Invariant Content Representation for Generalizable Medical Image Segmentation
Journal of Imaging Informatics in Medicine(2024)
摘要
Domain generalization (DG) for medical image segmentation due to privacy preservation prefers learning from a single-source domain and expects good robustness on unseen target domains. To achieve this goal, previous methods mainly use data augmentation to expand the distribution of samples and learn invariant content from them. However, most of these methods commonly perform global augmentation, leading to limited augmented sample diversity. In addition, the style of the augmented image is more scattered than the source domain, which may cause the model to overfit the style of the source domain. To address the above issues, we propose an invariant content representation network (ICRN) to enhance the learning of invariant content and suppress the learning of variability styles. Specifically, we first design a gamma correction-based local style augmentation (LSA) to expand the distribution of samples by augmenting foreground and background styles, respectively. Then, based on the augmented samples, we introduce invariant content learning (ICL) to learn generalizable invariant content from both augmented and source-domain samples. Finally, we design domain-specific batch normalization (DSBN) based style adversarial learning (SAL) to suppress the learning of preferences for source-domain styles. Experimental results show that our proposed method improves by 8.74 https://github.com/ZMC-IIIM/ICRN-DG .
更多查看译文
关键词
Domain generalization,Medical image segmentation,Data augmentation,Invariant content mining
AI 理解论文
溯源树
样例
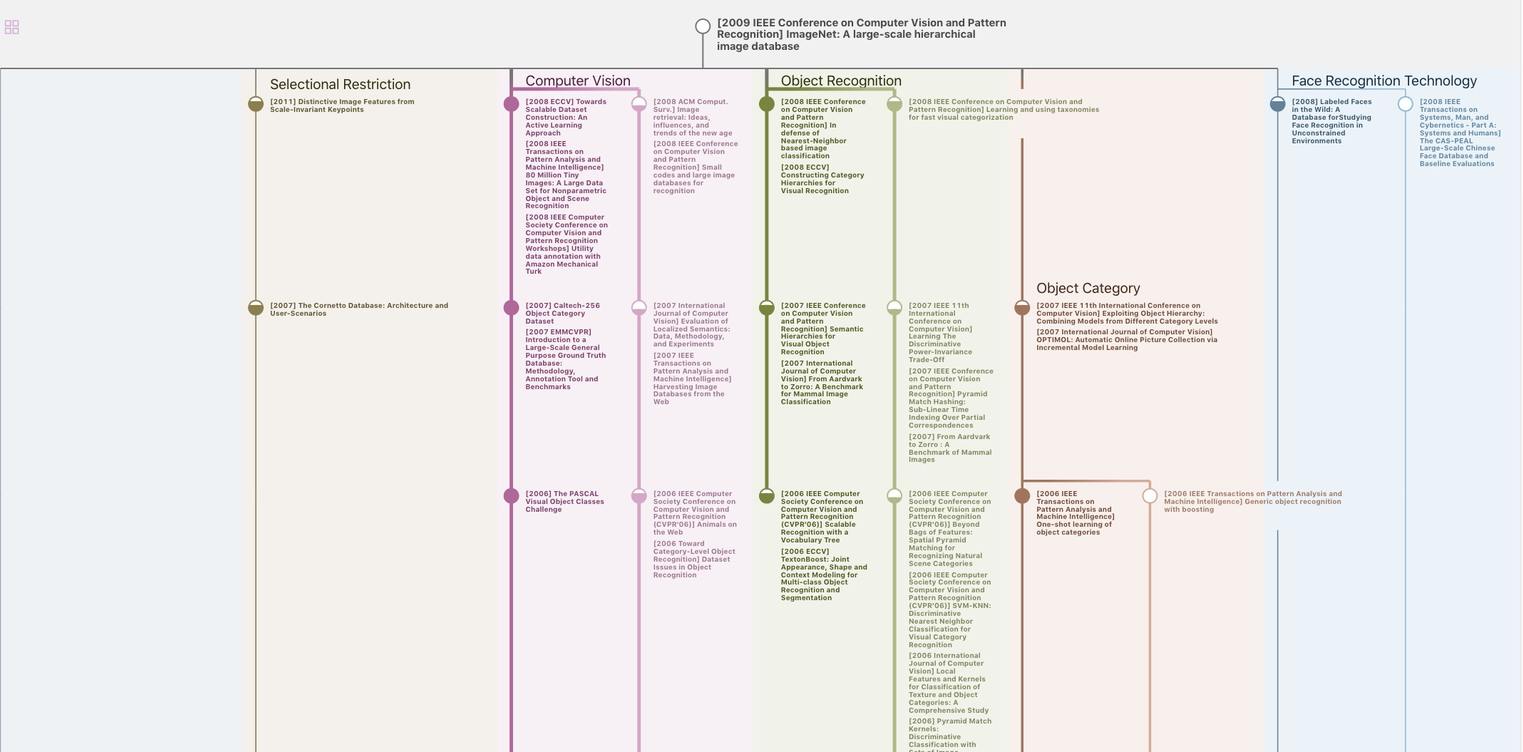
生成溯源树,研究论文发展脉络
Chat Paper
正在生成论文摘要