A Study of Data Fusion for Predicting Novel Activity in Enterprise Cyber-Security
2018 IEEE International Conference on Intelligence and Security Informatics (ISI)(2018)
摘要
Modern computer networks allow for the collection of vast amounts of data. A wide variety of sources record data relating to different aspects of computer and network activity. This wealth of available data, coupled with the persistent rise in successful cyber-security breaches, motivates the need for data-driven approaches to complement existing cyber-defence systems. Although obtainable, most of this data remains unexploited due to issues of data collection and privacy concerns. The majority of research has therefore been constrained to utilise limited data sets, usually obtained from only one of the many available data sources. We use a recently assembled public domain data set, which associates data from multiple sources in a real-world enterprise computer network, to demonstrate the advantages of data and entity fusion for cyber-security. We formulate an anomaly detection task employing time-delayed labels, which enables the use of supervised learning as a means of predicting novel activity. Our results show that an appropriate fusion of data from multiple sources and entities improves predictive accuracy.
更多查看译文
关键词
entity fusion,supervised learning,time-delayed labels,anomaly detection,cyber-defence systems,cyber-security breaches,real-world enterprise computer network,data sets,privacy concerns,data collection,data-driven approaches,network activity,enterprise cyber-security,data fusion,predictive accuracy
AI 理解论文
溯源树
样例
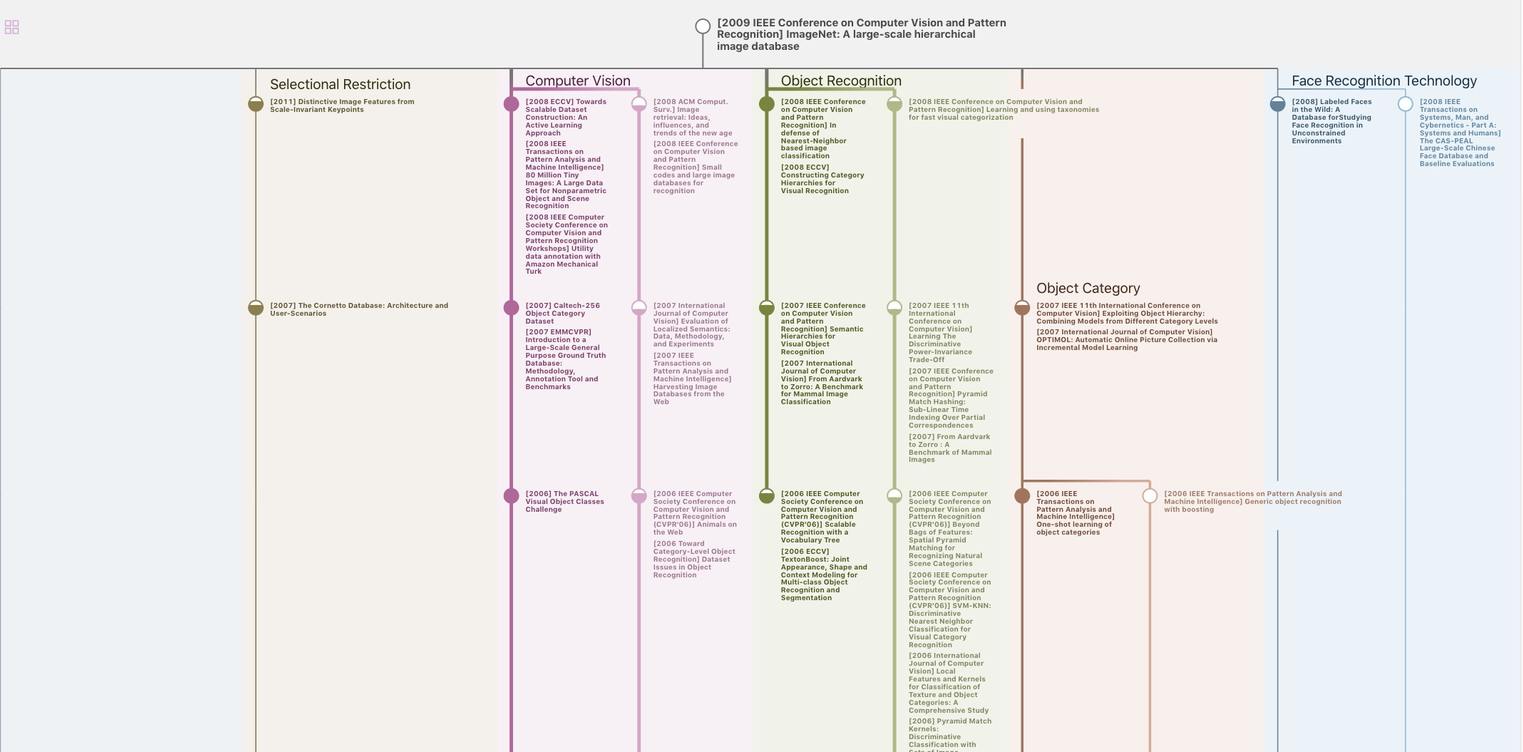
生成溯源树,研究论文发展脉络
Chat Paper
正在生成论文摘要