An Integrated Approach for Massive Sequential Data Processing in Civil Infrastructure Operation and Maintenance
IEEE Access(2018)
关键词
Civil infrastructure,massive data integration,sequential analysis,maintenance and operation management
AI 理解论文
溯源树
样例
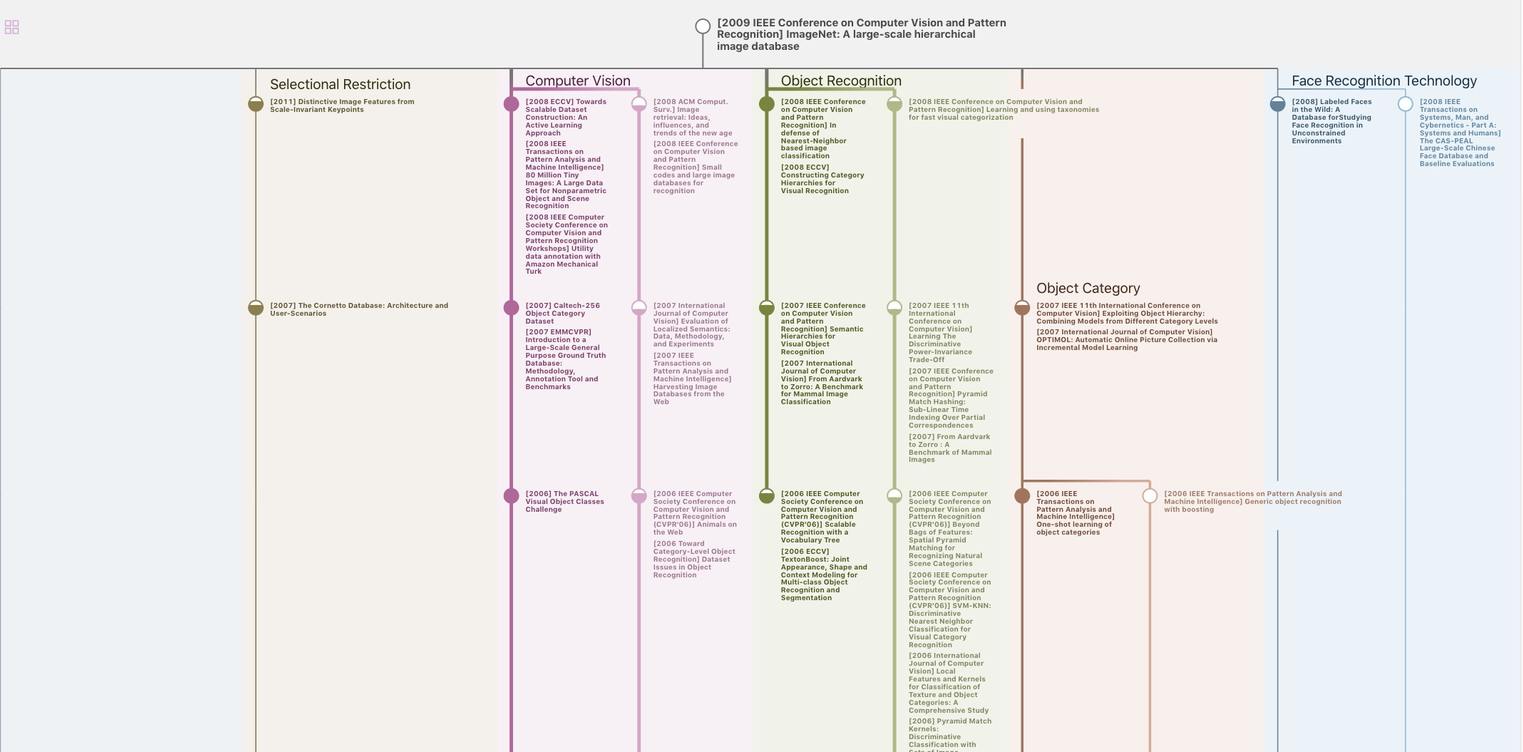
生成溯源树,研究论文发展脉络
Chat Paper
正在生成论文摘要