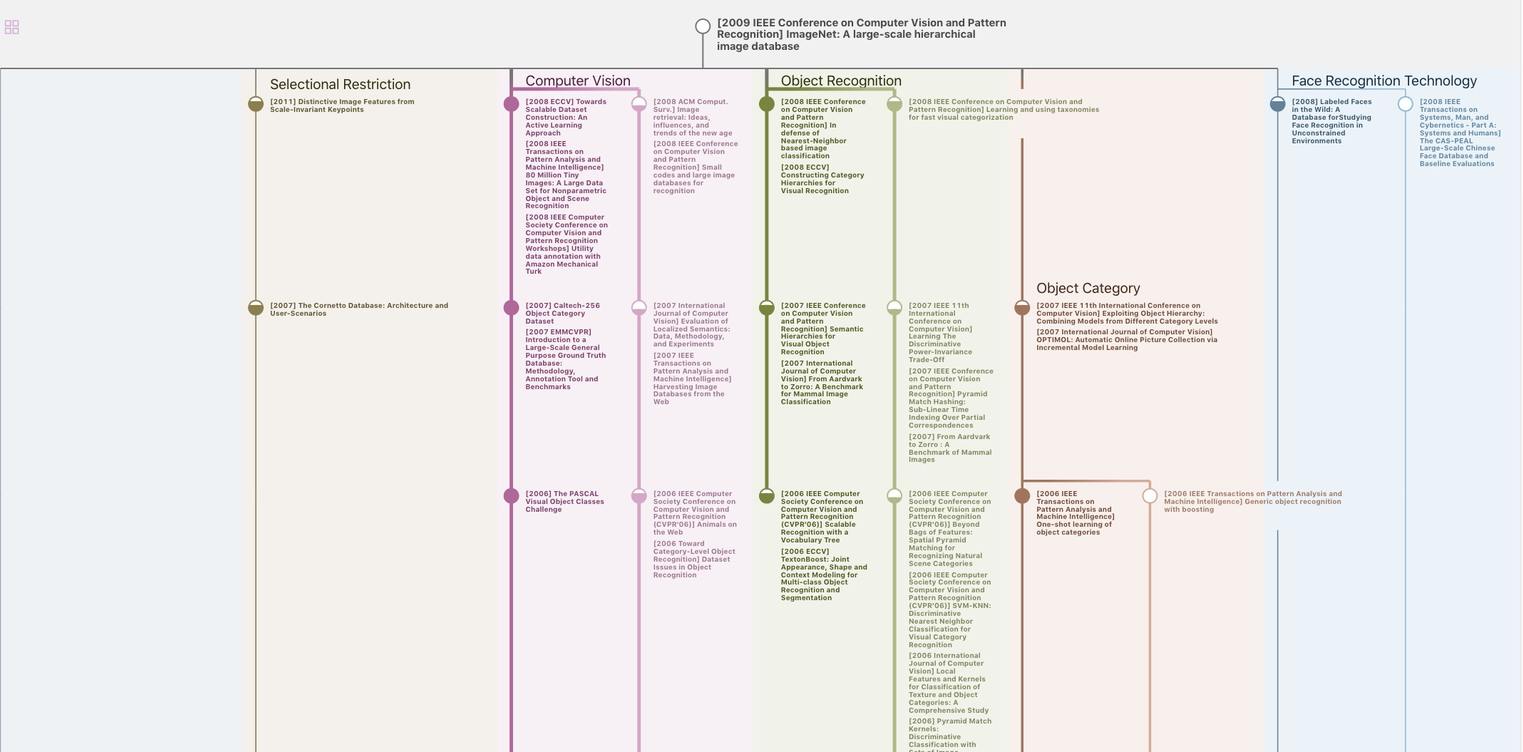
生成溯源树,研究论文发展脉络
Chat Paper
正在生成论文摘要