Predicting the PSQA results of volumetric modulated arc therapy based on dosiomics features: a multi-center study
Frontiers in Physics(2024)
摘要
Backgroud and objectivesThe implementation of patient-specific quality assurance (PSQA) has become a crucial aspect of the radiation therapy process. Machine learning models have demonstrated their potential as virtual QA tools, accurately predicting the gamma passing rate (GPR) of volumetric modulated arc therapy (VMAT)plans, thereby ensuring safe and efficient treatment for patients. However, there is limited multi-center research dedicated to predicting the GPR. In this study, a dosiomics-based machine learning approach was employed to construct a prediction model for classifying GPR in multiple radiotherapy institutions. Additionally, the model’s performance was compared by evaluating the impact of two distinct feature selection methods.MethodsA retrospective data collection was conducted on 572 VMAT patients across three radiotherapy institutions. Utilizing a three-dimensional dose verification technique grounded in real-time measurements, γ analysis was conducted according to the criteria of 3%/2 mm and 2%/2 mm, employing a dose threshold of 10% along with absolute dose and global normalization mode. Dosiomics features were extracted from the dose files, and distinct subsets of features were selected as inputs for the model using the random forest (RF) and RF combined with SHapley Additive exPlanations (SHAP) methods. The data underwent training using the extreme gradient boosting (XGBoost) algorithm, and the model’s classification performance was assessed through F1-score and area under the curve (AUC) values.ResultsThe model exhibited optimal performance under the 3%/2 mm criteria, utilizing a subset of 20 features and attaining an AUC value of 0.88 and an F1-score of 0.89. Similarly, under the 2%/2 mm criteria, the model demonstrated superior performance with a subset of 10 features, resulting in an AUC value of 0.91 and an F1-score of 0.89. The feature selection methods of RF and RF + SHAP have achieved good model performance by selecting as few features as possible.ConclusionBased on the multi-center PSQA results, it is possible to utilize dosiomics features extracted from dose files to construct a machine learning predictive model. This model demonstrates excellent discriminative abilities, thus promoting the progress of gamma passing rate prognostic models in clinical application and implementation. Furthermore, it holds potential in providing patients with secure and efficient personalized QA management, while also reducing the workload of medical physicists.
更多查看译文
关键词
machine learning,volumetric modulated arc therapy,dosiomics,gamma passing rate,multi-center study
AI 理解论文
溯源树
样例
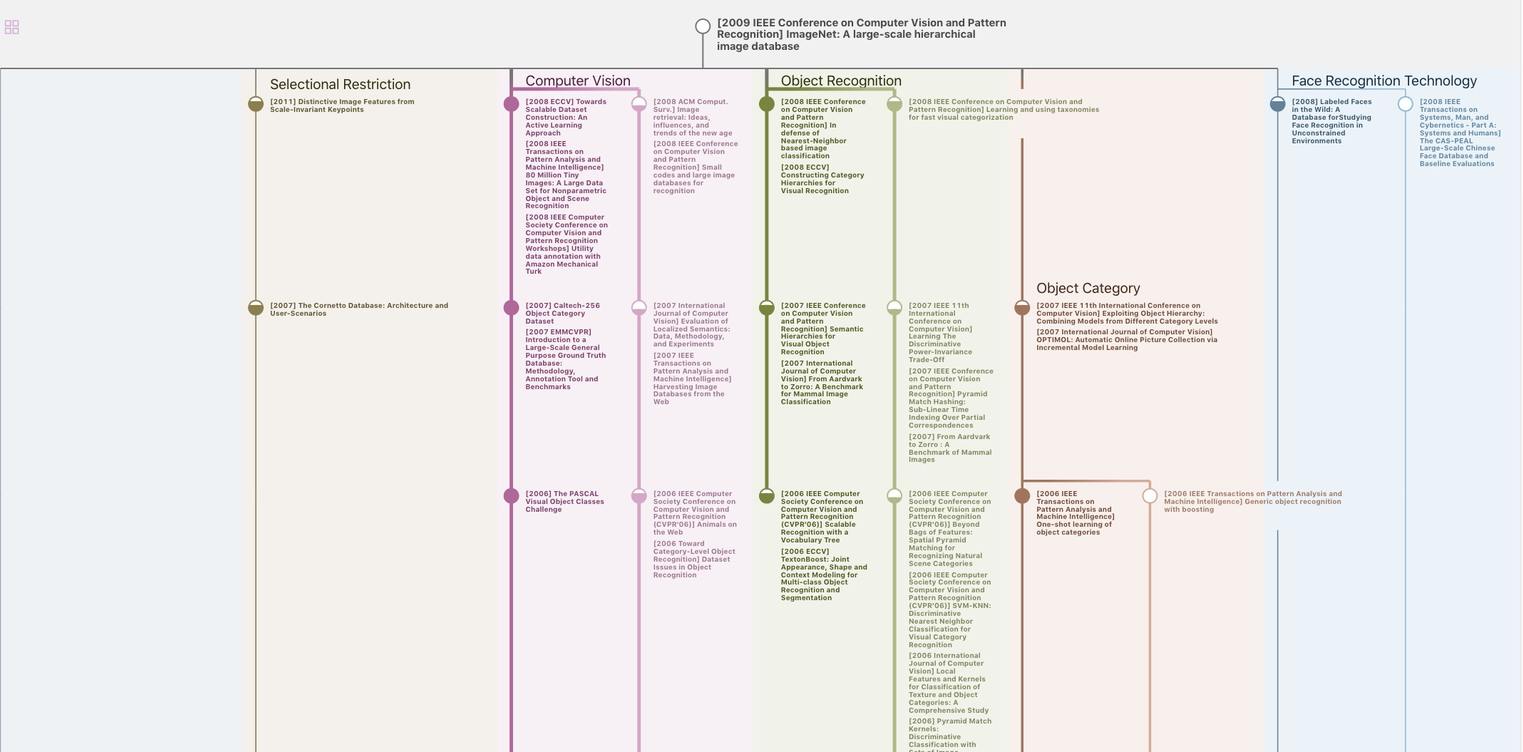
生成溯源树,研究论文发展脉络
Chat Paper
正在生成论文摘要