Safety reinforcement learning control via transfer learning
Automatica(2024)
摘要
Reinforcement learning (RL) has emerged as a promising approach for modern control systems. However, its success in real-world applications has been limited due to the lack of safety guarantees. To address this issue, the authors present a novel transfer learning framework that facilitates policy training in a non-dangerous environment, followed by transfer of the trained policy to the original dangerous environment. The transferred policy is theoretically proven to stabilize the original system while maintaining safety. Additionally, we propose an uncertainty learning algorithm incorporated in RL that overcomes natural data cascading and data evolution problems in RL to enhance learning accuracy. The transfer learning framework avoids trial-and-error in unsafe environments, ensuring not only after-learning safety but, more importantly, addressing the challenging problem of safe exploration during learning. Simulation results demonstrate the promise of the transfer learning framework for RL safety control on the task of vehicle lateral stability control with safety constraints.
更多查看译文
关键词
Reinforcement learning control,Safety,Stability,Transfer learning
AI 理解论文
溯源树
样例
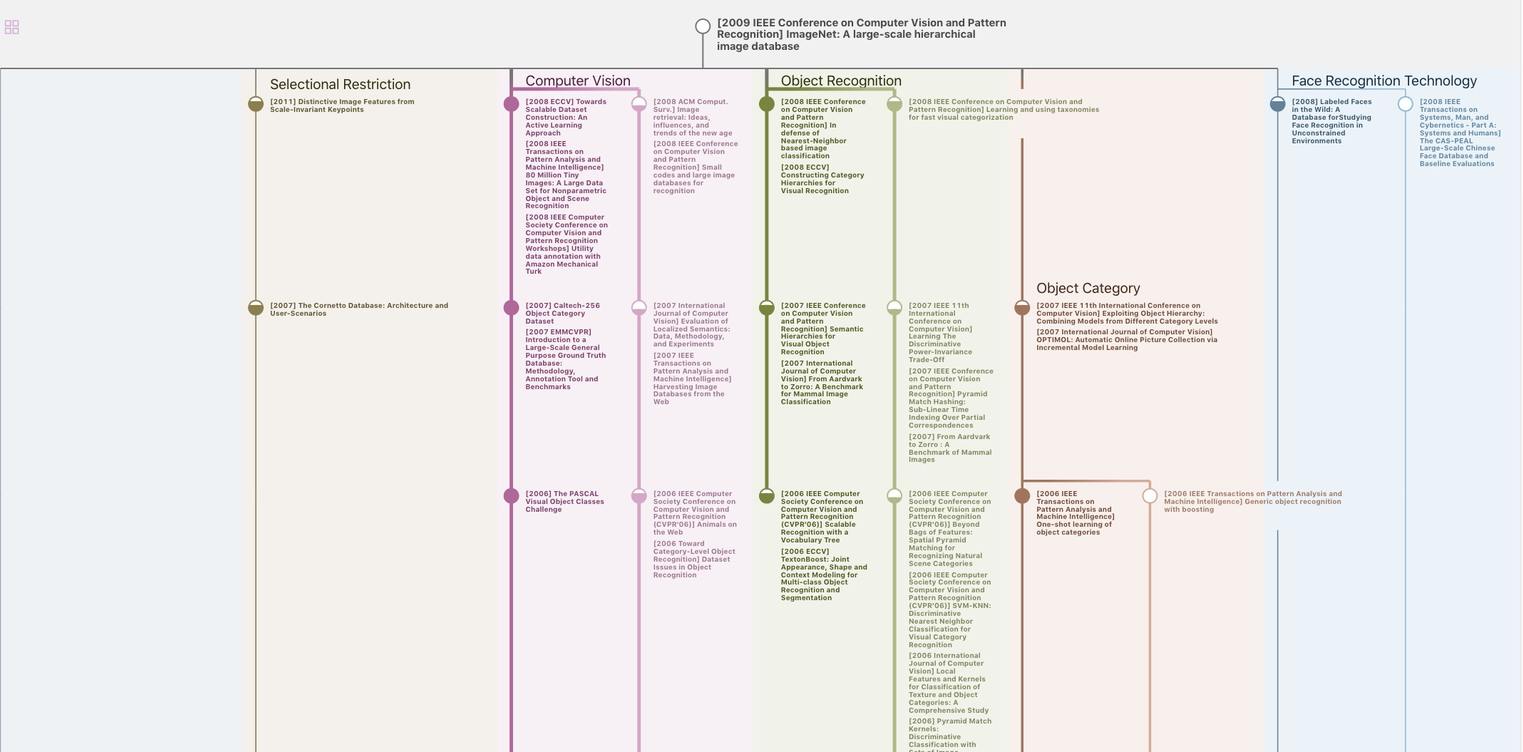
生成溯源树,研究论文发展脉络
Chat Paper
正在生成论文摘要