DGConv: A Novel Convolutional Neural Network Approach for Weld Seam Depth Image Detection
CMC-COMPUTERS MATERIALS & CONTINUA(2024)
摘要
We propose a novel image segmentation algorithm to tackle the challenge of limited recognition and segmentation performance in identifying welding seam images during robotic intelligent operations. Initially, to enhance the capability of deep neural networks in extracting geometric attributes from depth images, we developed a novel deep geometric convolution operator (DGConv). DGConv is utilized to construct a deep local geometric feature extraction module, facilitating a more comprehensive exploration of the intrinsic geometric information within depth images. Secondly, we integrate the newly proposed deep geometric feature module with the Fully Convolutional Network (FCN8) to establish a high-performance deep neural network algorithm tailored for depth image segmentation. Concurrently, we enhance the FCN8 detection head by separating the segmentation and classification processes. This enhancement significantly boosts the network's overall detection capability. Thirdly, for a comprehensive assessment of our proposed algorithm and its applicability in real-world industrial settings, we curated a line-scan image dataset featuring weld seams. This dataset, named the Standardized Linear Depth Profile (SLDP) dataset, was collected from actual industrial sites where autonomous robots are in operation. Ultimately, we conducted experiments utilizing the SLDP dataset, achieving an average accuracy of 92.7%. Our proposed approach exhibited a remarkable performance improvement over the prior method on the identical dataset. Moreover, we have successfully deployed the proposed algorithm in genuine industrial environments, fulfilling the prerequisites of unmanned robot operations.
更多查看译文
关键词
Weld image detection,deep learning,semantic segmentation,depth map geometric feature extraction
AI 理解论文
溯源树
样例
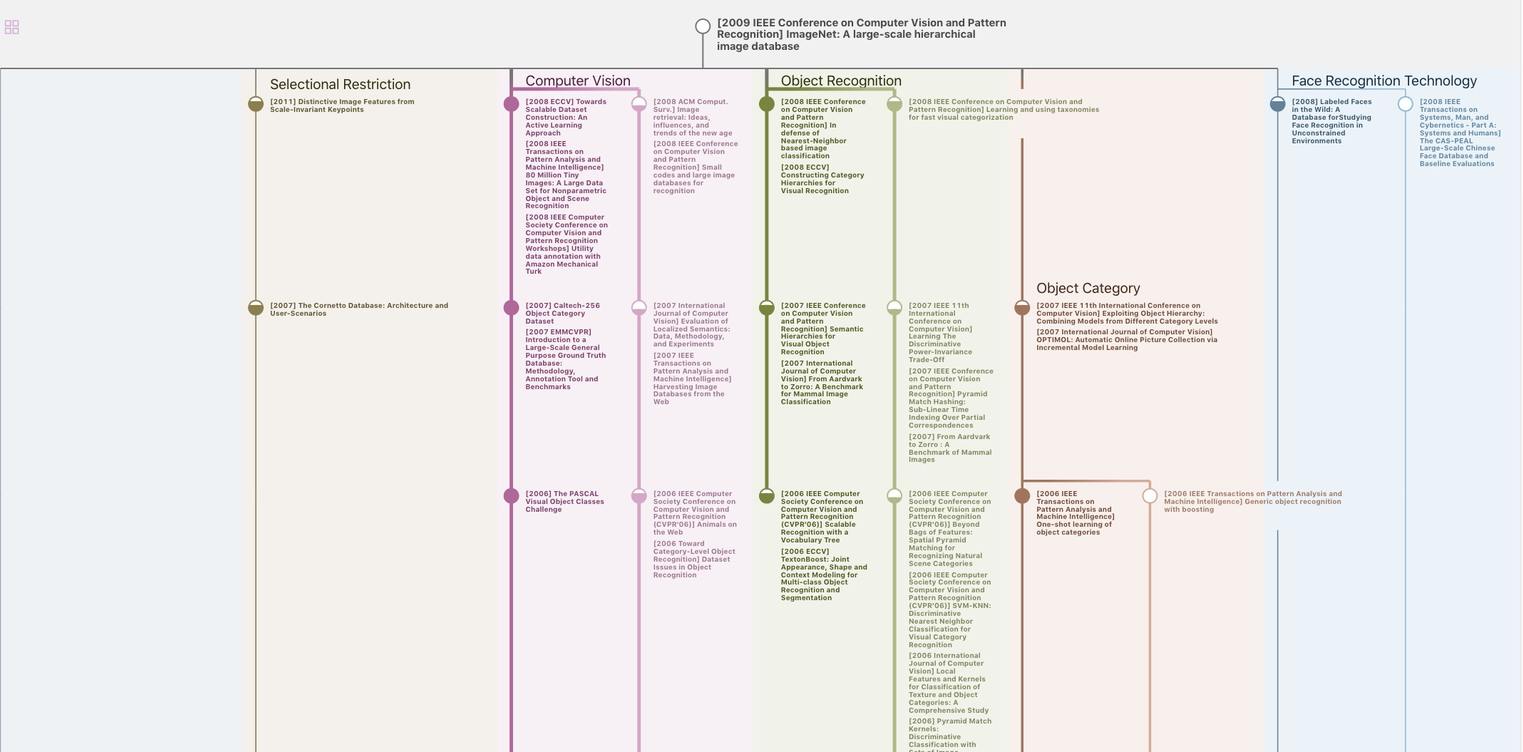
生成溯源树,研究论文发展脉络
Chat Paper
正在生成论文摘要