DeepVID v2: Self-Supervised Denoising with Decoupled Spatiotemporal Enhancement for Low-Photon Voltage Imaging.
bioRxiv : the preprint server for biology(2024)
摘要
SIGNIFICANCE:Voltage imaging is a powerful tool for studying the dynamics of neuronal activities in the brain. However, voltage imaging data are fundamentally corrupted by severe Poisson noise in the low-photon regime, which hinders the accurate extraction of neuronal activities. Self-supervised deep learning denoising methods have shown great potential in addressing the challenges in low-photon voltage imaging without the need for ground truth, but usually suffer from the tradeoff between spatial and temporal performance.
AIM:We present DeepVID v2, a novel self-supervised denoising framework with decoupled spatial and temporal enhancement capability to significantly augment low-photon voltage imaging.
APPROACH:DeepVID v2 is built on our original DeepVID framework, which performs frame-based denoising by utilizing a sequence of frames around the central frame targeted for denoising to leverage temporal information and ensure consistency. The network further integrates multiple blind pixels in the central frame to enrich the learning of local spatial information. Additionally, DeepVID v2 introduces a new edge extraction branch to capture fine structural details in order to learn high spatial resolution information.
RESULTS:We demonstrate that DeepVID v2 is able to overcome the tradeoff between spatial and temporal performance, and achieve superior denoising capability in resolving both high-resolution spatial structures and rapid temporal neuronal activities. We further show that DeepVID v2 is able to generalize to different imaging conditions, including time-series measurements with various signal-to-noise ratios (SNRs) and in extreme low-photon conditions.
CONCLUSIONS:Our results underscore DeepVID v2 as a promising tool for enhancing voltage imaging. This framework has the potential to generalize to other low-photon imaging modalities and greatly facilitate the study of neuronal activities in the brain.
更多查看译文
AI 理解论文
溯源树
样例
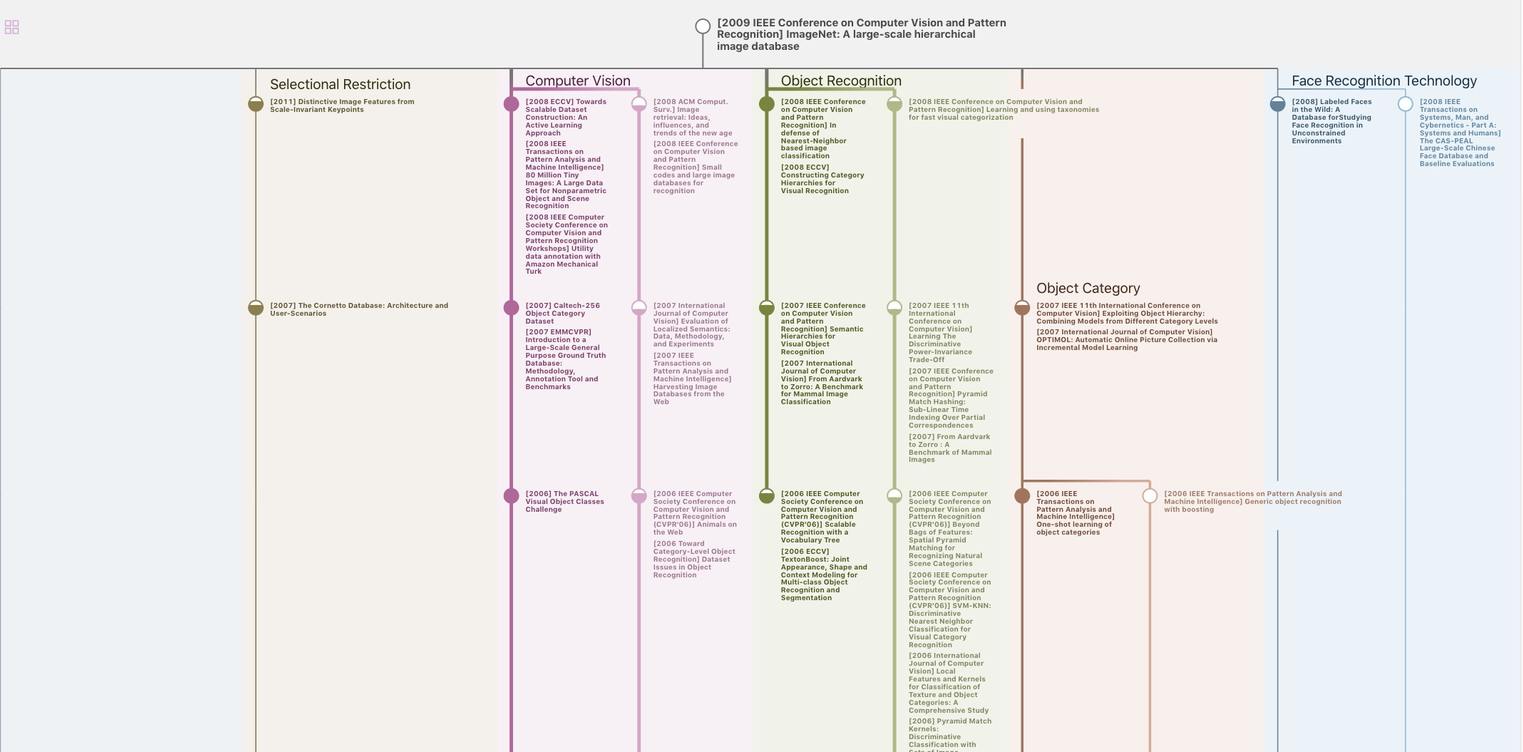
生成溯源树,研究论文发展脉络
Chat Paper
正在生成论文摘要