Limitations of Using Constraint Set Utility in Semi-Supervised Clustering
MetaSel'15 Proceedings of the 2015 International Conference on Meta-Learning and Algorithm Selection - Volume 1455(2015)
AI Read Science
Must-Reading Tree
Example
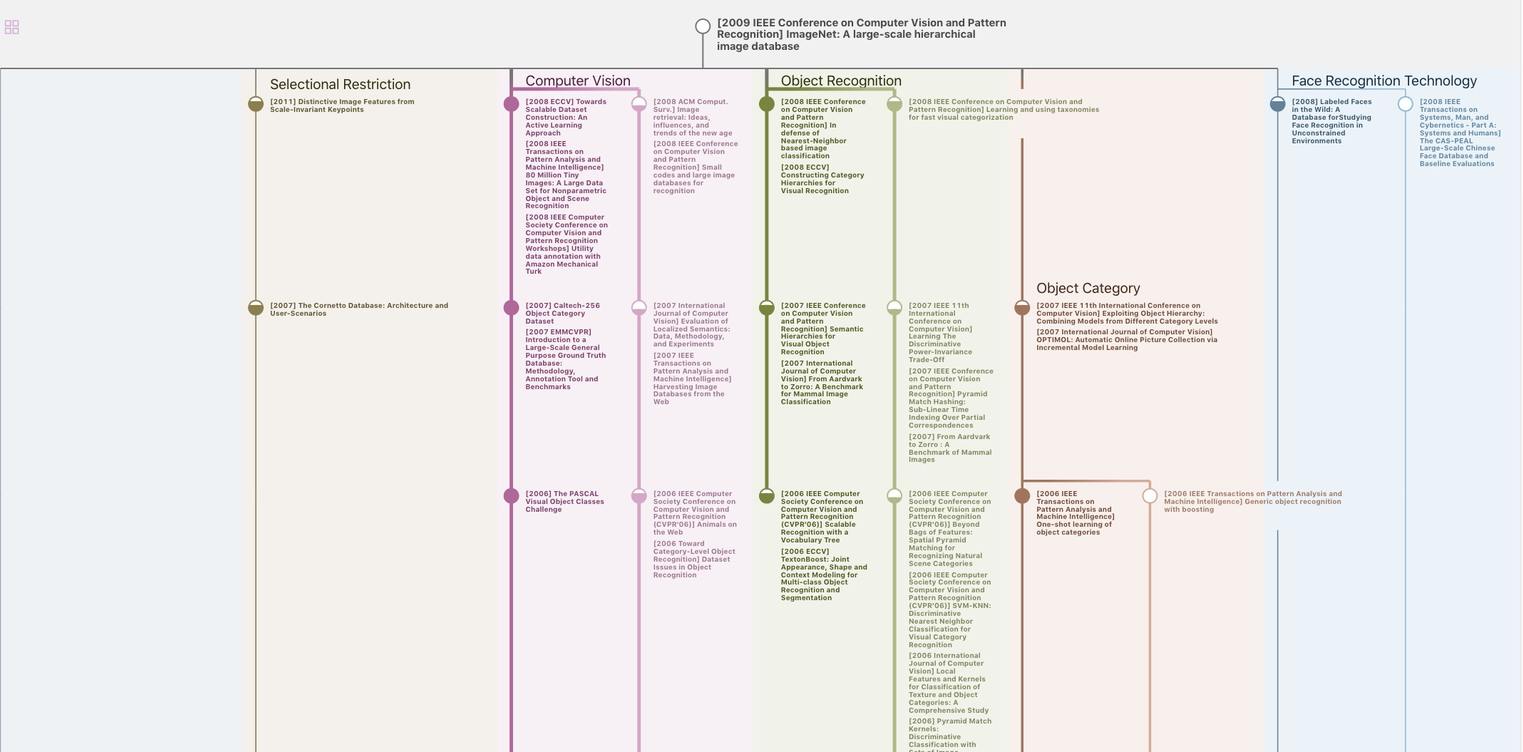
Generate MRT to find the research sequence of this paper
Chat Paper
Summary is being generated by the instructions you defined