Adaptive Task-Wise Message Passing for Multi-Task Learning: A Spatial Interaction Perspective
IEEE Transactions on Circuits and Systems for Video Technology(2024)
摘要
Recent advancements have facilitated the simultaneous processing of multiple dense prediction tasks, utilizing diverse correlations between these tasks. However, many of these advances predominantly focus on a singular or fixed task interaction, leading to negative transfer effects. In this paper, we introduce an end-to-end model called the Adaptive Task-Wise Message Passing Network (ATMPNet) for multi-task learning. Our proposed model focuses on excavating comprehensive spatial messages among tasks in an adaptive manner. To achieve this, ATMPNet incorporates the Adaptive Spatial Message Interaction (ASMI) module, which models various local spatial message interactions and global interactions among tasks. ASMI explores potential spatial relationships by generating a task-specific message pool for each target task. Furthermore, we propose an Adaptive Task Message Passing (ATMP) module, a novel method for aggregating messages. The ATMP module generates refined global-local messages from each message pool and adaptively transfers them to the corresponding target tasks through a well-designed message passing scheme. We conduct extensive experiments on the NYUD-v2 and PASCAL-Context datasets to evaluate the effectiveness of ATMPNet. The results demonstrate the state-of-the-art performance of our proposed model in handling multi-task learning scenarios. Code will be publicly available in here.
更多查看译文
关键词
Multi-task learning,Dense prediction,Self-attention mechanism,Graph neural network
AI 理解论文
溯源树
样例
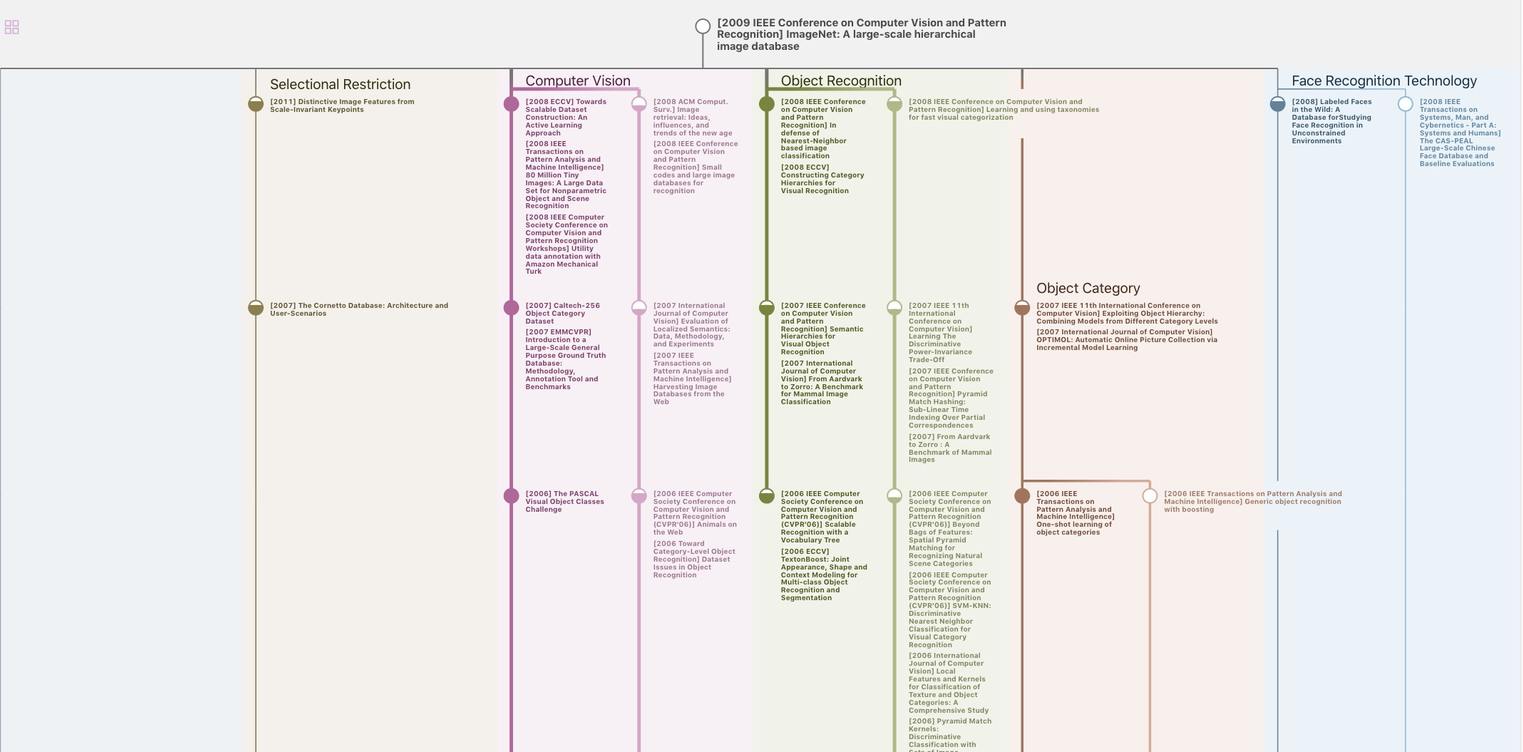
生成溯源树,研究论文发展脉络
Chat Paper
正在生成论文摘要