Automated cross layer feature selection for effective intrusion detection in networked systems.
IEEE Conference on Communications and Network Security(2016)
摘要
Traditionally, anomaly detection mechanisms have relied on the inspection of certain manually (by domain experts) chosen features in order to determine if a networked system is under attack or not. Unfortunately, the approach, while somewhat effective in flagging known attacks, yields either low true positive rates or high false positive rates when the attacks are mutated slightly or in the presence of zero day attacks. One can traditionally gather a lot of data at different layers (packet contents, application logs, OS behaviors, etc.) as evidence that could be used for intrusion detection. However, it is not easy to determine which of these evidence vectors or features are useful in facilitating highly accurate intrusion detection. In this paper, we undertake an in-depth experimental study to determine whether appropriately trained search algorithms can help us find the right set of features for detecting a class of attacks (e.g., denial of service). The output of such algorithms yields a set of features that should potentially improve detection accuracy. Towards this we monitor 365 features across system layers and compare the detection performance of 3 popular feature selection algorithms to reduce the state space of the feature set for two classes of attacks. We find that the approach can yield significantly improved detection accuracy in comparison to statically chosen single features, sub or super sets of features of what the algorithms yield.
更多查看译文
关键词
automated cross layer feature selection,intrusion detection,networked systems,anomaly detection mechanisms,flagging known attacks,zero day attacks,packet contents,application logs,OS behaviors,trained search algorithms,denial of service attack
AI 理解论文
溯源树
样例
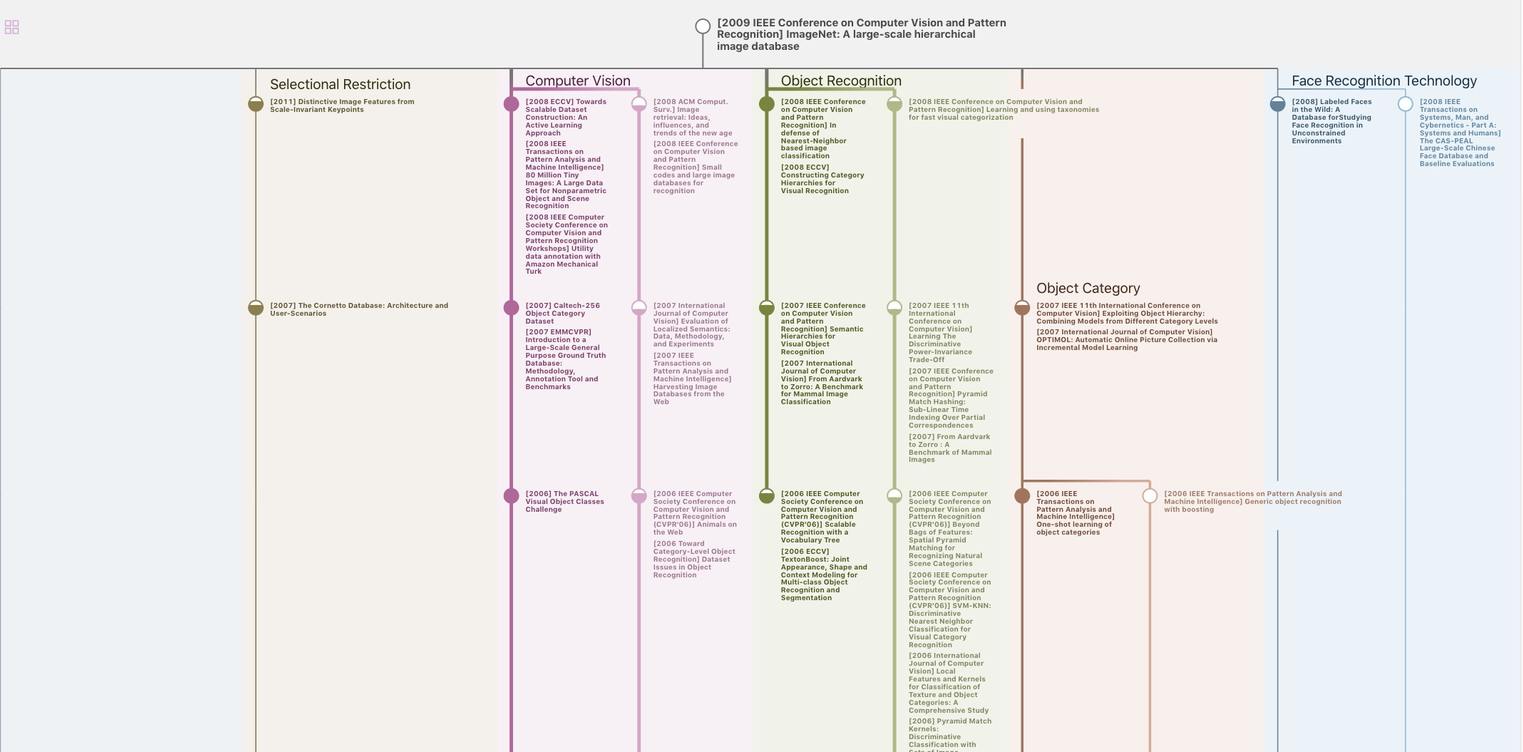
生成溯源树,研究论文发展脉络
Chat Paper
正在生成论文摘要