A hierarchical model for recognizing alarming states in a batteryless sensor alarm intervention for preventing falls in older people.
Pervasive and Mobile Computing(2017)
摘要
Falls are common among older people, especially in hospitals and nursing homes. The combination of pervasive sensing and statistical learning methods is creating new possibilities for automatic monitoring of activities of hospitalized older people to provide targeted and timely supervision by clinical staff to reduce falls. In this paper we introduce a hierarchical conditional random fields model to predict alarming states (being out of the bed or chair) from a passive wearable embodiment of a sensor worn over garment to provide an intervention mechanism to reduce falls. Our approach predicts alarm states in real time and avoids the use of empirically determined heuristics methods alone or in combination with machine learning based models, or multiple cascaded classifiers for generating alarms from activity prediction streams. Instead, the proposed hierarchical approach predicts alarms based on learned relationships between alarms, sensor information and predicted low-level activities. We evaluate the performance of the approach with 14 healthy older people and 26 hospitalized older patients and demonstrate similar or better performance than machine learning based approaches combined with heuristics based methods.
更多查看译文
关键词
Falls prevention,Hierarchical CRF,Human activity recognition,Bed exit,Chair exit
AI 理解论文
溯源树
样例
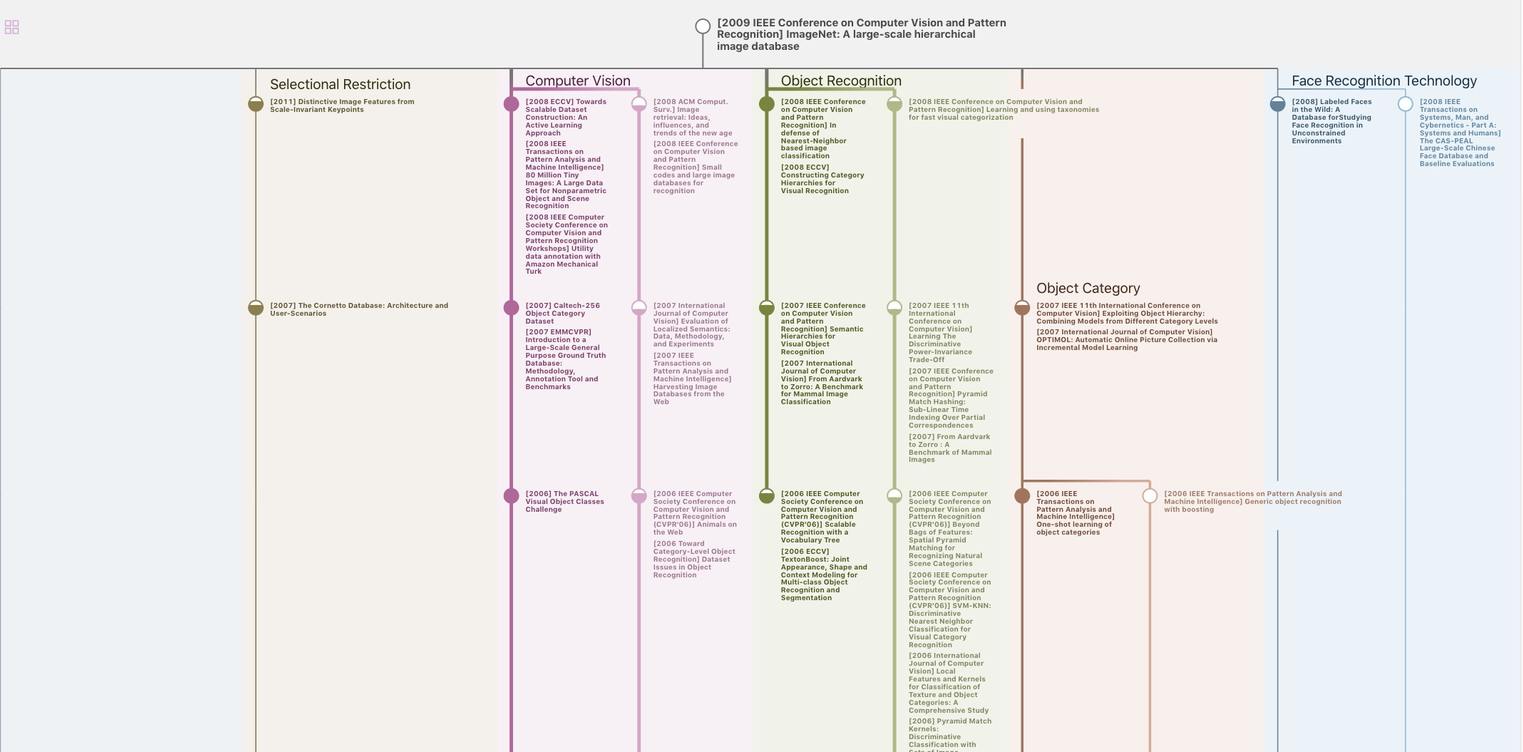
生成溯源树,研究论文发展脉络
Chat Paper
正在生成论文摘要