Artificial Intelligence for Safety and Reliability: A Descriptive, Bibliometric and Interpretative Review on Machine Learning
Journal of Loss Prevention in the Process Industries(2024)
摘要
This research provides a structured review of studies that utilize Artificial Intelligence for safety and reliability. In particular, it focuses on Machine Learning techniques to perform fault detection and diagnosis, anomaly detection, system prognosis, reliability analysis, and risk assessment of engineering-related systems across the industry. Relevant studies were identified through clear research questions, screened, and assessed for eligibility. Explicit inclusion and exclusion criteria were defined to verify the suitability of the records. The analysis encompasses a descriptive, bibliometric, and interpretative review. The descriptive analysis details how different ML approaches are adapted and implemented across various domains of safety and reliability. The bibliometric analysis provides in-depth and comprehensive statistics, covering aspects such as data types used, preprocessing steps undertaken, and categories of ML algorithms employed. The interpretative analysis offers a critical and forward-looking perspective on the current state of the field. A total of 308 papers were analyzed, mostly adopting supervised learning frameworks (81%) and addressing fault detection and diagnosis (57%) in more than 16 different industrial fields. The outcome of this study reflects the rapid development of this cross-cutting and interdisciplinary research field and highlights the potential for future improvement in the data-driven operational safety of industrial plants. Challenges and limitations have been highlighted and discussed, including data availability and label scarcity, data quality, trust and explainability, and interdisciplinary collaboration. Additionally, suggestions on potential solutions to overcome existing limitations and outline future directions are provided.
更多查看译文
AI 理解论文
溯源树
样例
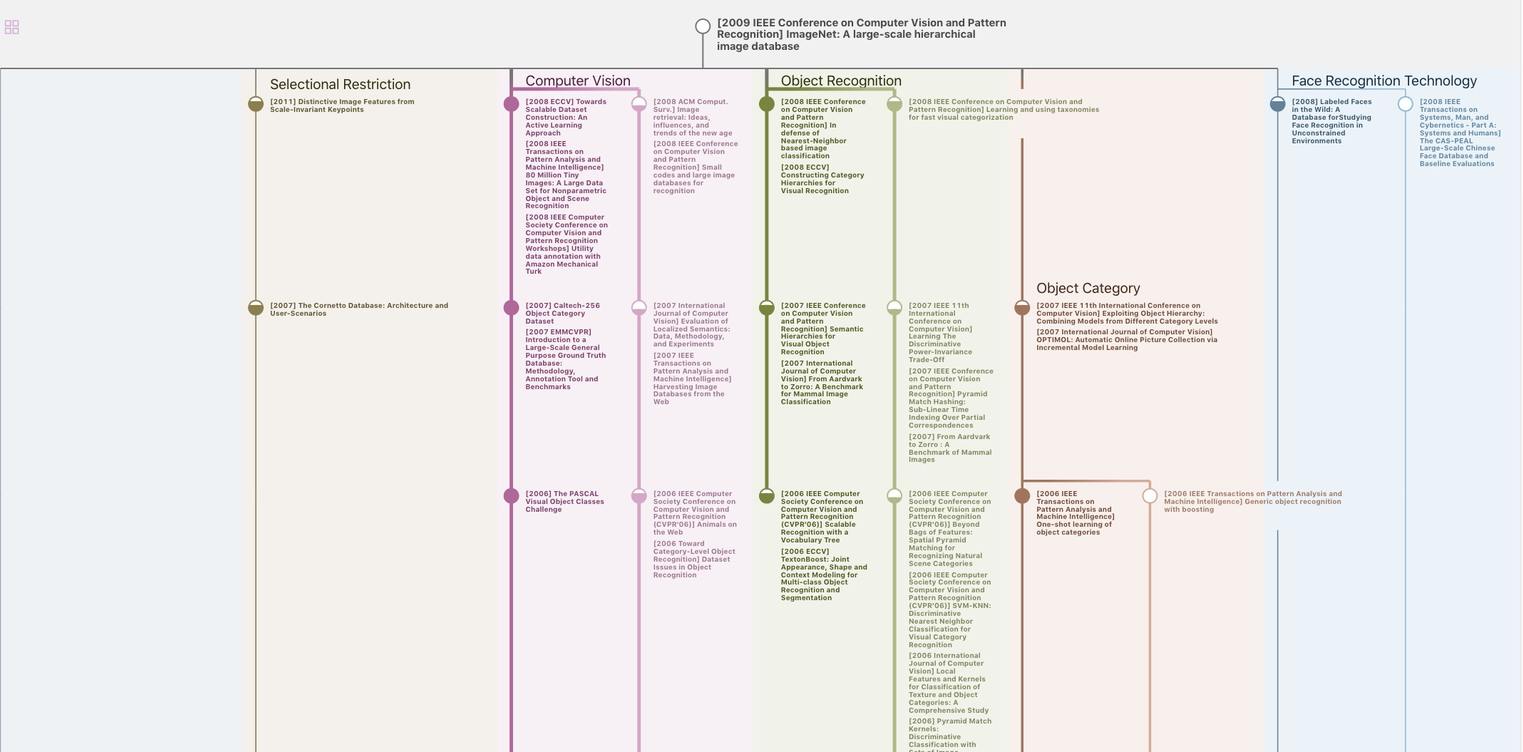
生成溯源树,研究论文发展脉络
Chat Paper
正在生成论文摘要