Continuously Improving the Resource Utilization of Iterative Parallel Dataflows
2016 IEEE 36th International Conference on Distributed Computing Systems Workshops (ICDCSW)(2016)
摘要
Parallel dataflow systems like Apache Flink allow analysis of large datasets with iterative programs. However, allocating a cost-effective set of resources for such jobs is a difficult task as the resource utilization depends on many factors such as dataset size, key value distributions, computational complexity of programs, and the underlying hardware. What's more, some of these factors are not well known before the execution. There are, for example, often no data statistics such as key value distributions available beforehand. For this reason, we propose to improve the resource utilization at runtime using the repetitive nature of iterative dataflow programs. Based on runtime statistics gathered in previous iterations, the resource allocation is adapted dynamically at the synchronization barriers between iterations. This approach has two advantages: First, at barriers detailed statistics can be available, even for parallelly executed task pipelines. Second, at barriers dataflows can be adapted without complex handling of intermediate task state. This paper presents a prototype integrated with Apache Flink and an evaluation on a cluster with 480 cores. One experiment shows a 57% reduction of the job runtime by allocating more resources for a shorter time, another experiment a release of up to 40% surplus resources without significantly extending the job runtime.
更多查看译文
关键词
Parallel Dataflows,Scalable Data Processing,Re-source Utilization,Dynamic Scaling
AI 理解论文
溯源树
样例
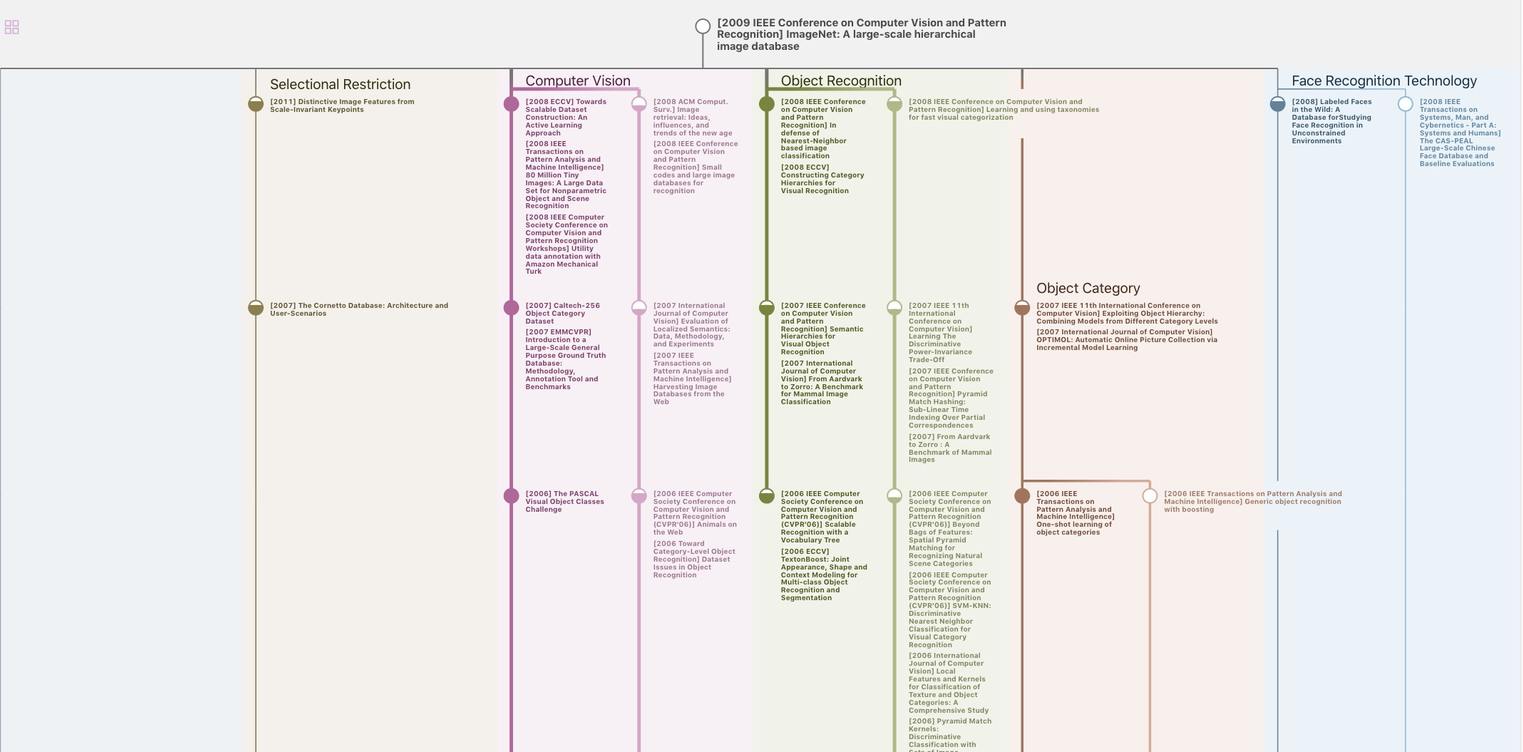
生成溯源树,研究论文发展脉络
Chat Paper
正在生成论文摘要