TEFLON: Thermally Efficient Dataflow-Aware 3D NoC for Accelerating CNN Inferencing on Manycore PIM Architectures
ACM Transactions on Embedded Computing Systems(2024)
摘要
Resistive random-access memory (ReRAM) based processing-in-memory (PIM) architectures are used extensively to accelerate inferencing/training with convolutional neural networks (CNNs). Three-dimensional (3D) integration is an enabling technology to integrate many PIM cores on a single chip. In this work, we propose the design of a thermally efficient dataflow-aware monolithic 3D (M3D) NoC architecture referred to as TEFLON to accelerate CNN inferencing without creating any thermal bottlenecks. TEFLON reduces the Energy-Delay-Product (EDP) by 4 2\% , 46\% , and 45 \% on an average compared to a conventional 3D mesh NoC for systems with 36-, 64-, and 100-PIM cores respectively. TEFLON reduces the peak chip temperature by 25 K and improves the inference accuracy by up to 11 \% compared to sole performance-optimized SFC-based counterpart for inferencing with diverse deep CNN models using CIFAR-10/100 datasets on a 3D system with 100-PIM cores.
更多查看译文
AI 理解论文
溯源树
样例
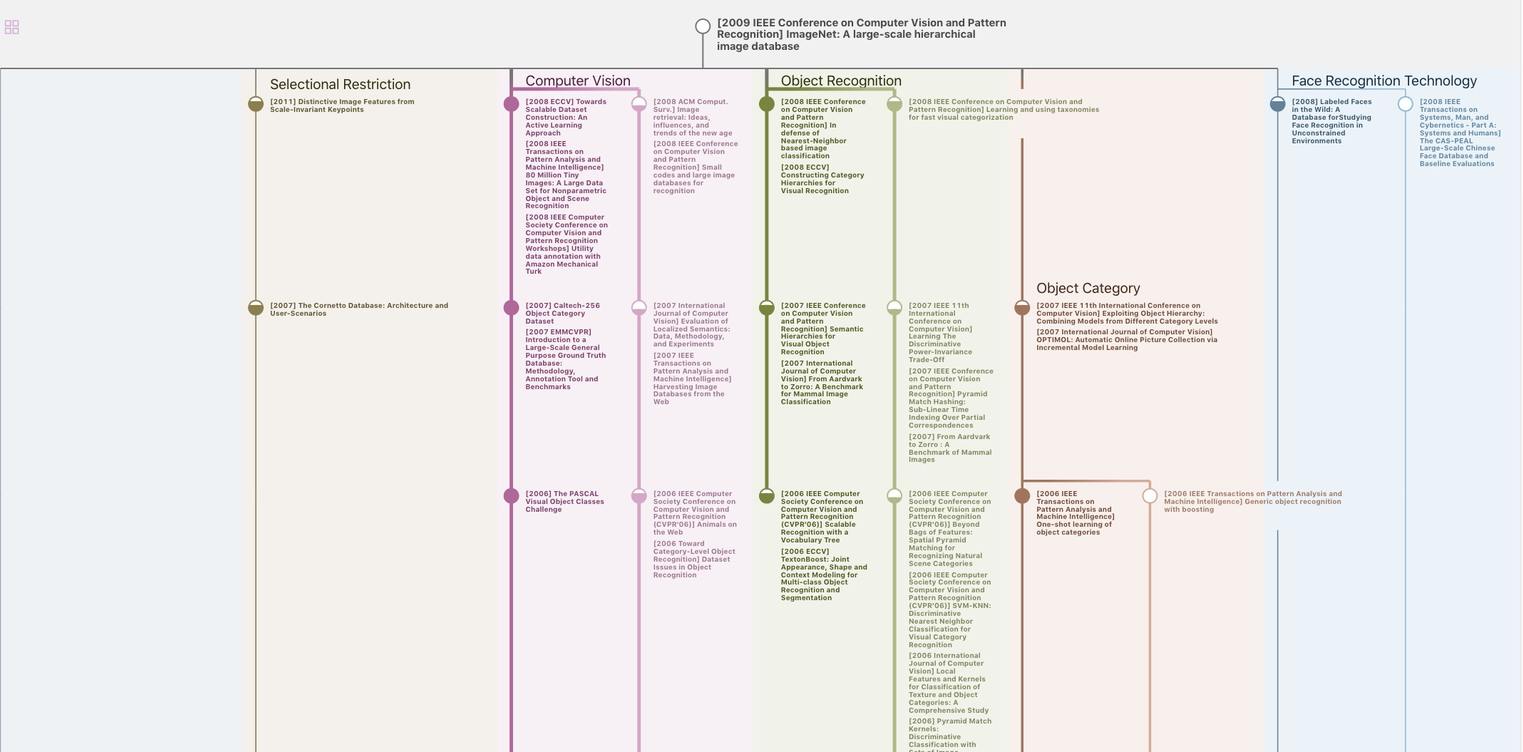
生成溯源树,研究论文发展脉络
Chat Paper
正在生成论文摘要