Continuous hidden process model for time series expression experiments.
BIOINFORMATICS(2007)
摘要
When analyzing expression experiments, researchers are often interested in identifying the set of biological processes that are up- or down-regulated under the experimental condition studied. Current approaches, including clustering expression profiles and averaging the expression profiles of genes known to participate in specific processes, fail to provide an accurate estimate of the activity levels of many biological processes.We introduce a probabilistic continuous hidden process Model (CHPM) for time series expression data. CHPM can simultaneously determine the most probable assignment of genes to processes and the level of activation of these processes over time. To estimate model parameters, CHPM uses multiple time series datasets and incorporates prior biological knowledge. Applying CHPM to yeast expression data, we show that our algorithm produces more accurate functional assignments for genes compared to other expression analysis methods. The inferred process activity levels can be used to study the relationships between biological processes. We also report new biological experiments confirming some of the process activity levels predicted by CHPM.A Java implementation is available at http:\\www.cs.cmu.edu\~yanxins\chpm.Supplementary data are available at Bioinformatics online.
更多查看译文
关键词
time series expression experiment,expression experiment,biological knowledge,continuous hidden process model,new biological experiment,biological process,time series expression data,expression analysis method,clustering expression profile,process activity level,expression profile,yeast expression data,process model,time series
AI 理解论文
溯源树
样例
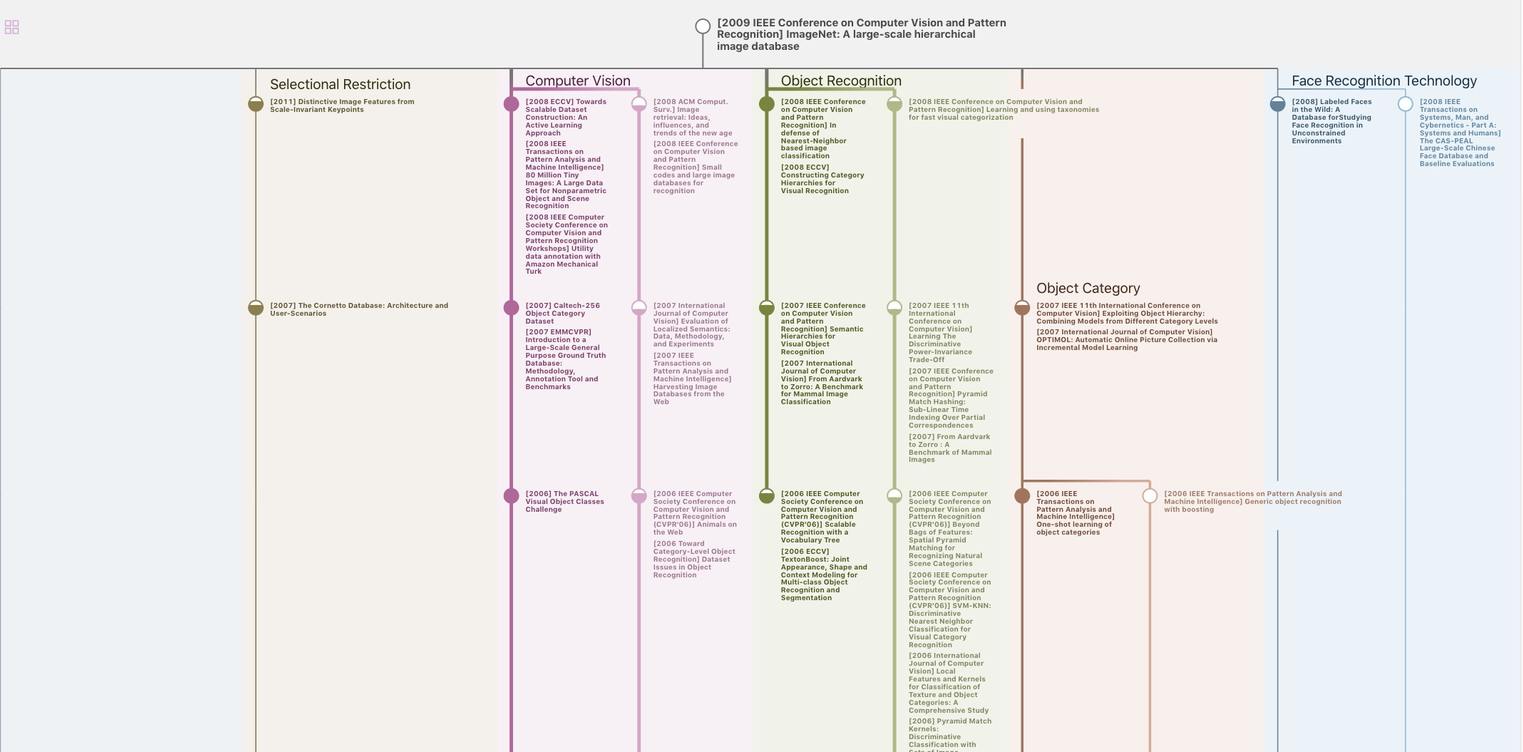
生成溯源树,研究论文发展脉络
Chat Paper
正在生成论文摘要