Discriminating DDoS Flows from Flash Crowds Using Information Distance
Gold Coast, QLD(2009)
摘要
Discriminating DDoS flooding attacks from flash crowds poses a tough challenge for the network security community. Because of the vulnerability of the original design of the Internet, attackers can easily mimic the patterns of legitimate network traffic to fly under the radar. The existing fingerprint or feature based algorithms are incapable to detect new attack strategies. In this paper, we aim to differentiate DDoS attack flows from flash crowds. We are motivated by the following fact: the attack flows are generated by the same prebuilt program (attack tools), however, flash crowds come from randomly distributed users all over the Internet. Therefore, the flow similarity among DDoS attack flows is much stronger than that among flash crowds. We employ abstract distance metrics, the Jeffrey distance, the Sibson distance, and the Hellinger distance to measure the similarity among flows to achieve our goal. We compared the three metrics and found that the Sibson distance is the most suitable one for our purpose. We apply our algorithm to the real datasets and the results indicate that the proposed algorithm can differentiate them with an accuracy around 65%.
更多查看译文
关键词
network security,ddos attack,attack flow,flash crowd,flooding attacks,flash crowds,discriminating ddos flows,legitimate network traffic,sibson distance,jeffrey distance,new attack strategy,fingerprint algorithms,attack tool,ddos attack flow,discriminating ddos flooding attack,information distance,hellinger distance,internet,abstract distance metrics,flow similarity,measurement,distance,security of data,feature based algorithms,distance metric,algorithm design and analysis,data mining,detectors,sensitivity
AI 理解论文
溯源树
样例
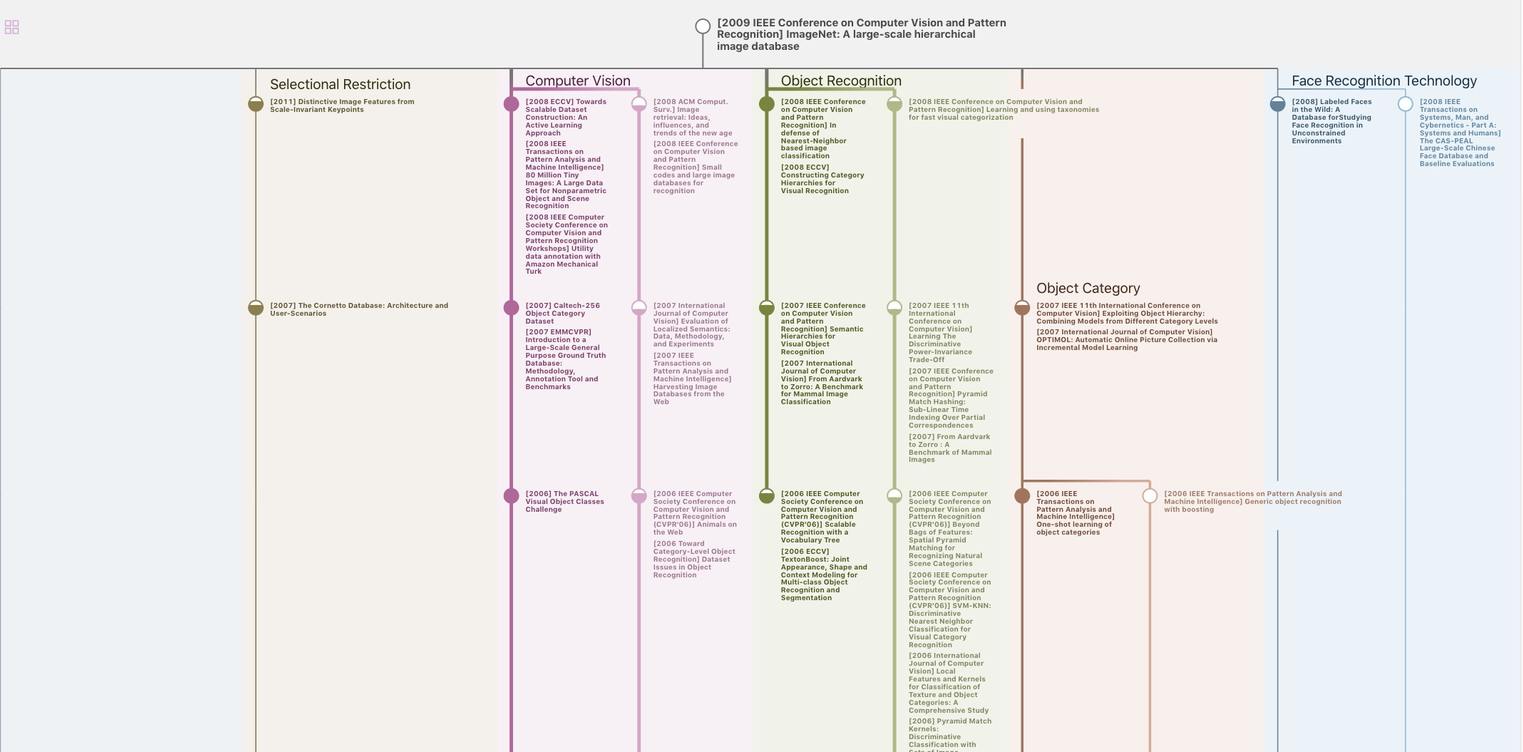
生成溯源树,研究论文发展脉络
Chat Paper
正在生成论文摘要