RESONATE: Reverberation environment simulation for improved classification of speech models
Berlin(2014)
摘要
Home monitoring systems currently gather information about peoples activities of daily living and information regarding emergencies, however they currently lack the ability to track speech. Practical speech analysis solutions are needed to help monitor ongoing conditions such as depression, as the amount of social interaction and vocal affect is important for assessing mood and well-being. Although there are existing solutions that classify the identity and the mood of a speaker, when the acoustic signals are captured in reverberant environments they perform poorly. In this paper, we present a practical reverberation compensation method called RESONATE, which uses simulated room impulse responses to adapt a training corpus for use in multiple real reverberant rooms. We demonstrate that the system creates robust classifiers that perform within 5 -- 10% of baseline accuracy of non-reverberant environments. We demonstrate and evaluate the performance of this matched condition strategy using a public dataset, and also in controlled experiments with six rooms, and two long-term and uncontrolled real deployments. We offer a practical implementation that performs collection, feature extraction, and classification on-node, and training and simulation of training sets on a base station or cloud service.
更多查看译文
关键词
feature extraction,reverberation,speech synthesis,RESONATE,acoustic signals,base station,classification on-node,cloud service,feature extraction,home monitoring systems,matched condition strategy,public dataset,reverberant rooms,reverberation compensation method,reverberation environment simulation,robust classifiers,social interaction,speech analysis solutions,speech model classification,training sets simulation,vocal affect,Reverberation Compensation,Speaker Identification
AI 理解论文
溯源树
样例
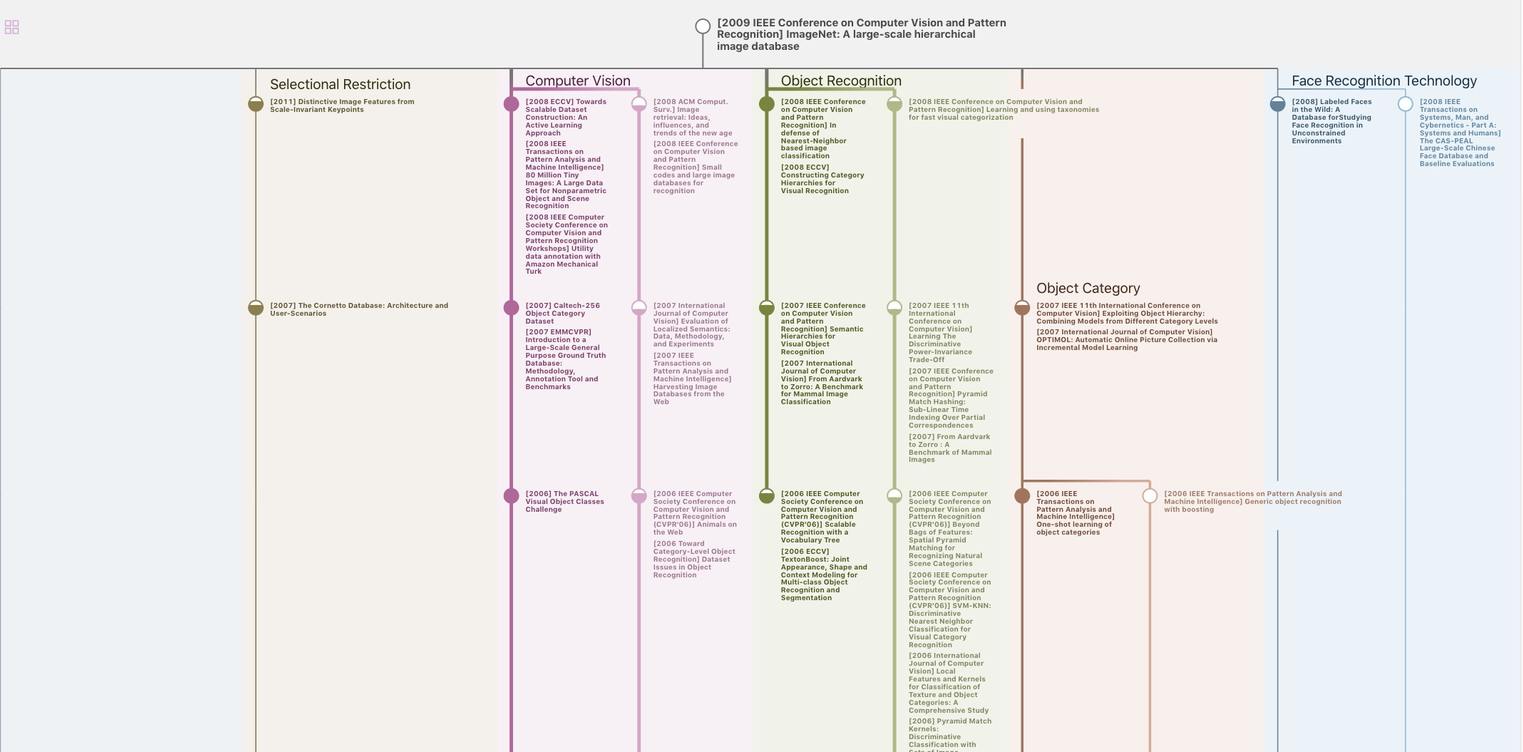
生成溯源树,研究论文发展脉络
Chat Paper
正在生成论文摘要