RGB-D Object Recognition Using Multi-Modal Deep Neural Network and DS Evidence Theory.
SENSORS(2019)
摘要
With the development of low-cost RGB-D (Red Green Blue-Depth) sensors, RGB-D object recognition has attracted more and more researchers' attention in recent years. The deep learning technique has become popular in the field of image analysis and has achieved competitive results. To make full use of the effective identification information in the RGB and depth images, we propose a multi-modal deep neural network and a DS (Dempster Shafer) evidence theory based RGB-D object recognition method. First, the RGB and depth images are preprocessed and two convolutional neural networks are trained, respectively. Next, we perform multi-modal feature learning using the proposed quadruplet samples based objective function to fine-tune the network parameters. Then, two probability classification results are obtained using two sigmoid SVMs (Support Vector Machines) with the learned RGB and depth features. Finally, the DS evidence theory based decision fusion method is used for integrating the two classification results. Compared with other RGB-D object recognition methods, our proposed method adopts two fusion strategies: Multi-modal feature learning and DS decision fusion. Both the discriminative information of each modality and the correlation information between the two modalities are exploited. Extensive experimental results have validated the effectiveness of the proposed method.
更多查看译文
关键词
RGB-D object recognition,deep neural network,multi-modal learning,DS evidence theory
AI 理解论文
溯源树
样例
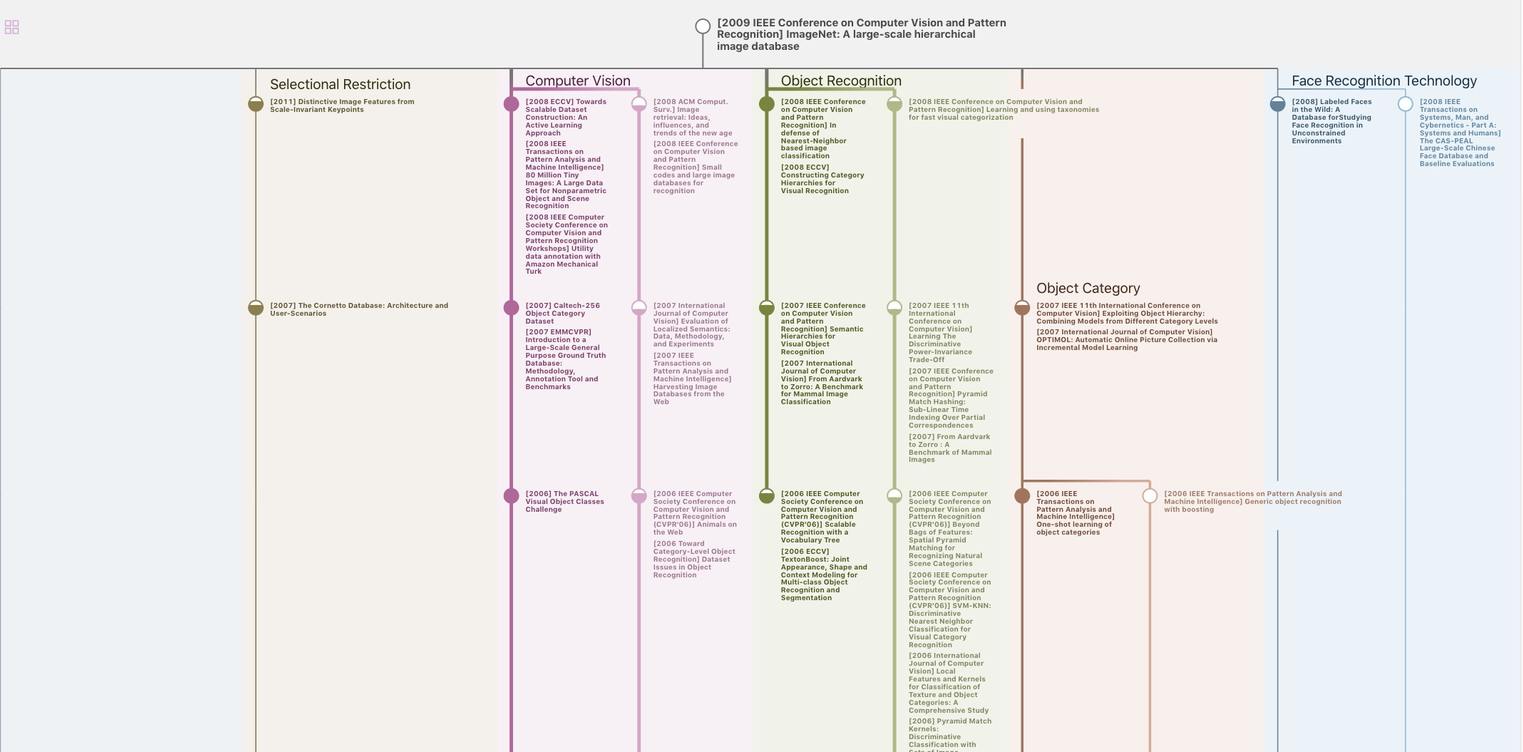
生成溯源树,研究论文发展脉络
Chat Paper
正在生成论文摘要