A novel identification model for rock burst grades-taking Jinping II hydropower station hub engineering as an example
Computers and Geotechnics(2024)
摘要
In order to accurately assess the degree of rock burst hazards in actual engineering, the relevance vector machine (RVM) model based on principal component analysis (PCA) and particle swarm optimization (PSO) is introduced to identify the grades of rock burst. Compared with current machine learning methods for rock burst identification, the presented PCA-PSO-RVM model effectively makes up for the disadvantages of low accuracy, complex calculation, and parameter adjustment limitations, where PCA indirectly improves identification accuracy by the dimensionality reduction, PSO implements the automatic optimization of kernel function parameters, and Gaussian kernel function in RVM greatly reduces the computational complexity of nonlinear mapping. The samples of rock burst are the field data from the 3# diversion tunnel of Jinping II hydropower station, and the grades of rock burst are identified by RVM with the cross-validation method. The identification results indicate that the average accuracy of four segments reaches 95.46%, which proves the high accuracy and strong adaptability of the presented PCA-PSO-RVM model.
更多查看译文
关键词
Rock burst grade,Principal component analysis,Particle swarm optimization,Relevance vector machine
AI 理解论文
溯源树
样例
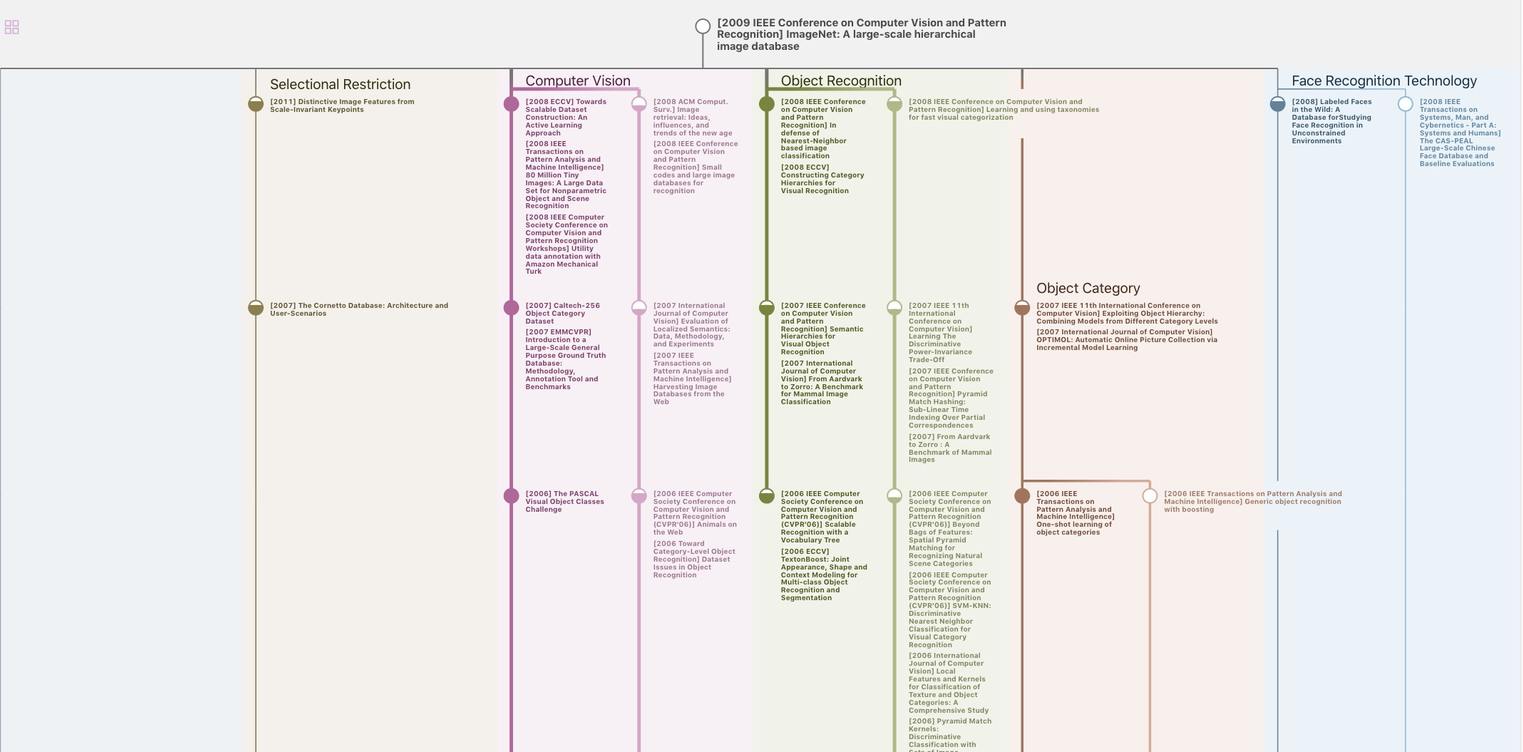
生成溯源树,研究论文发展脉络
Chat Paper
正在生成论文摘要