Robust Reward Placement under Uncertainty
CoRR(2024)
Abstract
Reward placement is a common optimization problem in network diffusion
processes, where a number of rewards are to be placed in a network so as to
maximize the total reward obtained as agents move randomly in it. In many
settings, the precise mobility network might be one of several possible, based
on parameters outside our control, such as the weather conditions affecting
peoples' transportation means. Solutions to the reward placement problem must
thus be robust to this uncertainty, by achieving a high utility in all possible
networks. To study such scenarios, we introduce the Robust Reward Placement
problem (RRP). Agents move randomly on a Markovian Mobility Model that has a
predetermined set of locations but its precise connectivity is unknown and
chosen adversarialy from a known set Π of candidates. Network optimization
is achieved by selecting a set of reward states, and the goal is to maximize
the minimum, among all candidates, ratio of rewards obtained over the optimal
solution for each candidate. We first prove that RRP is NP-hard and
inapproximable in general. We then develop Ψ-Saturate, a pseudo-polynomial
time algorithm that achieves an ϵ-additive approximation by exceeding
the budget constraint by a factor that scales as O(ln|Π|/ϵ). In
addition, we present several heuristics, most prominently one inspired from a
dynamic programming algorithm for the max-min 0-1 Knapsack problem. We
corroborate our theoretical findings with an experimental evaluation of the
methods in both synthetic and real-world datasets.
MoreTranslated text
AI Read Science
Must-Reading Tree
Example
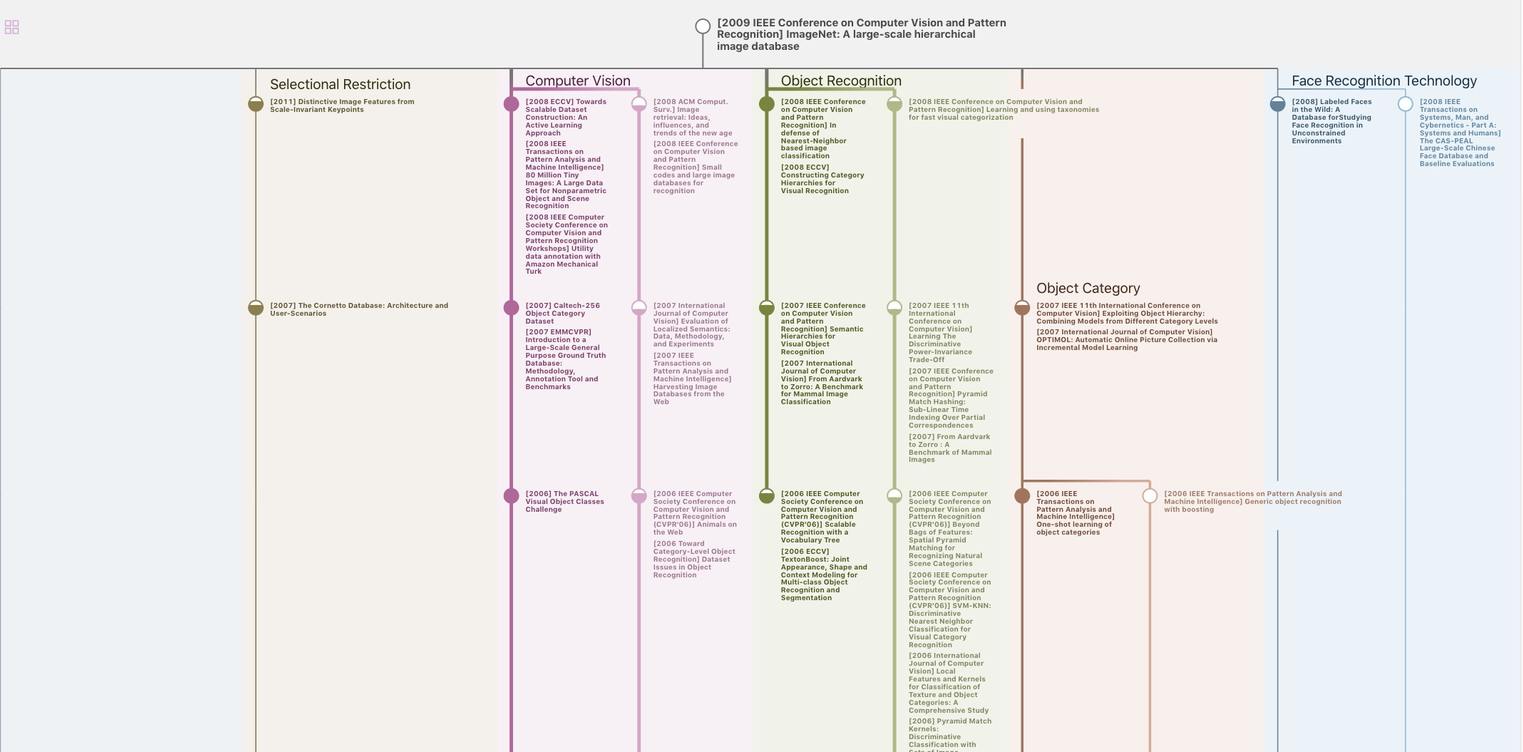
Generate MRT to find the research sequence of this paper
Chat Paper
Summary is being generated by the instructions you defined