A Decoder-Based Spike Train Metric for Analyzing the Neural Code in the Retina
Frontiers in Systems Neuroscience(2009)
AI Read Science
Must-Reading Tree
Example
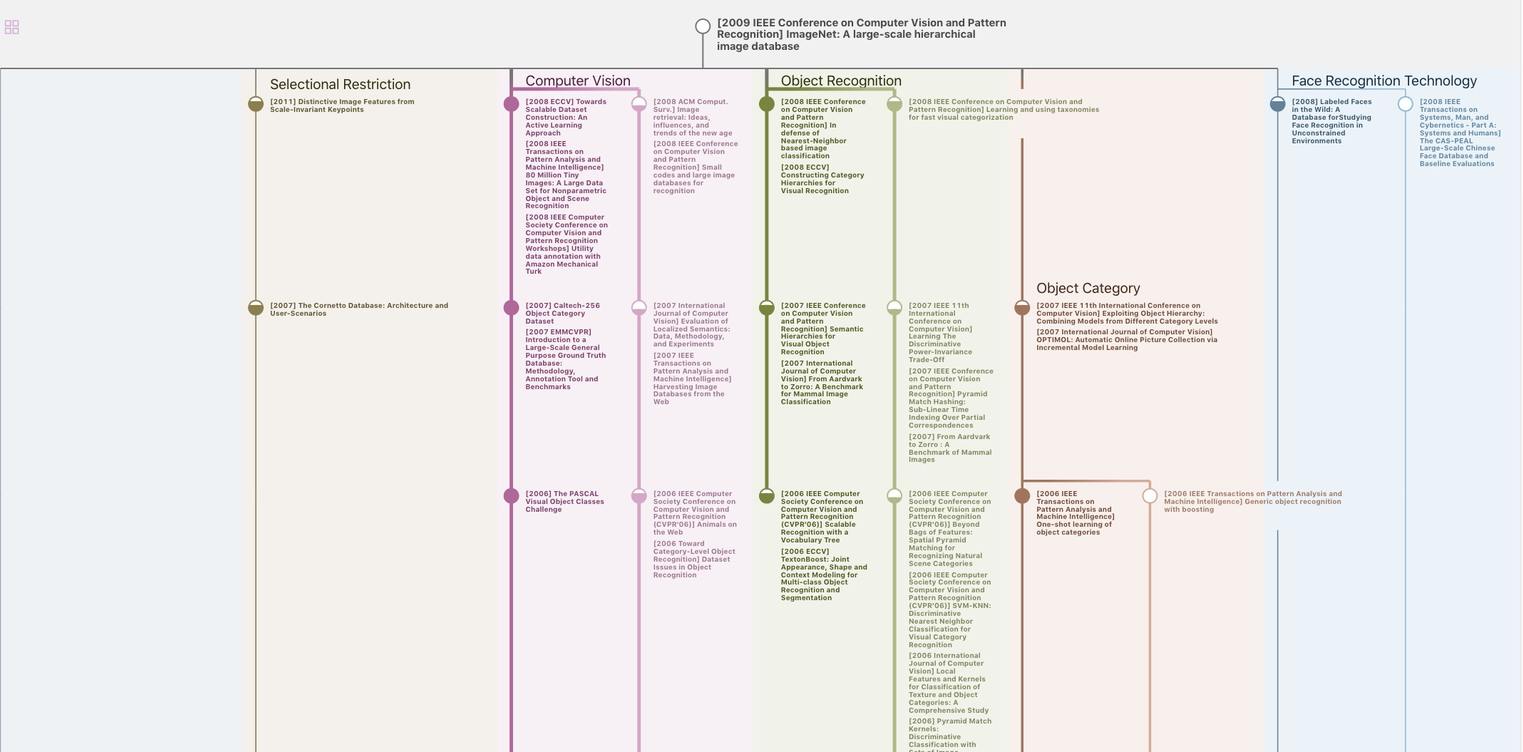
Generate MRT to find the research sequence of this paper
Chat Paper
Summary is being generated by the instructions you defined