Turning A Curse into A Blessing: Data-Aware Memory-Efficient Training of Graph Neural Networks by Dynamic Exiting
WWW 2024(2024)
AI Read Science
Must-Reading Tree
Example
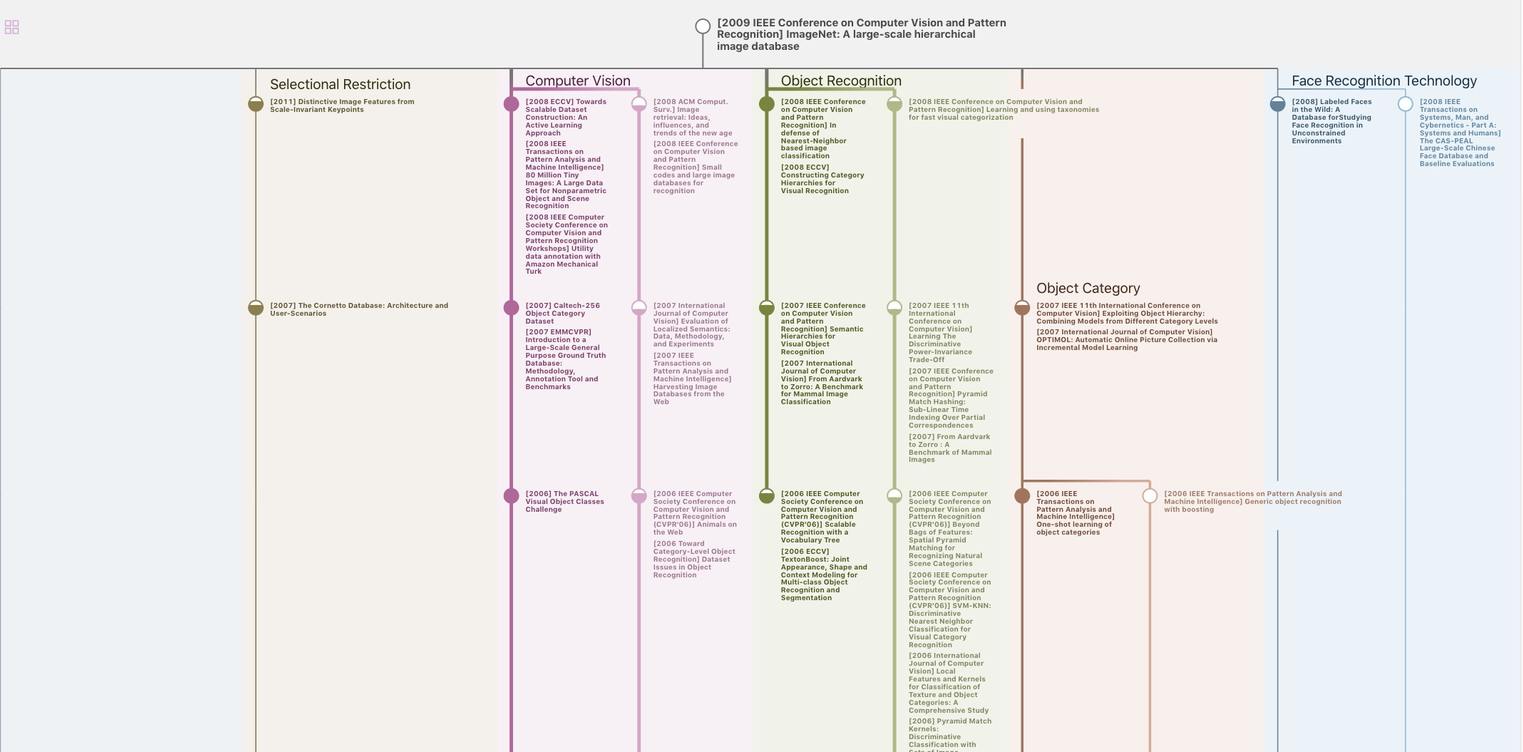
Generate MRT to find the research sequence of this paper
Chat Paper
Summary is being generated by the instructions you defined