Cross-Domain Hallucination Network for Fine-Grained Object Recognition
PROCEEDINGS 2018 IEEE/CVF CONFERENCE ON COMPUTER VISION AND PATTERN RECOGNITION WORKSHOPS (CVPRW)(2018)
关键词
cross-domain,hallucination,fine-grained classification
AI 理解论文
溯源树
样例
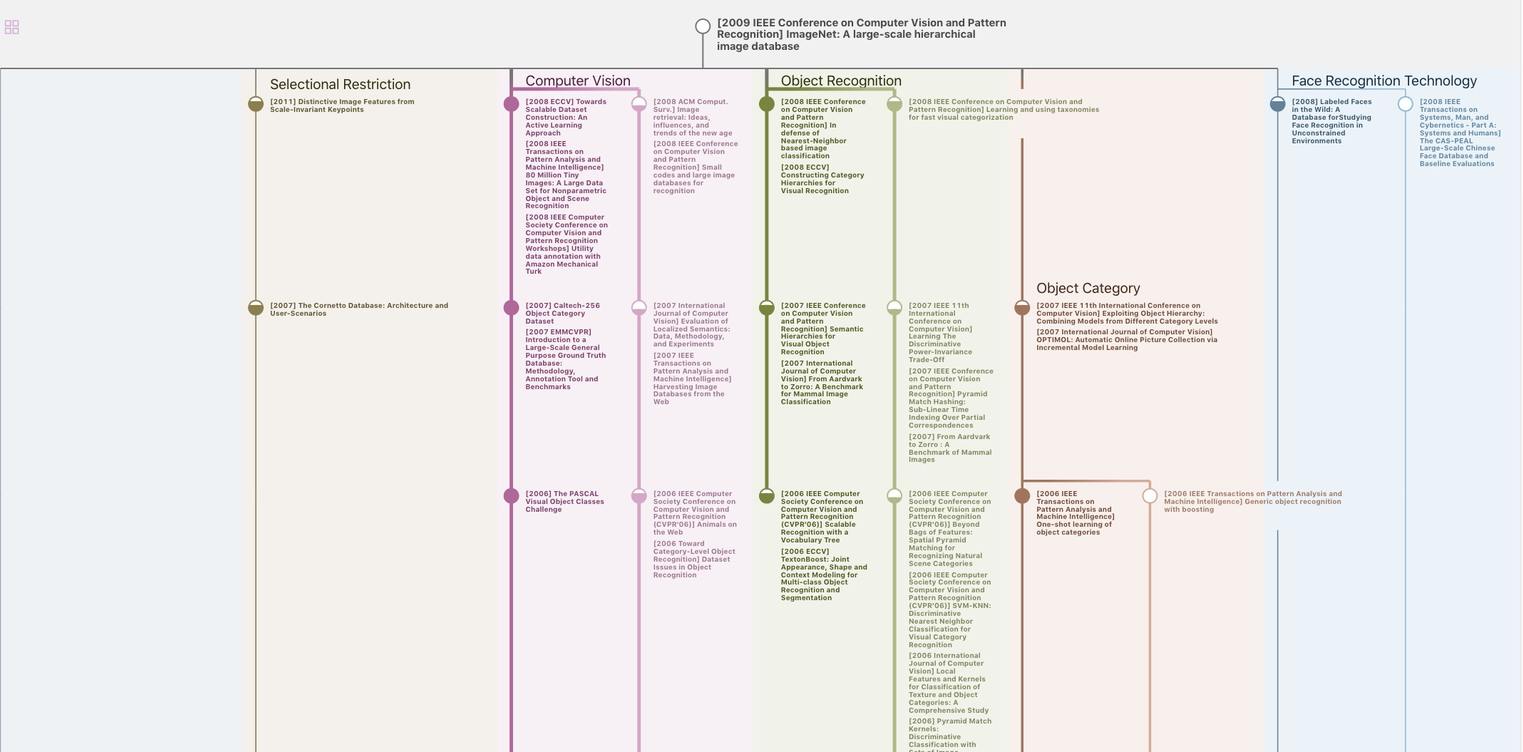
生成溯源树,研究论文发展脉络
Chat Paper
正在生成论文摘要