Predicting Maximum Pitting Corrosion Depth in Buried Transmission Pipelines: Insights from Tree-Based Machine Learning and Identification of Influential Factors
Process Safety and Environmental Protection(2024)
摘要
Pitting corrosion is a primary type of external corrosion that poses a critical challenge in the oil and gas industry, potentially leading to severe environmental, health, and economic consequences if left unaddressed. Researchers have attempted to comprehend and forecast it through diverse methods, including machine learning and artificial intelligence. However, achieving a precise estimation of pitting growth while considering the influence of various environmental and operational factors remains a concern. This study aims to develop a model by employing tree-based machine learning algorithms, including Decision Tree, Random Forest, LightGBM, CatBoost, and XGBoost, to enhance the prediction accuracy of the maximum depth of pitting corrosion in buried transmission pipelines while also facilitating interpretability. The Random Forest model was found to be the best-performing model with a mean absolute error (MAE) of 0.588. Beyond prediction accuracy, the present analysis contributes practical insights for industry application. First, the model identifies pipes at high risk of severe pitting corrosion under challenging environmental conditions, enabling targeted preventive measures. Secondly, the model's interpretation, utilising game-theoretic Shapely values and permutation importance, informs strategic decisions on the burial of new pipes, ultimately extending their operational lifespan. These applications emphasise the potential of our findings to significantly improve the safety, reliability, and risk mitigation strategies in oil and gas pipeline operations.
更多查看译文
关键词
Buried transmission pipelines,Pitting corrosion,Machine learning,Interpretability,Decision Tree
AI 理解论文
溯源树
样例
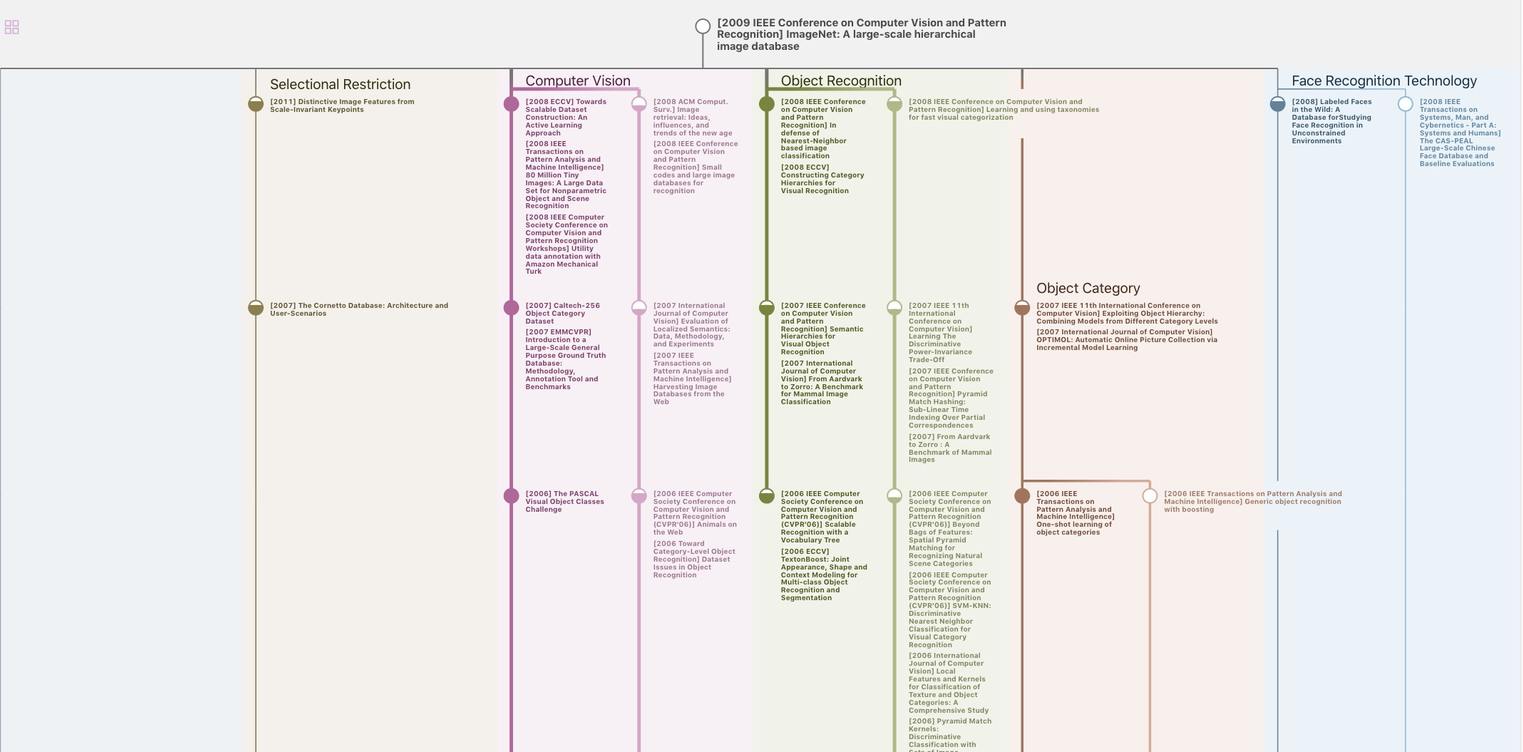
生成溯源树,研究论文发展脉络
Chat Paper
正在生成论文摘要