Complexity Measure as a Feature to Classify Schizophrenic and Healthy Participants
Computer Modelling and Simulation(2012)
摘要
Nonlinear analysis of electroencephalogram (EEG) signals reflects new information in understanding of brain activity. Here, a complicated signal like EEG is considered as an output of a nonlinear dynamic system (brain). The intrinsic changes of the EEG signals are described as the variation of their fractal dimension. In this study, EEG signal complexity of ten schizophrenic patients and ten age-matched healthy participants are analyzed in two different approaches, time-domain and phase-space. In the time-domain approach, three popular methods are used to compute the complexity. In the second approach, the chaotic dynamical attractors expressed in the phase space are reconstructed and their correlation dimension (D2) which is a measure of the complexity is calculated. In this study, an efficient algorithm to calculate a time-varying dimension estimate is applied. The results indicate a diminished complexity in the EEGs of the schizophrenic patients and confirm findings associated with the fact that schizophrenic patients are characterized by less complex neurobehavioral measures than healthy participants. Finally, Support Vector Machine (SVM) is applied on the estimated fractal dimension and results show 86.4%, 81.0%, 73.9% and 70.4% classification accuracy using correlation, Higuchi, Katz and Petrosian respectively.
更多查看译文
关键词
healthy participants,correlation dimension,time-varying dimension estimate,age-matched healthy participant,fractal dimension,estimated fractal dimension,complexity measure,classify schizophrenic,brain activity,schizophrenic patient,eeg signal,eeg signal complexity,diminished complexity,electroencephalography,svm,schizophrenia,support vector machine,phase space,support vector machines,eeg,vectors,correlation,time domain,computational complexity,fractals
AI 理解论文
溯源树
样例
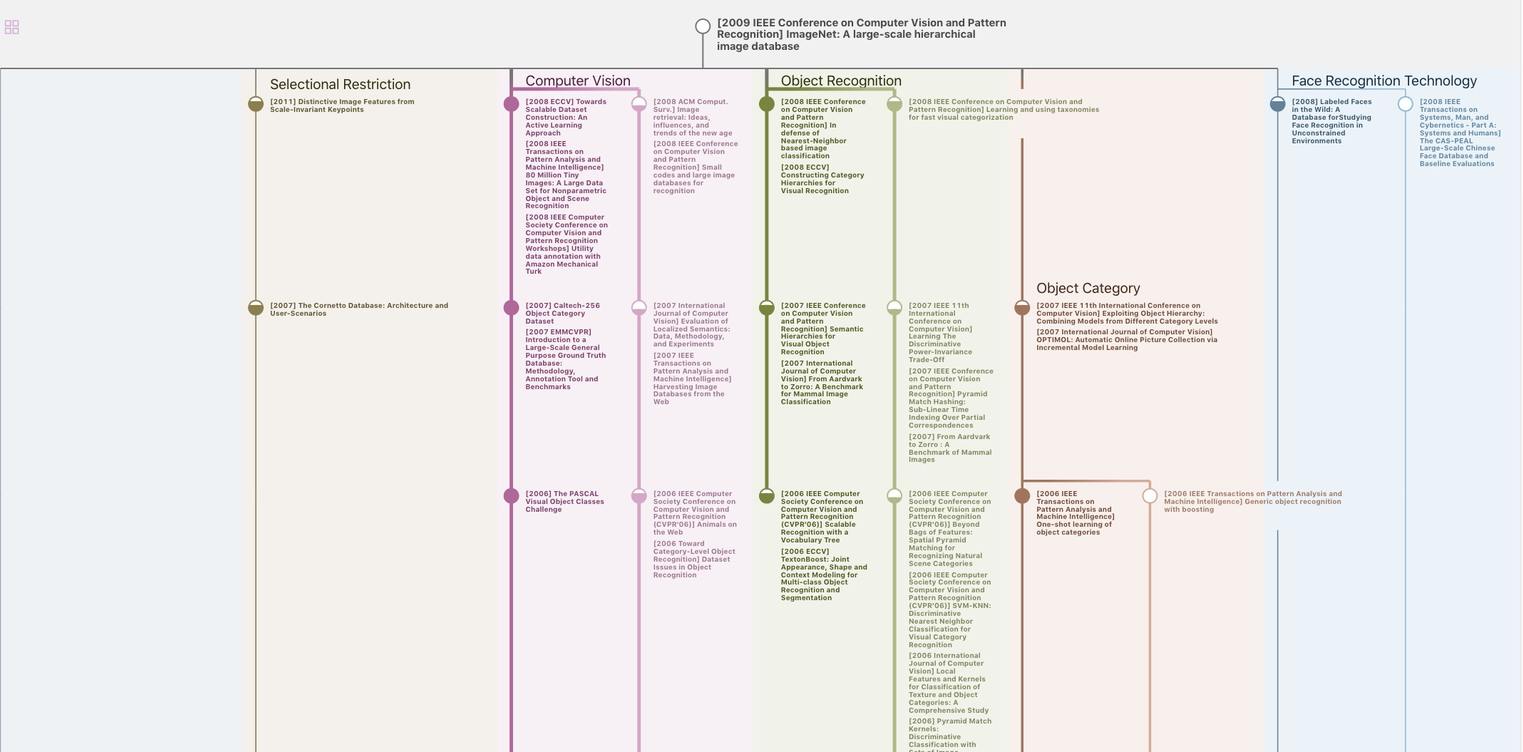
生成溯源树,研究论文发展脉络
Chat Paper
正在生成论文摘要