Establishing a machine learning model based on dual-energy CT enterography to evaluate Crohn’s disease activity
Insights into Imaging(2024)
摘要
The simplified endoscopic score of Crohn’s disease (SES-CD) is the gold standard for quantitatively evaluating Crohn’s disease (CD) activity but is invasive. This study aimed to develop and validate a machine learning (ML) model based on dual-energy CT enterography (DECTE) to noninvasively evaluate CD activity. We evaluated the activity in 202 bowel segments of 46 CD patients according to the SES-CD score and divided the segments randomly into training set and testing set at a ratio of 7:3. Least absolute shrinkage and selection operator (LASSO) was used for feature selection, and three models based on significant parameters were established based on logistic regression. Model performance was evaluated using receiver operating characteristic (ROC), calibration, and clinical decision curves. There were 110 active and 92 inactive bowel segments. In univariate analysis, the slope of spectral curve in the venous phases (λHU-V) has the best diagnostic performance, with an area under the ROC curve (AUC) of 0.81 and an optimal threshold of 1.975. In the testing set, the AUC of the three models established by the 7 variables to differentiate CD activity was 0.81–0.87 (DeLong test p value was 0.071–0.766, p > 0.05), and the combined model had the highest AUC of 0.87 (95
更多查看译文
关键词
Inflammatory bowel disease,Crohn’s disease,Dual energy CT,Machine learning,Activity
AI 理解论文
溯源树
样例
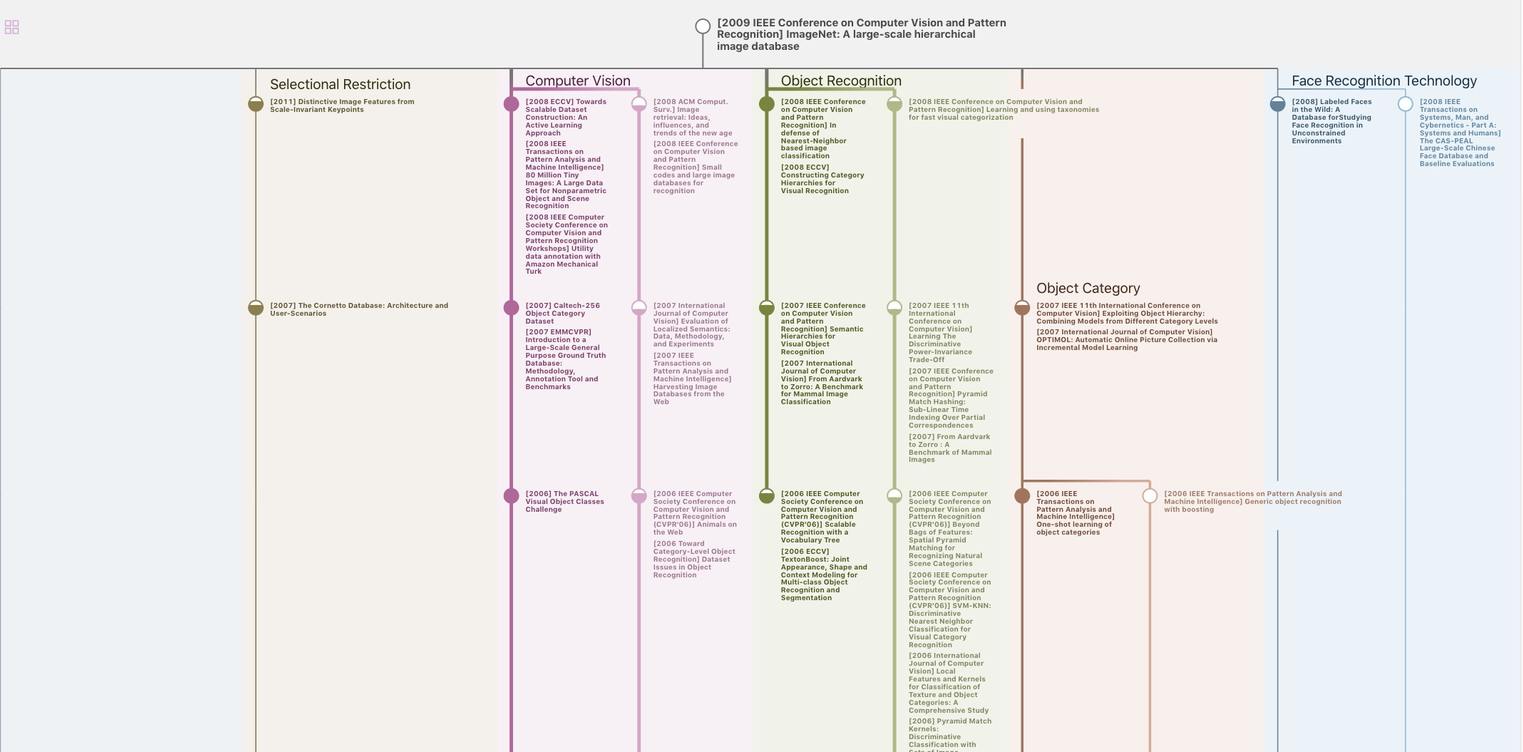
生成溯源树,研究论文发展脉络
Chat Paper
正在生成论文摘要