Learning Hierarchical Representation Model for NextBasket Recommendation.
Annual International ACM SIGIR Conference on Research and Development in Information Retrieval(2015)
Key words
Hierarchical Representation Model,Sequential Behavior,General Taste,Next Basket Recommendation
AI Read Science
Must-Reading Tree
Example
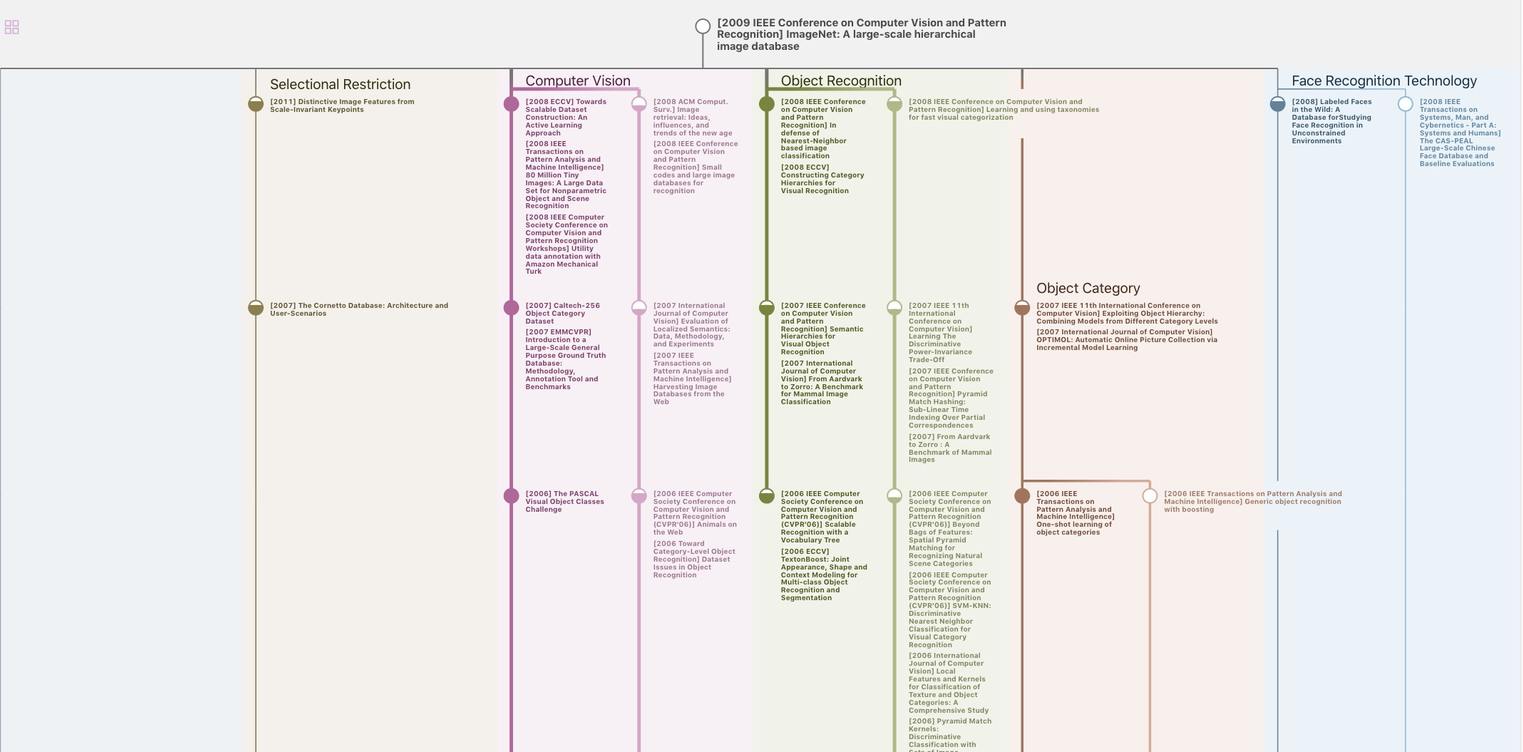
Generate MRT to find the research sequence of this paper
Chat Paper
Summary is being generated by the instructions you defined