Sensor-Managed Anomaly Detection for Camouflage Detection in Airborne Multispectral Imagery
2024 IEEE Aerospace Conference(2024)
摘要
Multispectral sensors provide valuable spectral information suitable for a variety of remote sensing applications, especially when cost-effectiveness, portability, and energy efficiency are essential, such as on small tactical drones. Since all materials have unique spectral signatures, this spectral information can be utilized to detect camouflaged targets in military reconnaissance scenarios. However, varying environmental conditions like vegetation and weather in real-world reconnaissance scenarios, and the computational complexity introduced by the large number of bands, make successful camouflage detections difficult. To address these issues, a novel sensor-managed anomaly detection method is proposed. This approach determines a subset of sensor bands of a multispectral imaging system based on current environmental conditions and their ability to expose camouflaged targets. Instead of processing all available bands, a spectral anomaly detector then processes only this subset of bands. To evaluate the robustness of the proposed approach, four different spectral anomaly detection methods are employed and compared: the RX detector, LRX detector, AED detector, and LPD detector. Experiments on an extensive multi-spectral dataset for camouflage detection show nearly equivalent detection performance when spectral anomaly detectors are applied to the managed subset compared to all sensor bands. This demonstrates the effectiveness of the proposed approach in reducing computational complexity while maintaining detection performance, which is particularly important for small tactical drones in military reconnaissance scenarios.
更多查看译文
关键词
Multispectral Images,Anomaly Detection,Airborne Multispectral Imagery,Computational Complexity,Detection Performance,Detection Dataset,Spectral Detection,Multispectral Sensors,Anomaly Detection Methods,Large Number Of Bands,Multispectral Imaging System,Sensor Band,Training Data,Test Data,Predictive Performance,Higher Threshold,Object Detection,Sensory Function,Intersection Over Union,Area Under Curve,Computer-aided Diagnosis,Target Scene,Artificial Turf,Detection Function,Low False Alarm Rate,Pixel Representation,Part Of Table,Mahalanobis Distance,Binary Threshold
AI 理解论文
溯源树
样例
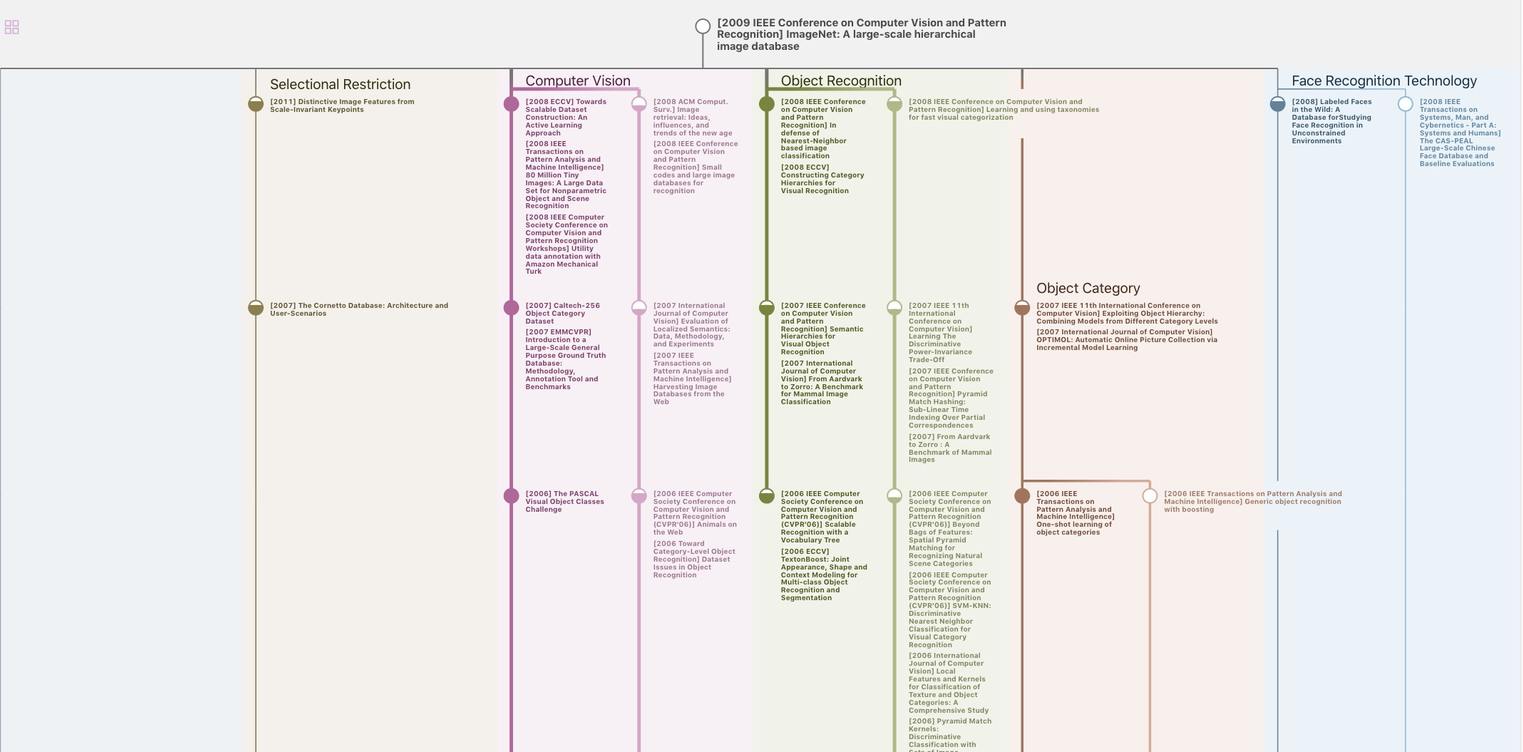
生成溯源树,研究论文发展脉络
Chat Paper
正在生成论文摘要