A Comprehensive Computer-Aided Polyp Detection System for Colonoscopy Videos.
Lecture Notes in Computer Science Information Processing in Medical Imaging(2015)
Key words
Discrete Cosine Transform,Candidate Location,Convolutional Neural Network,Vote Scheme,Discrete Cosine Transform Coefficient
AI Read Science
Must-Reading Tree
Example
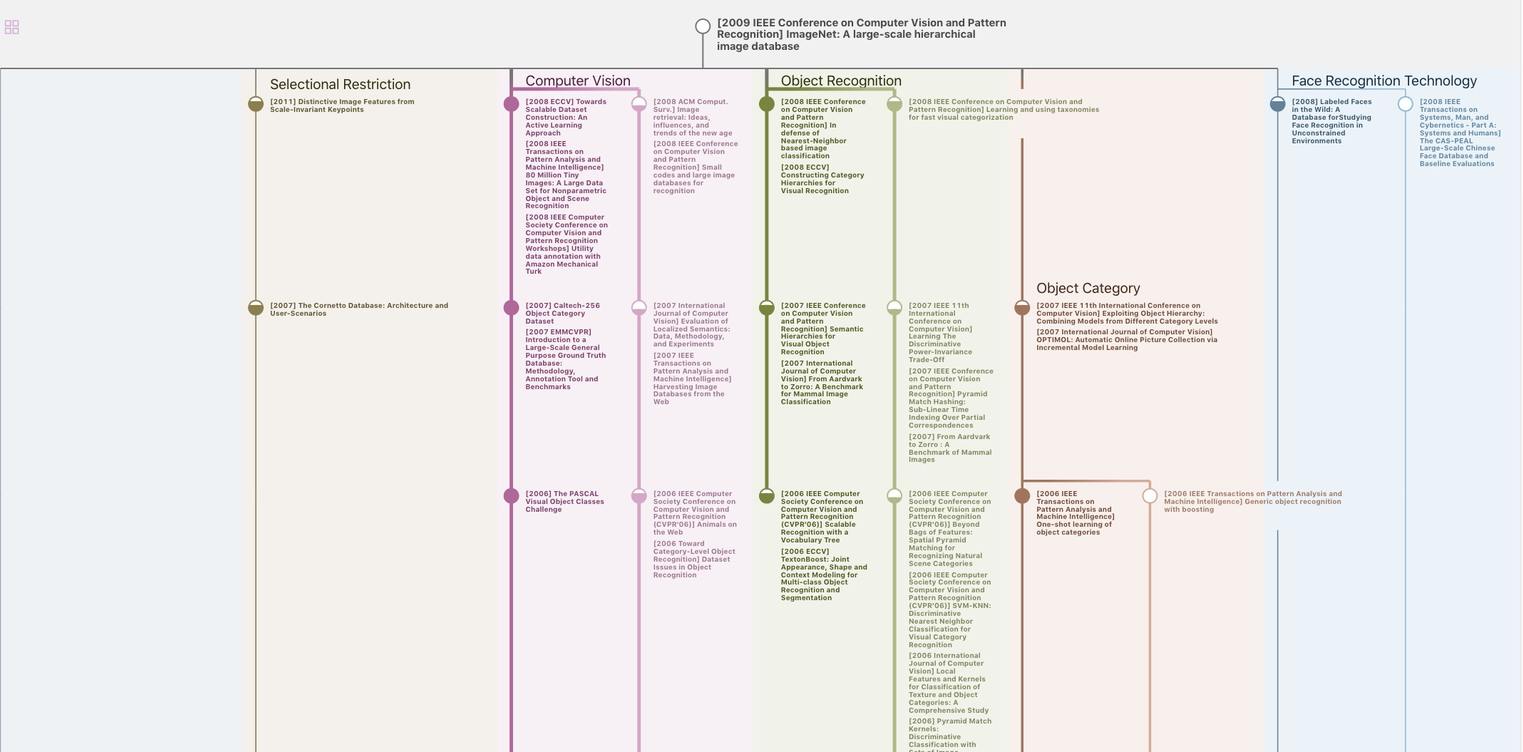
Generate MRT to find the research sequence of this paper
Chat Paper
Summary is being generated by the instructions you defined