Predictive modeling of land surface temperature (LST) based on Landsat-8 satellite data and machine learning models for sustainable development
JOURNAL OF CLEANER PRODUCTION(2024)
摘要
Accurate prediction of Land Surface Temperature (LST) is critical for understanding and mitigating the effects of climate change and land use dynamics. This study proposes a novel approach that leverages ensemble models and correlation analysis based on Landsat -8 satellite data to forecast LST and explore its environmental relationships. Time -series satellite data spanning winter and summer seasons of 2018-2019 was retrieved from the Google Earth Engine (GEE) platform. LST, normalized difference vegetation index (NDVI), rainfall, and evapotranspiration (ET) datasets were derived from Landsat -8 data within GEE to facilitate LST modeling. The ensemble framework combines three powerful machine learning algorithms: XG-Boost, Bagging-XG-Boost, and AdaBoost, to enhance the accuracy and robustness of LST predictions. Compared to standalone models, the proposed ensemble models demonstrated significant improvements in LST prediction accuracy. While XG-Boost and AdaBoost achieved moderate accuracies with R2 values of 0.57 and 0.60, respectively, the Bagging ensemble model surpassed them with an outstanding R2 of 0.75. Furthermore, a correlation analysis by using linear regression (LR) model explored the relationships between ET, rainfall, NDVI, and LST. The analysis revealed strong positive correlations between NDVI and ET (R2 = 0.95), while correlations between NDVI and LST (R2 = 0.31) and NDVI and rainfall (R2 = 0.47) were weaker. These findings contribute significantly to our understanding of LST trends and the impact of climate change on environmental variables. Ultimately, this knowledge can inform effective sustainable decision-making in the area.
更多查看译文
关键词
LST,Machine learning,Satellite data,GEE,Ensemble model,Energy,SDG
AI 理解论文
溯源树
样例
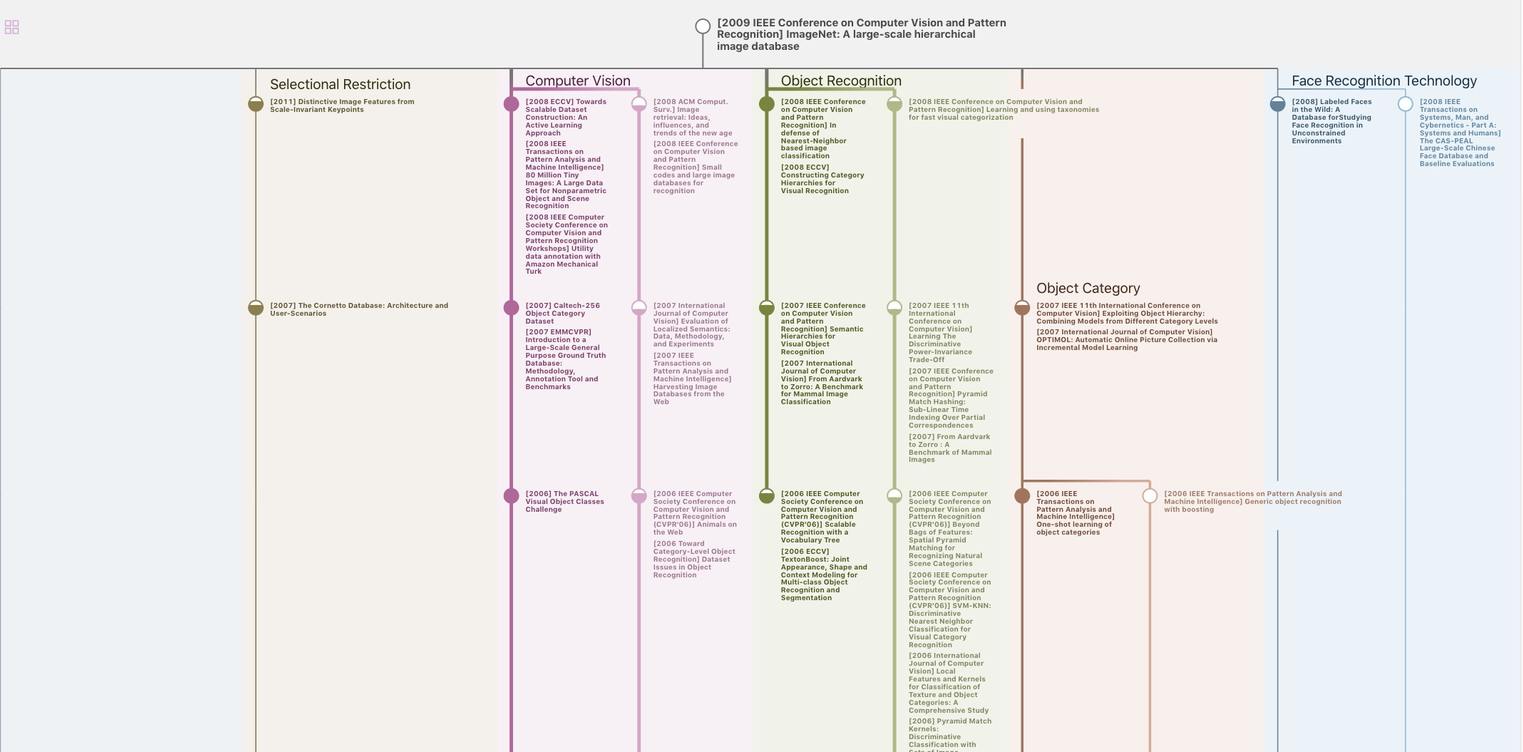
生成溯源树,研究论文发展脉络
Chat Paper
正在生成论文摘要