Memory-Bound and Taxonomy-Aware K-Mer Selection for Ultra-Large Reference Libraries.
Annual International Conference on Research in Computational Molecular Biology(2024)
Abstract
Classifying sequencing reads based on k -mer matches to a reference library is widely used in applications such as taxonomic profiling. Given the ever-increasing number of genomes publicly available, it is increasingly impossible to keep all or a majority of their k -mers in memory. Thus, there is a growing need for methods for selecting a subset of k -mers while accounting for taxonomic relationships. We propose k -mer RANKer (KRANK), a method that uses a set of heuristics to efficiently and effectively select a size-constrained subset of k -mers from a diverse and imbalanced taxonomy that suffers biased sampling. Empirical evaluations demonstrate that a fraction of all k -mers in large reference libraries can achieve comparable accuracy to the full set.
MoreTranslated text
AI Read Science
Must-Reading Tree
Example
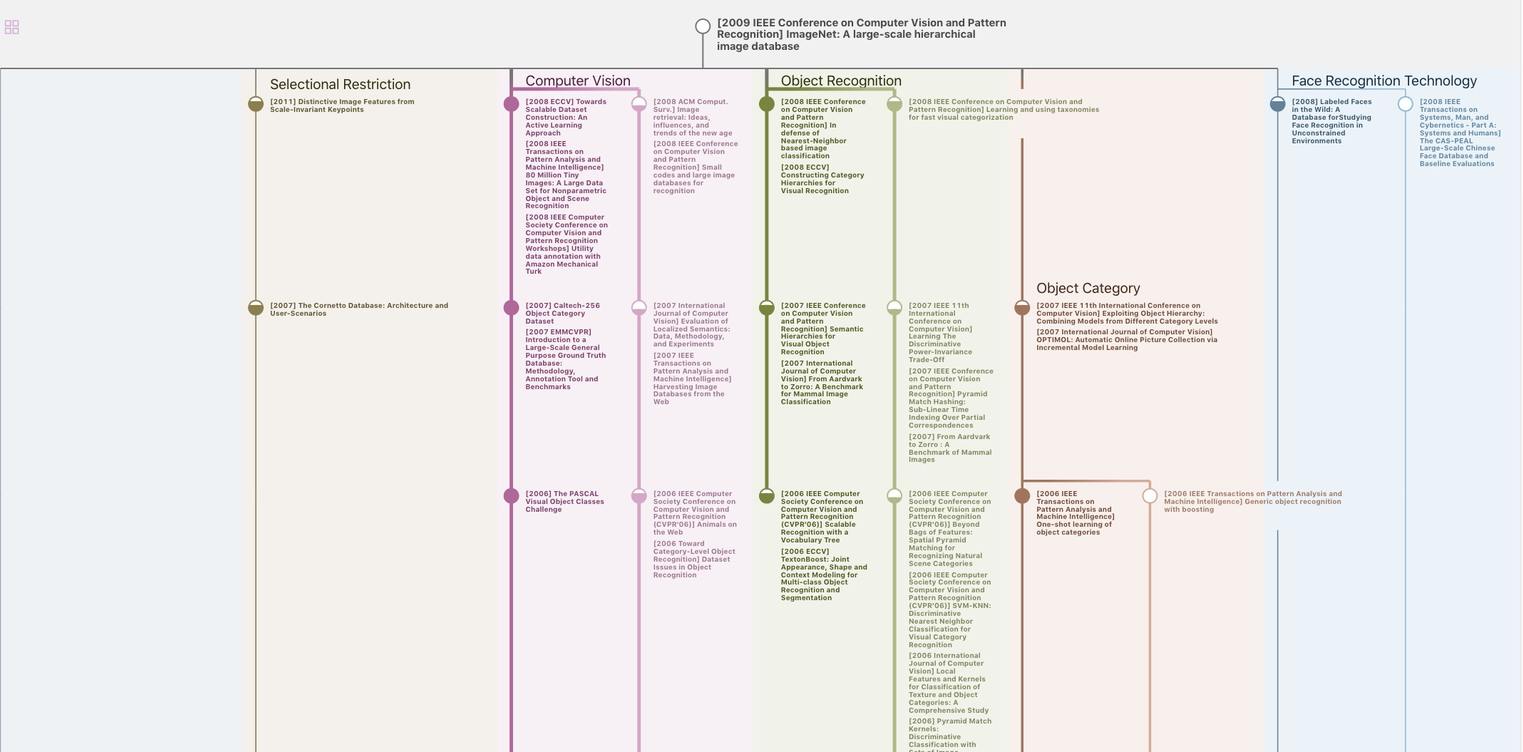
Generate MRT to find the research sequence of this paper
Chat Paper
Summary is being generated by the instructions you defined