Effective feature fusion for pattern classification based on intra-class and extra-class discriminative correlation analysis
FUSION(2017)
摘要
Information fusion aims to exploit truthful knowledge from various sources in a reliable and accurate way. Fusion of information can be conducted at three abstraction levels including feature level, score level and decision level. The feature fusion approaches have the advantages of preserving effective discriminative structure underlying various features. In this paper, we propose an effective feature fusion algorithm based on intra-class and extra-class discriminative correlation analysis (IEDCA), aiming to eliminate between-class correlation and retain enough feature dimension for correlation analysis. IEDCA explores the intra-class correlation including both the pairs-wise correlation like CCA-based feature fusion approaches and the correlation across different features within the same class. Our proposed method can be used in unimodal feature fusion as well as multimodal feature fusion, and extensive experiments have proved its effectiveness.
更多查看译文
关键词
Feature fusion, canonical correlation analysis, pattern classification
AI 理解论文
溯源树
样例
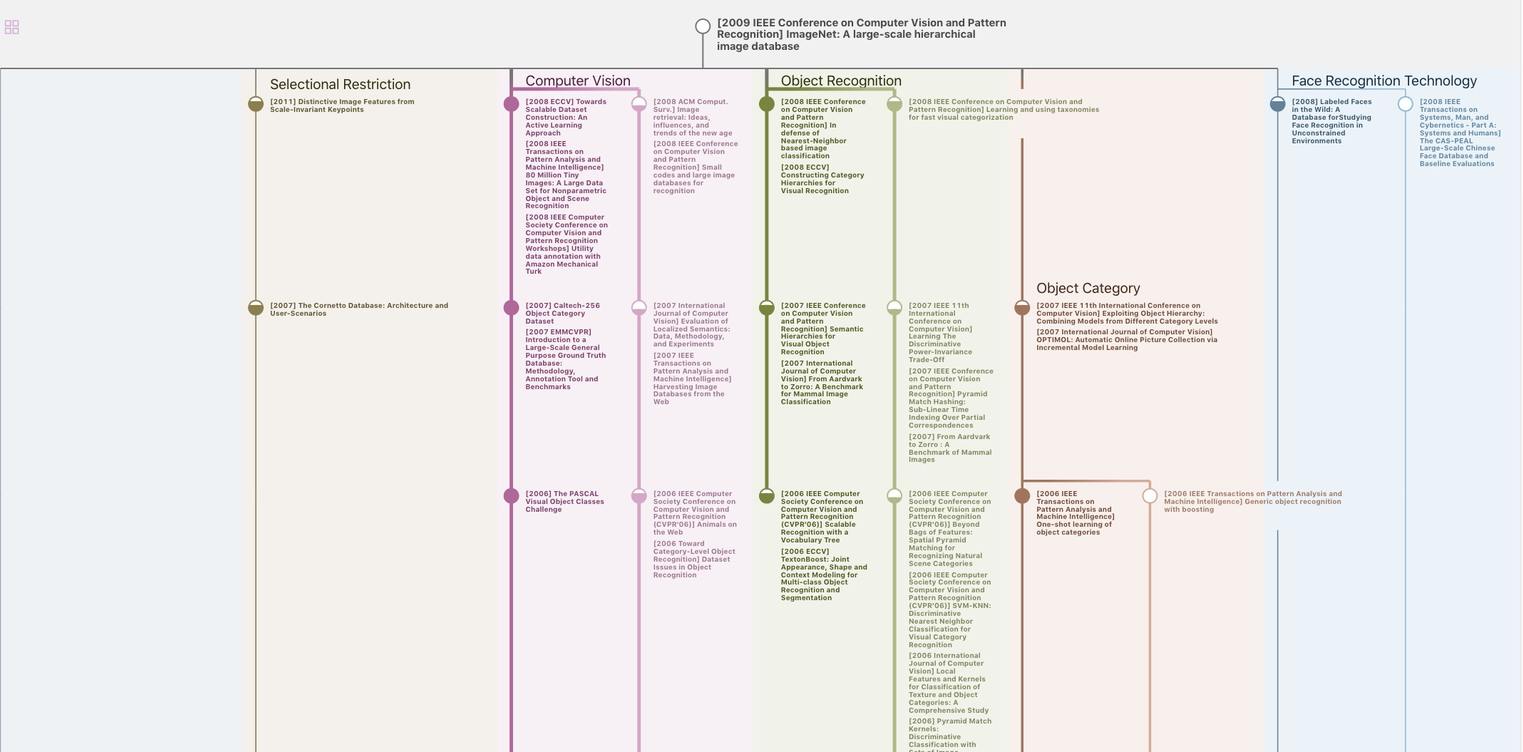
生成溯源树,研究论文发展脉络
Chat Paper
正在生成论文摘要