Reinforcement learning-based order-dispatching optimization in the ride-sourcing service
Computers & Industrial Engineering(2024)
摘要
Since the emergence of ride-sourcing, the dispatching problem has been a hot research issue in the travel market. Previous studies designed various refined algorithms to improve the dispatching efficiency. However, the heterogeneity of passenger cancellation behavior and driver's work patterns is ignored in the modeling of these studies, which may result in the algorithms failing to entirely capture the randomness in the real world, thereby affecting dispatching efficiency. To fill this research gap, this paper employs a discrete choice model and Gaussian mixture model to model passenger cancellation behavior and extract driver working patterns, respectively. Furthermore, a cooperative deep deterministic policy gradient (DDPG) algorithm is proposed for dispatching. The algorithm constructs decision networks separately for drivers with different working patterns to learn the optimal dispatching decision and designs a centralized neural network to coordinate the dispatching of all vehicles to maximize the global benefit. A price incentive strategy is proposed to improve passenger loyalty by providing incentive prices. Moreover, based on the constructed discrete choice model, a simulation environment is designed to describe the cancellation behavior in the real world and support the training of the algorithm. Experimental results based on real data in Beijing show that the cooperative DDPG algorithm can improve the order response rate by 2% to 6% and platform revenue by 3% to 9% in multiple travel periods while providing a better travel experience to passengers. Additionally, the price incentive strategy also effectively reduces passenger cancellation.
更多查看译文
关键词
Ride-sourcing,Order-dispatching,Reinforcement learning,Price incentives,Gaussian mixture model
AI 理解论文
溯源树
样例
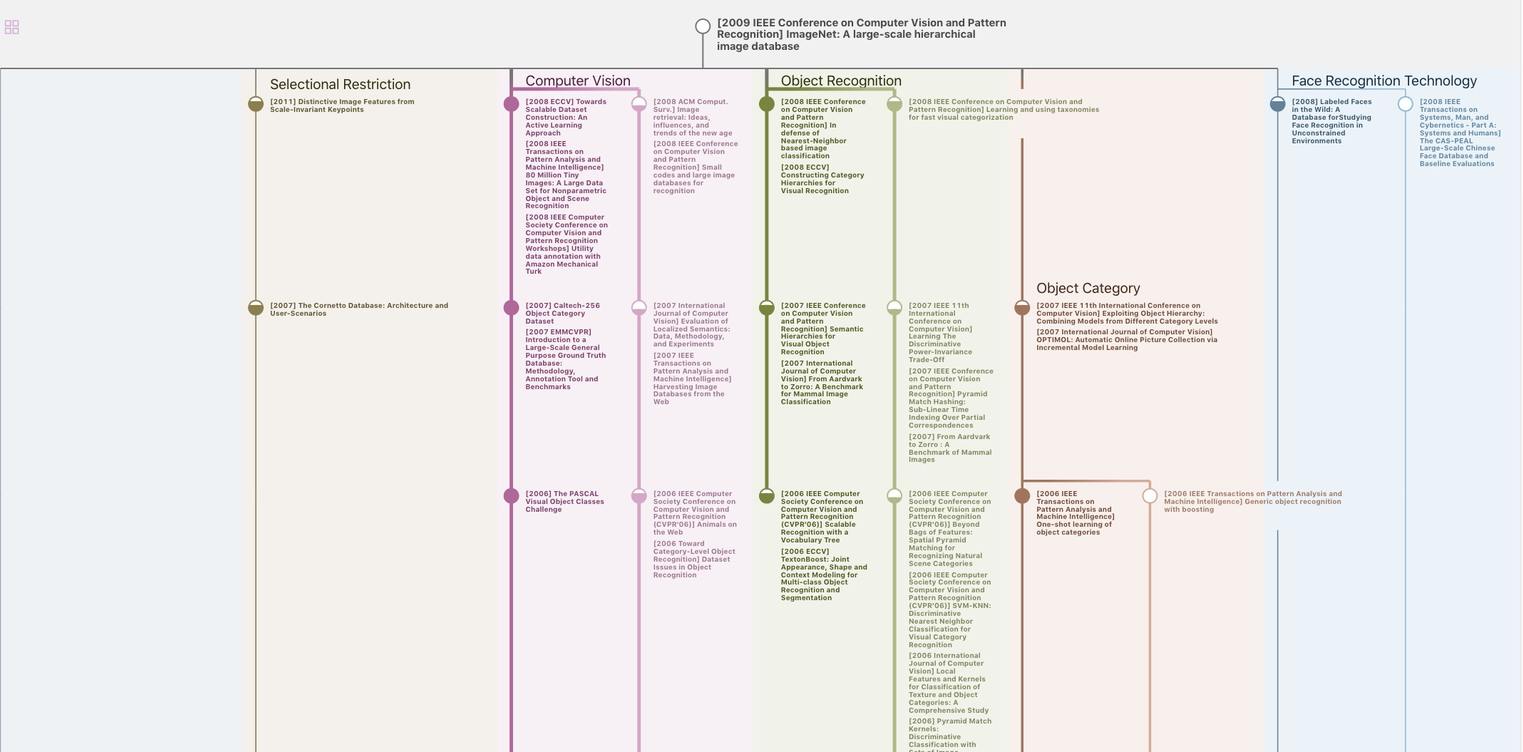
生成溯源树,研究论文发展脉络
Chat Paper
正在生成论文摘要