A Power Data Generation Method Based on CNN-LSTM-GAN for Cable Transmission Line Assessment
2023 IEEE 7th Conference on Energy Internet and Energy System Integration (EI2)(2023)
摘要
At present, the quality of feature data collected through cable transmission lines needs to be improved. There are insufficient credible data samples that can support project review technology analysis and research, which limits the development and application of data-driven smart cable transmission line review technologies. Based on this, this article uses the convo-lutional neural network(CNN), long short-term memory(LSTM) and generative adversarial network(GAN) model to solve the problems of missing power data and insufficient number of samples in the cable transmission line review scenario. First, we use convolutional neural network and long short-term memory to finish the missing power data restoration. Then, based on the completed data set, generative adversarial network is used to generate power data. Finally, the effectiveness of the CNN-LSTM-GAN model was verified by taking the power data in real cable transmission line review as an example. The results show that the data generated by this method has high accuracy, which helps to accurately draw load curves and solve project review difficulties.
更多查看译文
关键词
power data generation,missing power data restoration,convolutional neural network(CNN),long short-term memory(LSTM),generative adversarial network(GAN)
AI 理解论文
溯源树
样例
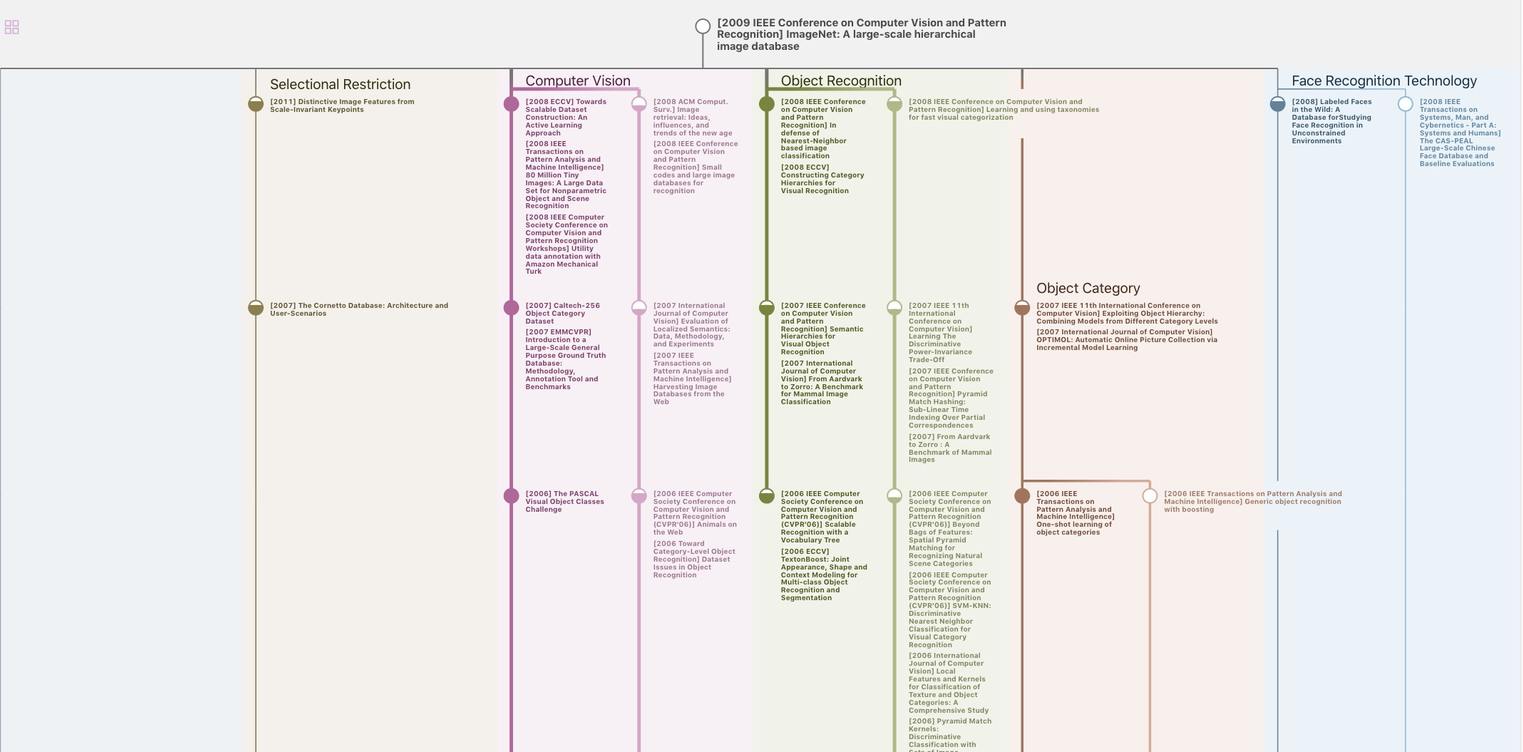
生成溯源树,研究论文发展脉络
Chat Paper
正在生成论文摘要