Efficient and Robust Nonlocal Means Denoising of MR Data Based on Salient Features Matching
Computer Methods and Programs in Biomedicine(2011)
Key words
Nonlocal means,Image denoising,Magnetic resonance imaging
AI Read Science
Must-Reading Tree
Example
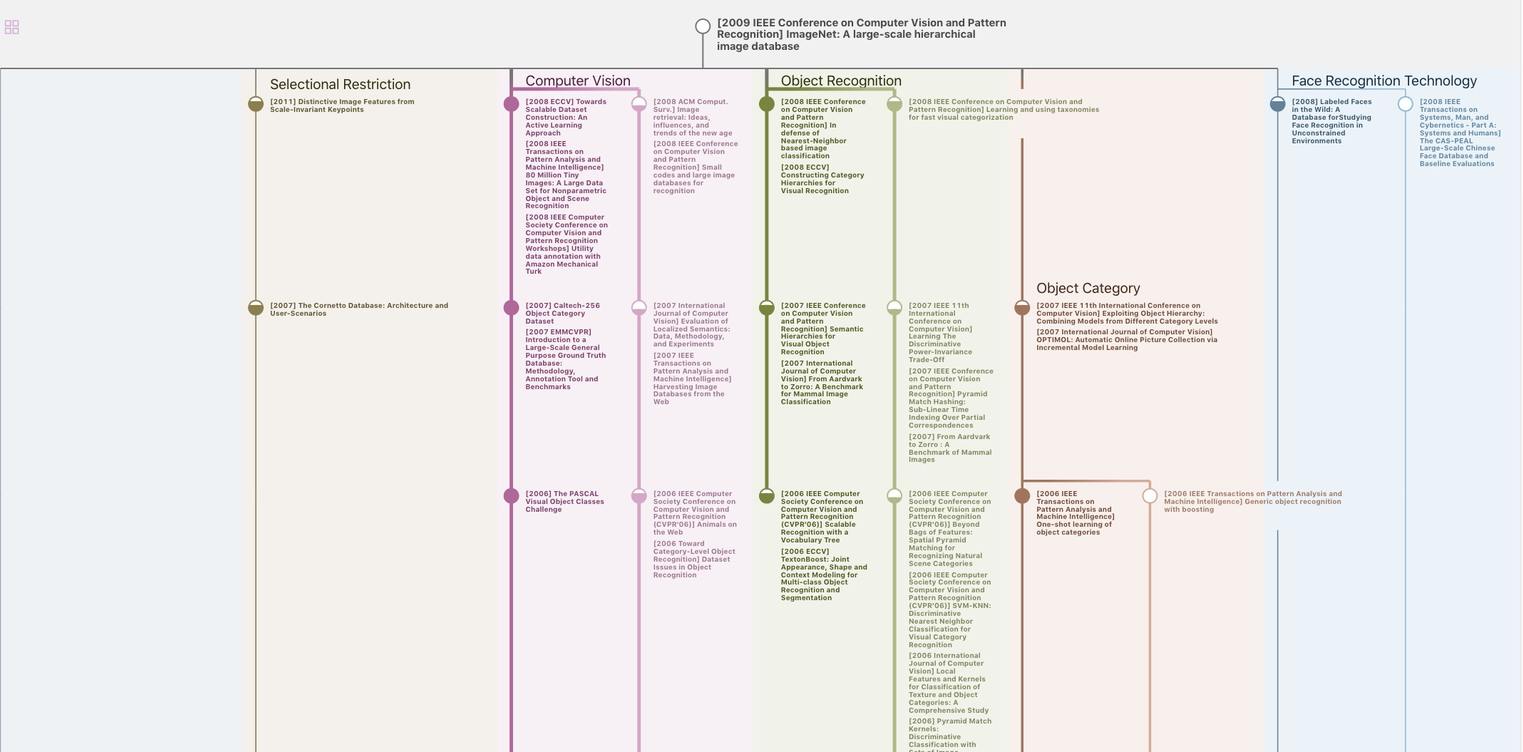
Generate MRT to find the research sequence of this paper
Chat Paper
Summary is being generated by the instructions you defined