Advancing robust regression: Addressing asymmetric noise with the BLINEX loss function
Information Fusion(2024)
摘要
In real-world applications, regression performance is significantly impeded by complex noise. The choice of loss function is pivotal in building robust regression models, which broadly fall into two categories: bounded symmetric loss functions and asymmetric loss functions. The former struggle with asymmetric noise, while the latter are formulated as segmented functions involving complicates optimization. To handle various noise within a concise framework, we propose a robust regression model based on the asymmetric bounded linear-exponential (BLINEX) loss function, called BXSVR. Firstly, the asymmetry of the BLINEX loss function enables BXSVR to apply different penalties to positively and negatively skewed noise with equal regression error magnitudes to balance the effect of asymmetric noise. Secondly, the boundedness of the BLINEX loss function allows BXSVR to mitigate the detrimental effects of noise. Thirdly, the smoothness and differentiability of the BLINEX loss function contributes to the optimization of BXSVR. Due to the above properties, BSXVR can adaptively and effectively manage diverse noise scenarios. We use the Nesterov accelerated gradient (NAG) algorithm to optimize BSXVR and analyze the complexity of our algorithm. Extensive experiments on synthetic datasets, real-world datasets, and an application dataset confirm that BXSVR is more competitive than other benchmark methods in terms of various measures.
更多查看译文
关键词
Support vector regression,Asymmetric noise,BLINEX loss function,Robustness
AI 理解论文
溯源树
样例
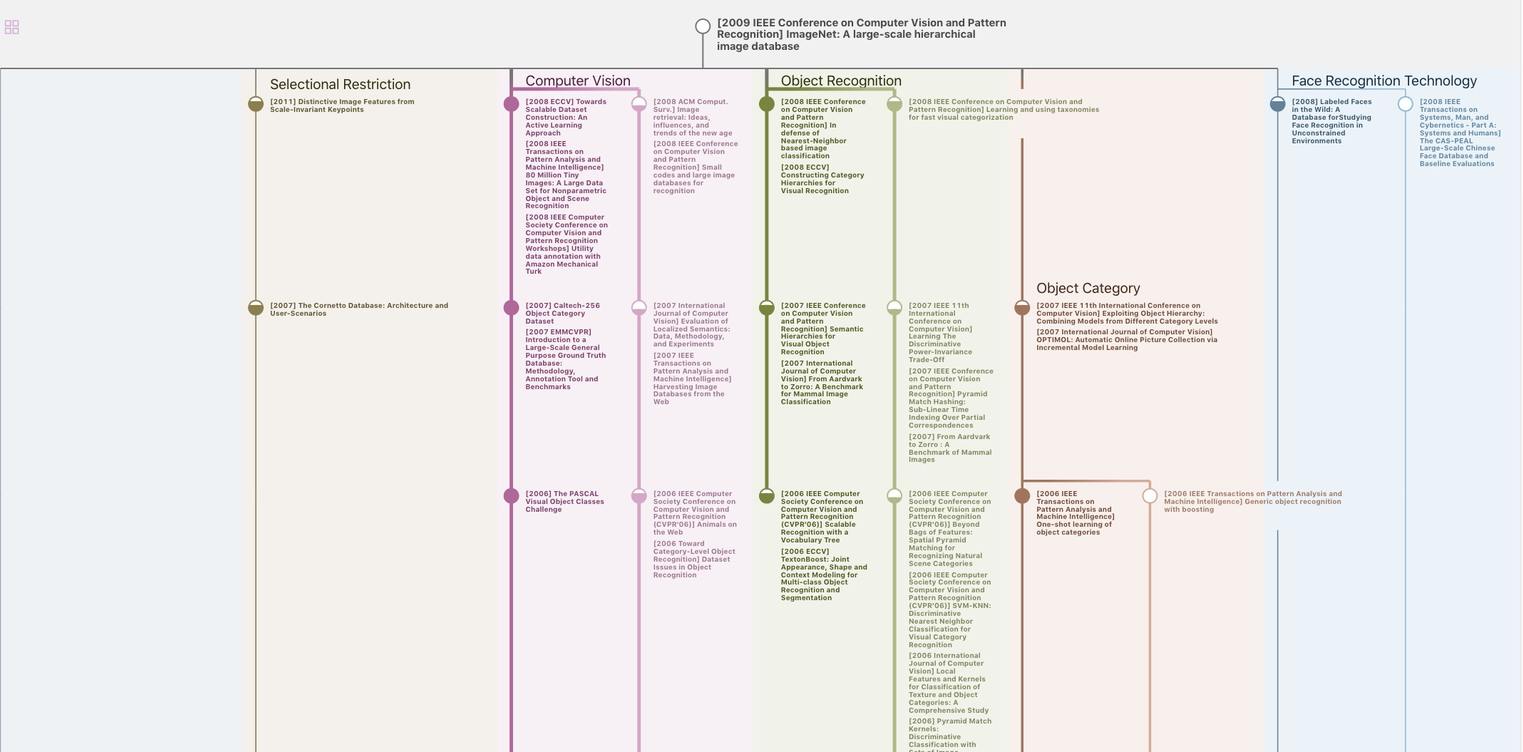
生成溯源树,研究论文发展脉络
Chat Paper
正在生成论文摘要