A global and joint knowledge distillation method with gradient-modulated dynamic parameter adaption for EMU bogie bearing fault diagnosis
Measurement(2024)
摘要
Deep learning has exhibited remarkable performance and achieved significant breakthroughs in railway transportation equipment fault diagnosis. However, in engineering practice, the escalating complexity of model computations poses a challenge, necessitating the compression and acceleration of models as a crucial technology for deploying intelligent algorithms on edge devices that have limited power and memory capabilities. Additionally, the disparity in the distributions of design and operational data poses another obstacle to accurate diagnosis. Despite various training strategies proposed, they often prioritize either network acceleration and quantification or domain adaptation, which may compromise accuracy and efficiency in real-world applications involving both domain-shifted data and resource-constrained environments.To address these challenges, we introduce a global and joint knowledge distillation approach that incorporates gradient-modulated dynamic parameter adaption specifically for bogie bearing fault diagnosis. By distilling the global and joint knowledge from sophisticated neural networks, the resulting compact models not only maintain diagnosis accuracy but also require fewer computational resources for inference. Furthermore, the gradient-modulated dynamic parameter updating strategy ensures stability during the iterative training of both the teacher and student networks. Extensive experiments conducted on two benchmark datasets demonstrate that the proposed algorithm surpasses pure distillation methods, highlighting its effectiveness and efficiency in railway transportation equipment fault diagnosis.
更多查看译文
关键词
Fault diagnosis,Deep learning,Network acceleration,Knowledge distillation
AI 理解论文
溯源树
样例
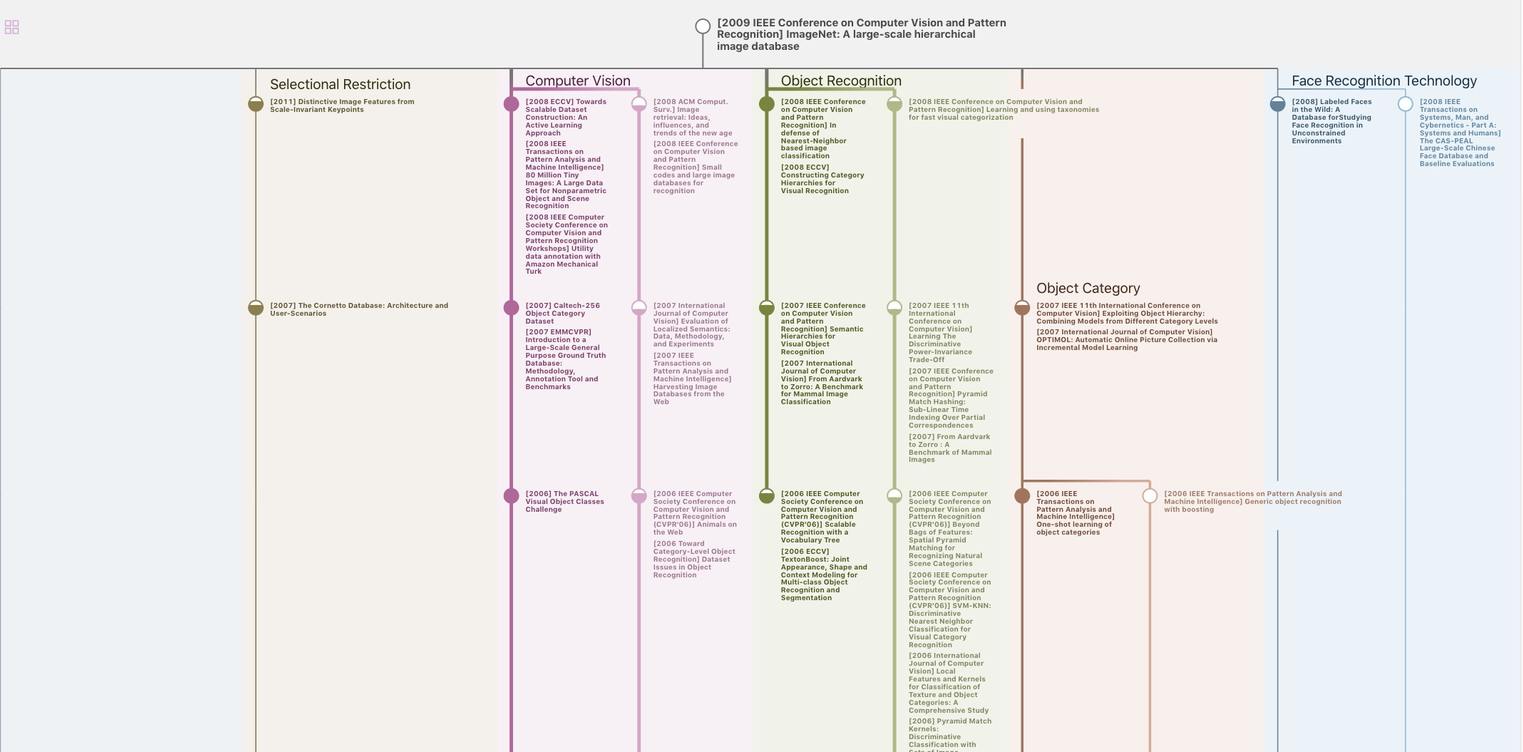
生成溯源树,研究论文发展脉络
Chat Paper
正在生成论文摘要