A novel residual hybrid dynamic model for unmanned helicopters: combining both physical and deep learning models
Nonlinear Dynamics(2024)
摘要
Unmanned helicopters are unstable, high-order nonlinear systems. It is difficult to model the system dynamics accurately by physical models due to simplifications and assumptions. Deep learning based modeling methods could achieve high prediction accuracy without any simplifications and assumptions, while their results are hard to interpret. To ensure both accuracy and interpretability, a novel residual hybrid dynamic model is proposed, which combines a physical model, describing the essential dynamic characteristics of unmanned helicopters, and a convolutional neural network and long short-term memory neural network (CNN-LSTM) based deep learning model, compensating the errors of the physical model by the residual connection. Meanwhile, the CNN-LSTM model could efficiently learn the information in both state-control and time dimensions due to the well-designed network structure. Moreover, a two-step optimization method is proposed to optimize parameters of the residual hybrid dynamic model on the flight data from the Stanford Autonomous Helicopter Project. The experiment results demonstrate that the proposed residual hybrid dynamic model exhibits superior accuracy, efficiency, and generalization capabilities compared to the baseline models.
更多查看译文
关键词
Unmanned helicopter,Dynamic model,Deep learning,Hybrid model
AI 理解论文
溯源树
样例
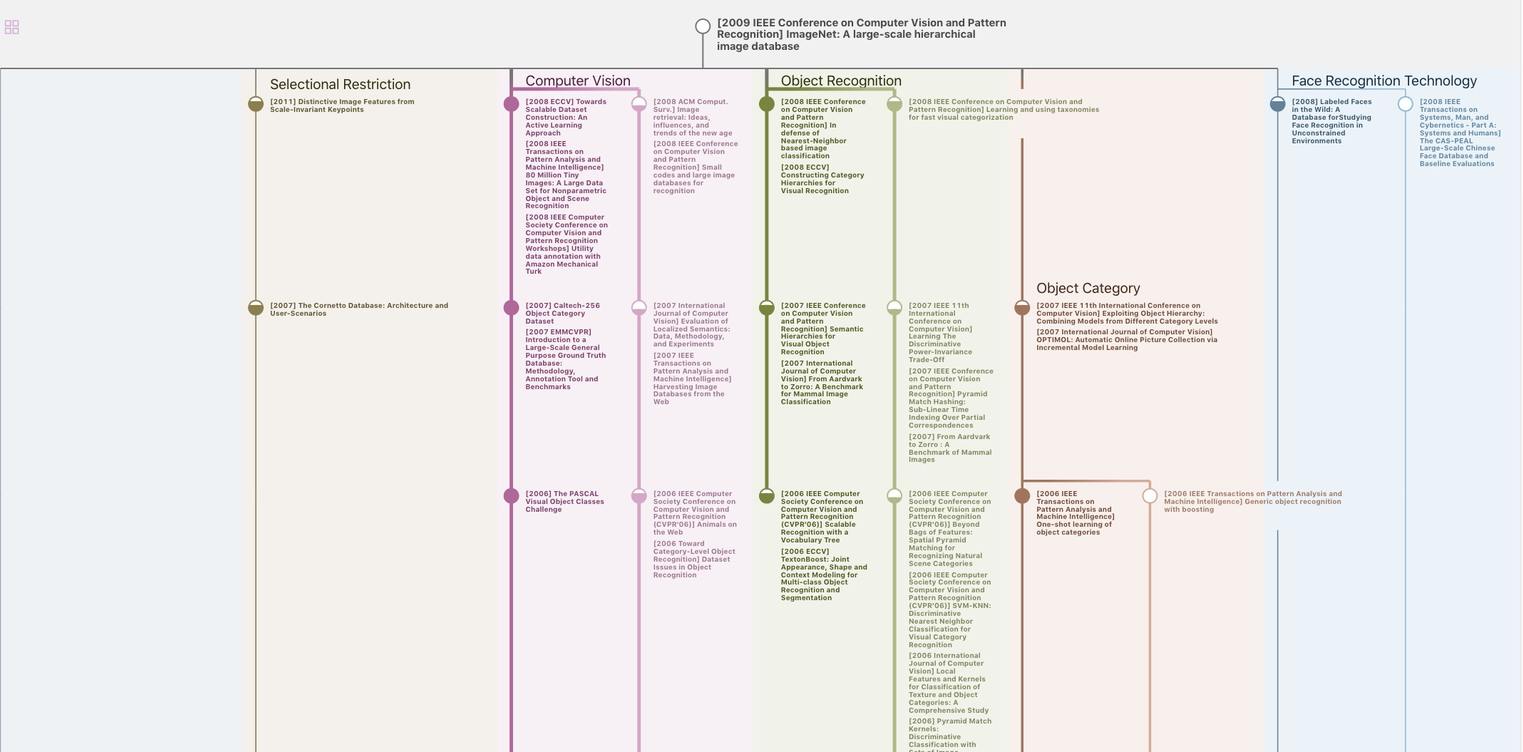
生成溯源树,研究论文发展脉络
Chat Paper
正在生成论文摘要