Quantitative study of rainfall lag effects and integration of machine learning methods for groundwater level prediction modelling
Hydrological Processes(2024)
摘要
AbstractGroundwater level (GWL) is a significant indicator for quantifying groundwater availability. Currently, hydrologists worldwide are actively engaged in modelling and predicting GWL. In karst regions, GWL exhibit varying responses to rainfall events across different locations and the impact of rainfall events on GWL within the same location also varies. Despite incorporating rainfall as an input variable, most existing data‐driven GWL prediction models inadequately account for the spatio‐temporal heterogeneity of karst water areas. Therefore, this study proposes a new analysis method to investigate the response patterns of GWL to rainfall events in karst regions with typical spatio‐temporal variations, known as the sensitivity analysis of rainfall‐GWL response. The method introduces the rainfall response coefficient to describe the response characteristics of GWL to rainfall. Through the rainfall response coefficient, the rainfall response variable (RR) is calculated and incorporates it as an input in the RR‐long short‐term memory (LSTM) GWL prediction model. The effectiveness of proposed method was validated by GWL prediction in karst aquifers located in Jinan City, China, renowned for its spatial–temporal heterogeneity in karst development. Through the analysis and validation conducted by integrating geographical multi‐feature, the study revealed a significant improvement in the accuracy of the RR‐LSTM model after integrating RR as a variable, particularly during significant rainfall events. These findings affirm that the method proposed in this study is highly effective in karst regions characterized by anisotropic karst features.
更多查看译文
AI 理解论文
溯源树
样例
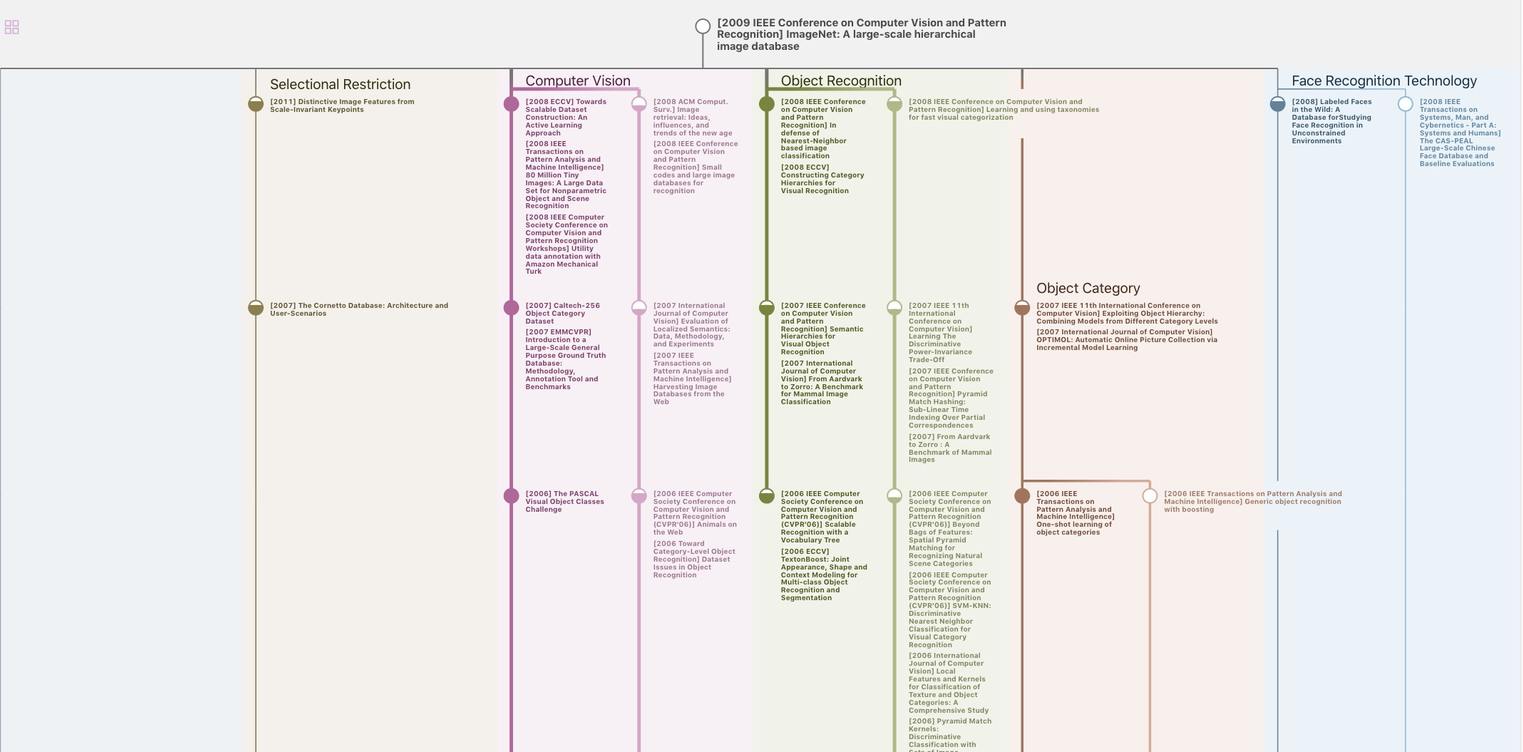
生成溯源树,研究论文发展脉络
Chat Paper
正在生成论文摘要