AWDepth: Monocular Depth Estimation for Adverse Weather via Masked Encoding
IEEE Transactions on Industrial Informatics(2024)
摘要
Monocular depth estimation has made considerable advances under clear weather conditions. However, how to learn accurate scene depth under rain and fog conditions and alleviate the negative influence of occlusion, light, visibility, etc., is an open problem. To address this problem, in this article, we split the adverse weather depth estimation network into two subbranches: the depth prediction branch and the masked encoding branch. The depth prediction branch is used for depth estimation. The masked encoding branch, inspired by masked image modeling, uses random masks to simulate occlusion or low visibility often seen in rain and fog, forcing this branch to learn to infer the prediction of masked regions from the context. In order to make the masked encoding better enhance the depth prediction, we designed the mask feature fusion module, which can fuse the depth and spatial context features of the two branches to produce a fine-level depth map. The experimental results on the Foggy Cityscapes and RainCityscapes datasets demonstrate that our method achieves state-of-the-art performance, significantly outperforming previous methods across all evaluation metrics.
更多查看译文
关键词
Depth estimation,masked image modeling,swin transformer
AI 理解论文
溯源树
样例
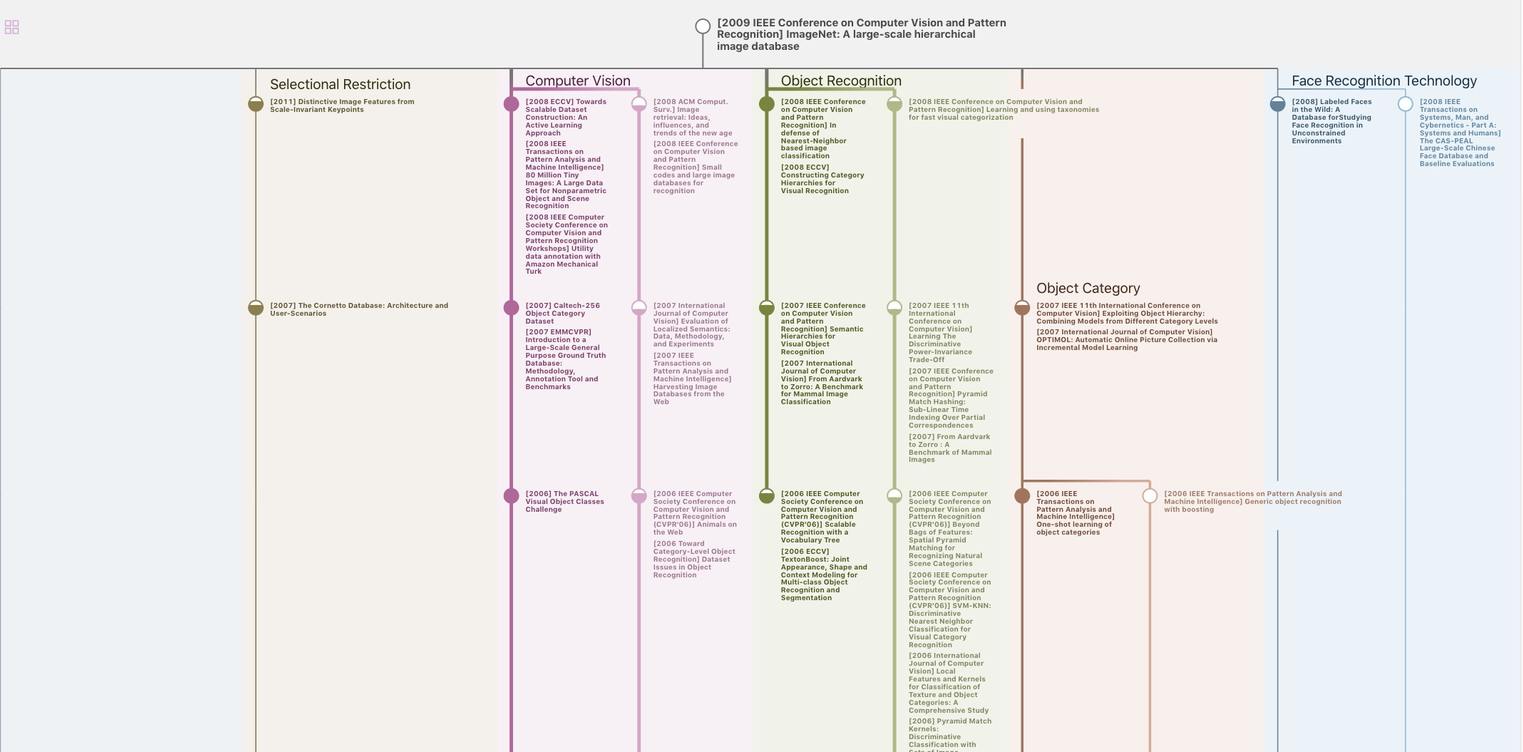
生成溯源树,研究论文发展脉络
Chat Paper
正在生成论文摘要