Combining Deep Learning And Rgbd Slam For Monocular Indoor Autonomous Flight
ADVANCES IN COMPUTATIONAL INTELLIGENCE, MICAI 2018, PT II(2018)
AI 理解论文
溯源树
样例
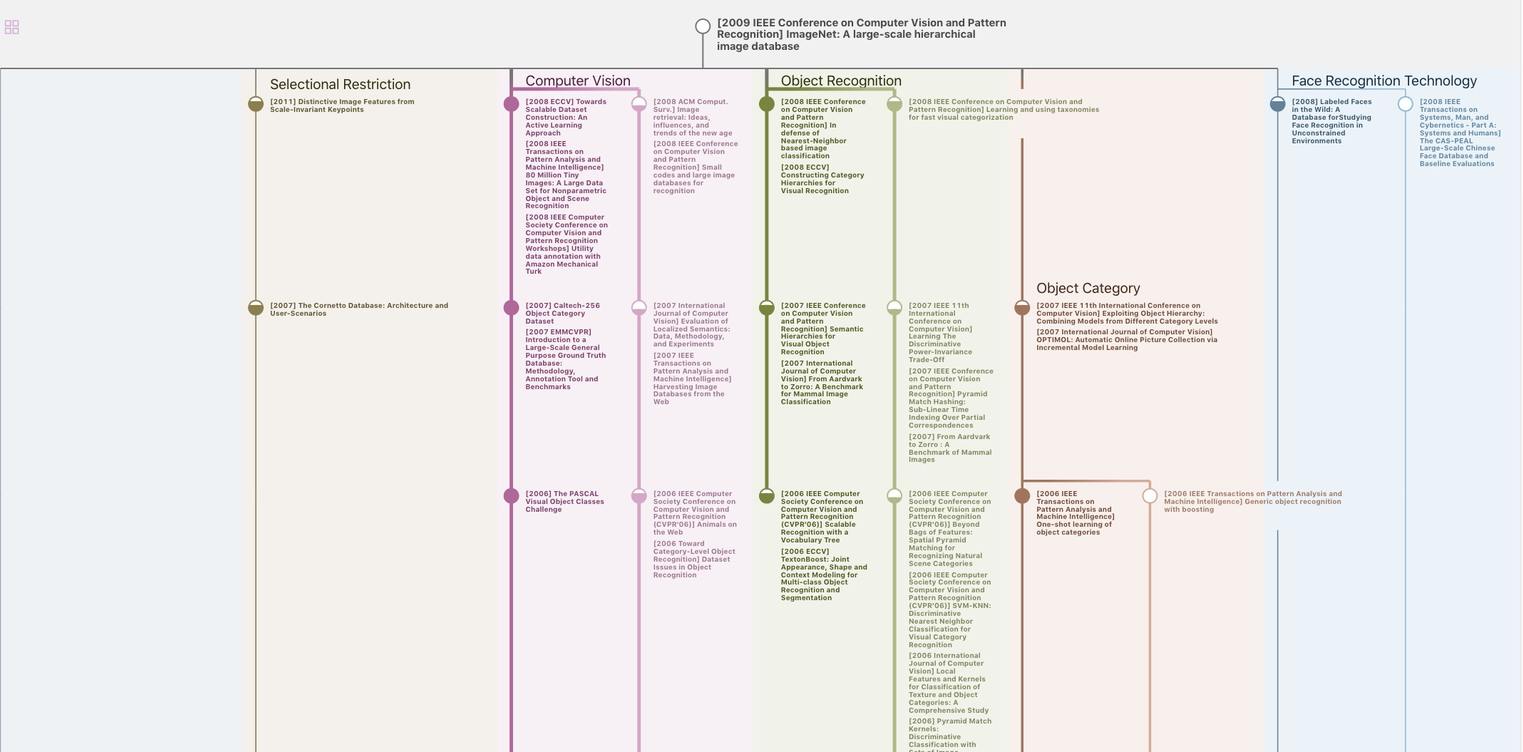
生成溯源树,研究论文发展脉络
Chat Paper
正在生成论文摘要
ADVANCES IN COMPUTATIONAL INTELLIGENCE, MICAI 2018, PT II(2018)