Machine Learning Based Automatic Detection Of Pulmonary Trunk
MEDICAL IMAGING 2011: COMPUTER-AIDED DIAGNOSIS(2011)
Key words
Pulmonary Embolism,Pulmonary Trunk,Automatic machine learning based detection,Haar,AdaBoost,Cascade
AI Read Science
Must-Reading Tree
Example
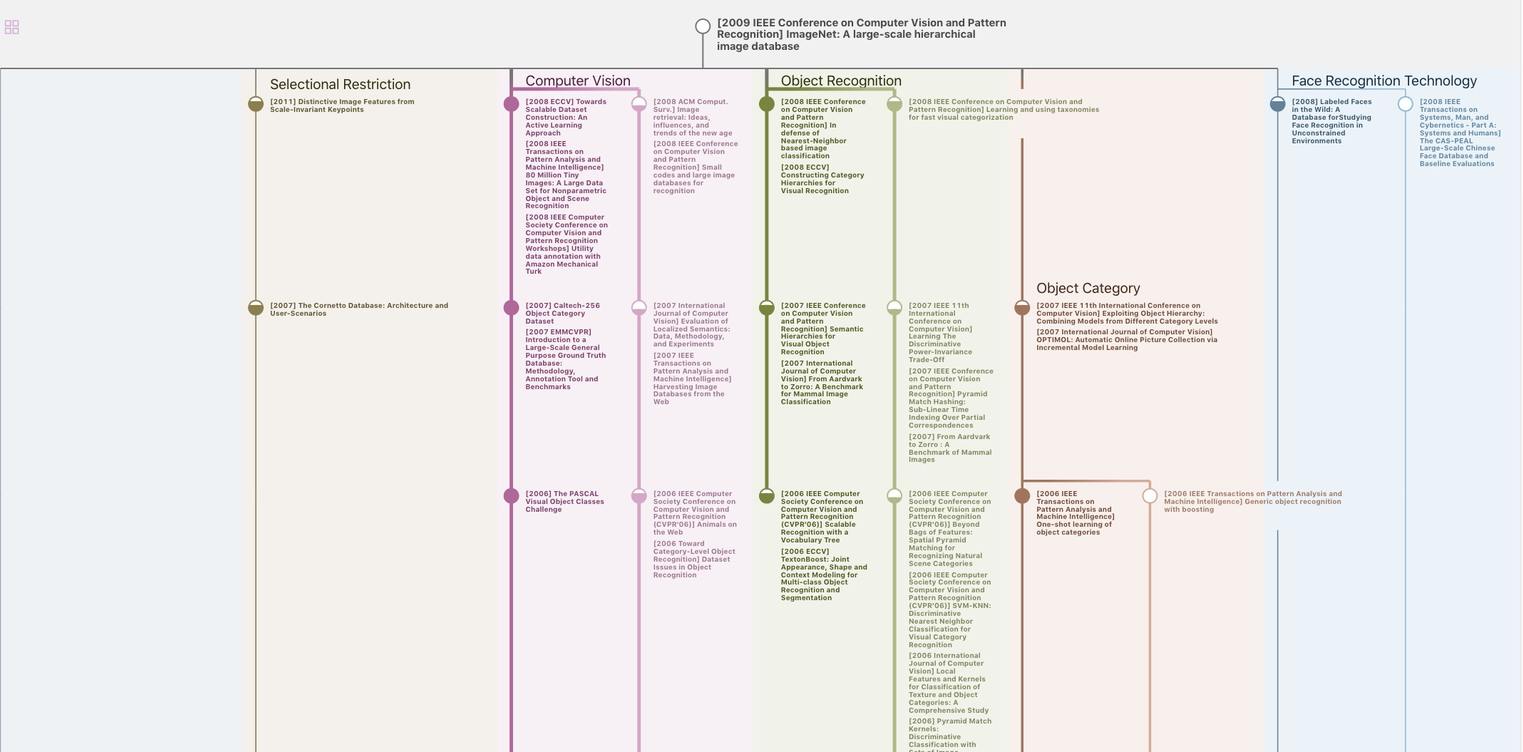
Generate MRT to find the research sequence of this paper
Chat Paper
Summary is being generated by the instructions you defined