Development and Preliminary Assessment of a Machine Learning Model to Predict Myocardial Infarction and Cardiac Arrest After Major Operations
Resuscitation(2024)
摘要
Introduction
Accurate prediction of complications often informs shared decision-making. Derived over 10 years ago to enhance prediction of intra/post-operative myocardial infarction and cardiac arrest (MI/CA), the Gupta score has been criticized for unreliable calibration and inclusion of a wide spectrum of unrelated operations. In the present study, we developed a novel machine learning (ML) model to estimate perioperative risk of MI/CA and compared it to the Gupta score.
Methods
Patients undergoing major operations were identified from the 2016-2020 ACS-NSQIP. The Gupta score was calculated for each patient, and a novel ML model was developed to predict MI/CA using ACS NSQIP-provided data fields as covariates. Discrimination (C-statistic) and calibration (Brier score) of the ML model were compared to the existing Gupta score within the entire cohort and across operative subgroups.
Results
Of 2,473,487 patients included for analysis, 25,177 (1.0%) experienced MI/CA (55.2% MI, 39.1% CA, 5.6% MI and CA). The ML model, which was fit using a randomly selected training cohort, exhibited higher discrimination within the testing dataset compared to the Gupta score (C-statistic 0.84 vs 0.80, p<0.001). Furthermore, the ML model had significantly better calibration in the entire cohort (Brier score 0.0097 vs 0.0100). Model performance was markedly improved among patients undergoing thoracic, aortic, peripheral vascular and foregut surgery.
Conclusions
The present ML model outperformed the Gupta score in the prognostication of MI/CA across a heterogenous range of operations. Given the growing integration of ML into healthcare, such models may be readily incorporated into clinical practice and guide benchmarking efforts.
更多查看译文
关键词
cardiac arrest,machine learning,NSQIP,Gupta score,myocardial infarction
AI 理解论文
溯源树
样例
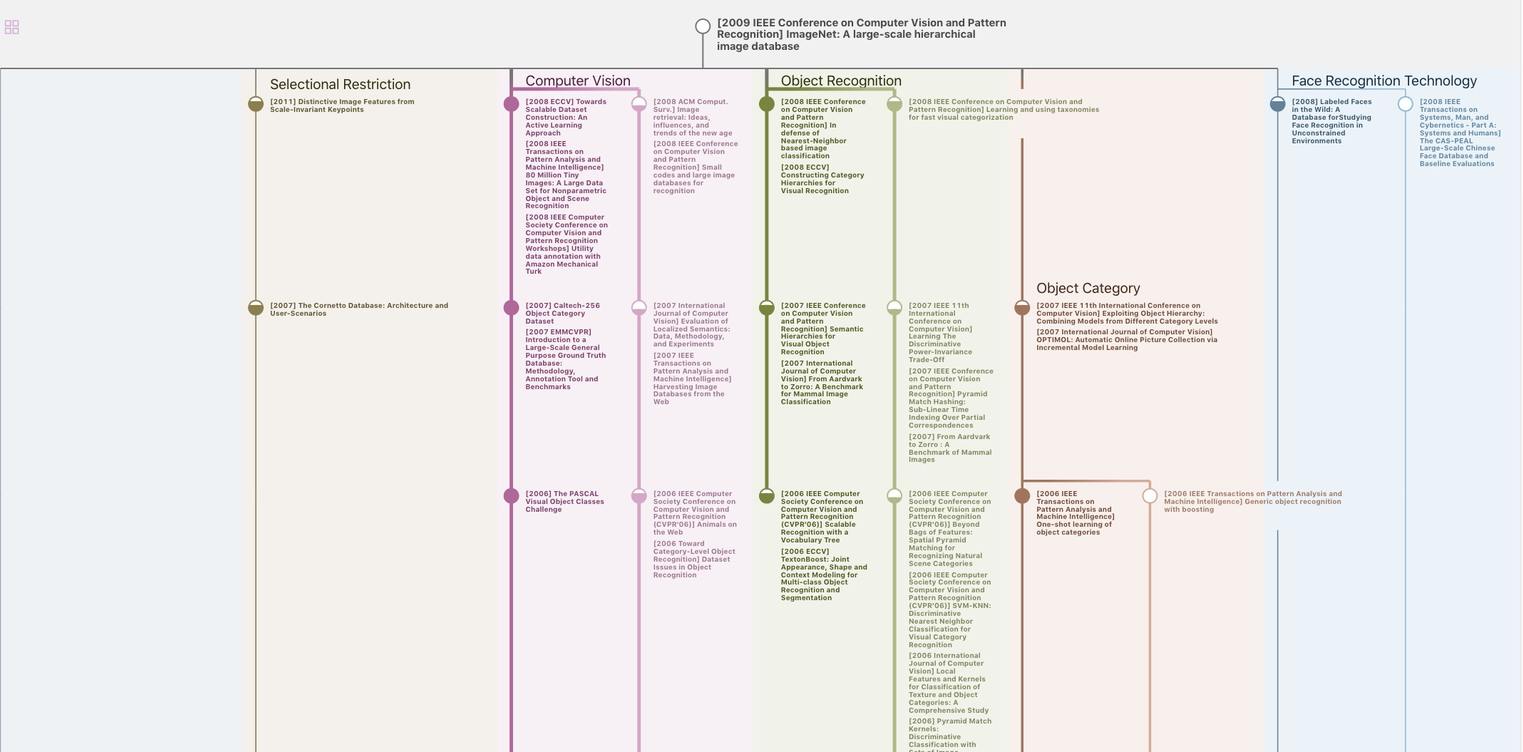
生成溯源树,研究论文发展脉络
Chat Paper
正在生成论文摘要