Cybernet Model: A New Deep Learning Model for Cyber DDoS Attacks Detection and Recognition
CMC-COMPUTERS MATERIALS & CONTINUA(2024)
摘要
Cyberspace is extremely dynamic, with new attacks arising daily. Protecting cybersecurity controls is vital for network security. Deep Learning (DL) models find widespread use across various fields, with cybersecurity being one of the most crucial due to their rapid cyberattack detection capabilities on networks and hosts. The capabilities of DL in feature learning and analyzing extensive data volumes lead to the recognition of network traffic patterns. This study presents novel lightweight DL models, known as Cybernet models, for the detection and recognition of various cyber Distributed Denial of Service (DDoS) attacks. These models were constructed to have a reasonable number of learnable parameters, i.e., less than 225,000, hence the name "lightweight." This not only helps reduce the number of computations required but also results in faster training and inference times. Additionally, these models were designed to extract features in parallel from 1D Convolutional Neural Networks (CNN) and Long ShortTerm Memory (LSTM), which makes them unique compared to earlier existing architectures and results in better performance measures. To validate their robustness and effectiveness, they were tested on the CIC-DDoS2019 dataset, which is an imbalanced and large dataset that contains different types of DDoS attacks. Experimental results revealed that both models yielded promising results, with 99.99% for the detection model and 99.76% for the recognition model in terms of accuracy, precision, recall, and F1 score. Furthermore, they outperformed the existing state-of-the-art models proposed for the same task. Thus, the proposed models can be used in cyber security research domains to successfully identify different types of attacks with a high detection and recognition rate.
更多查看译文
关键词
Deep learning,CNN,LSTM,Cybernet model,DDoS recognition
AI 理解论文
溯源树
样例
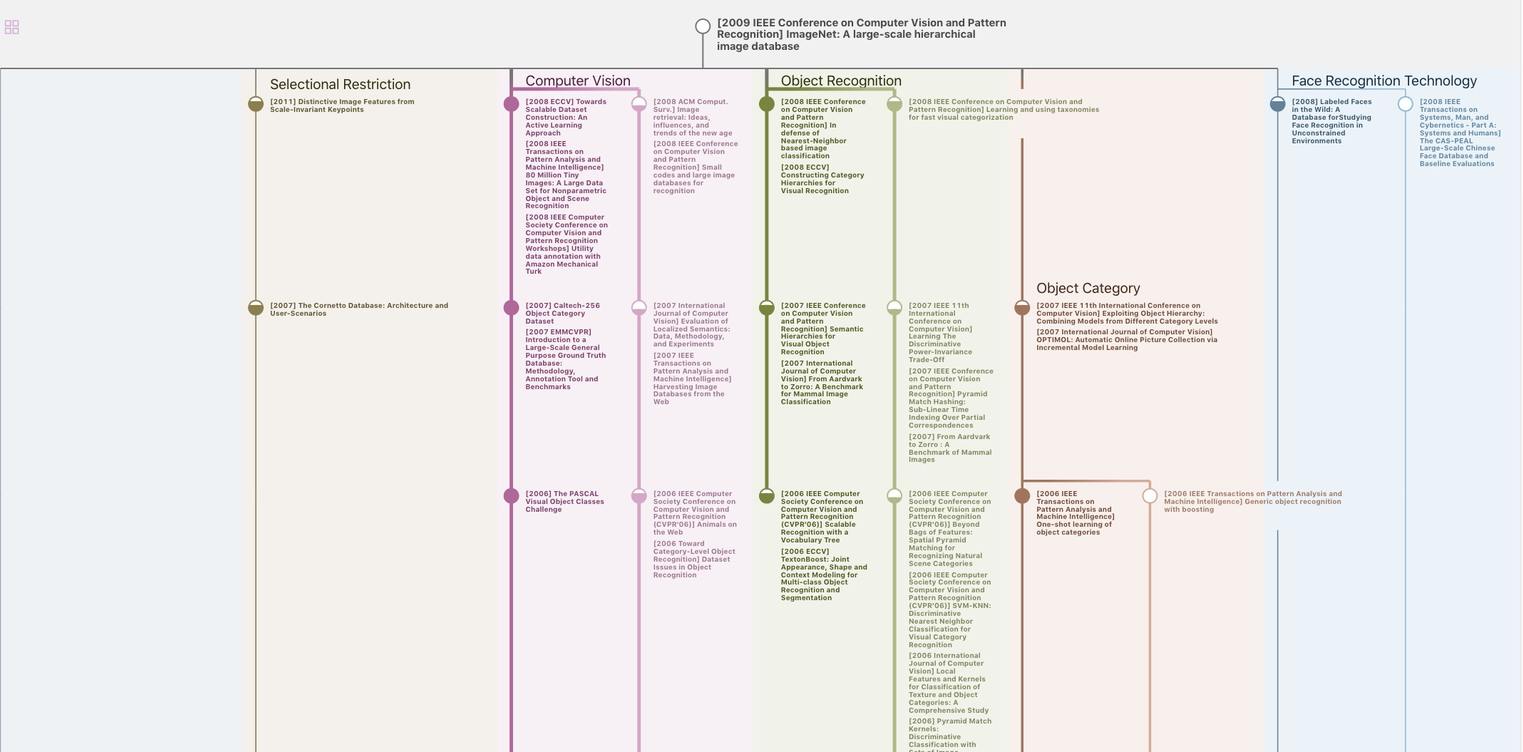
生成溯源树,研究论文发展脉络
Chat Paper
正在生成论文摘要